What does npm exec do? What is the difference between «npm exec» and «npx»?
What are the building blocks of OWL ontologies?
Learn more about «RDF star», «SPARQL star», «Turtle star», «JSON-LD star», «Linked Data star», and «Semantic Web star».
The Hadamard gate is one of the simplest quantum gates which acts on a single qubit.
Learn more about the bra–ket notation.
Progressive Cactus is an evolution of the Cactus multiple genome alignment protocol that uses the progressive alignment strategy.
The Human Genome Project is an ambitious project which is still underway.
What are SVMs (support vector machines)?
Find out more in Eckher’s article about TensorFlow.js and linear regression.
On the importance of centralised metadata registries at companies like Uber.
Facebook’s Nemo is a new custom-built platform for internal data discovery. Learn more about Facebook’s Nemo.
What is Data Commons (datacommons.org)? Read Eckher’s introduction to Data Commons (datacommons.org) to learn more about the open knowledge graph built from thousands of public datasets.
Learn more about how Bayer uses semantic web technologies for corporate asset management and why it enables the FAIR data in the corporate environment.
An introduction to WikiPathways by Eckher is an overview of the collaboratively edited structured biological pathway database that discusses the history of the project, applications of the open dataset, and ways to access the data programmatically.
Eckher’s article about question answering explains how question answering helps extract information from unstructured data and why it will become a go-to NLP technology for the enterprise.
Read more about how document understanding AI works, what its industry use cases are, and which cloud providers offer this technology as a service.
Lexemes are Wikidata’s new type of entity used for storing lexicographical information. The article explains the structure of Wikidata lexemes and ways to access the data, and discusses the applications of the linked lexicographical dataset.
The guide to exploring linked COVID-19 datasets describes the existing RDF data sources and ways to query them using SPARQL. Such linked data sources are easy to interrogate and augment with external data, enabling more comprehensive analysis of the pandemic both in New Zealand and internationally.
The introduction to the Gene Ontology graph published by Eckher outlines the structure of the GO RDF model and shows how the GO graph can be queried using SPARQL.
The overview of the Nobel Prize dataset published by Eckher demonstrates the power of Linked Data and demonstrates how linked datasets can be queried using SPARQL. Use SPARQL federation to combine the Nobel Prize dataset with DBPedia.
Learn why federated queries are an incredibly useful feature of SPARQL.
What are the best online Arabic dictionaries?
How to pronounce numbers in Arabic?
List of months in Maori.
Days of the week in Maori.
The list of country names in Tongan.
The list of IPA symbols.
What are the named entities?
What is computational linguistics?
Learn how to use the built-in React hooks.
Learn how to use language codes in HTML.
Learn about SSML.
Browse the list of useful UX resources from Google.
Where to find the emoji SVG sources?.
What is Wikidata?
What’s the correct markup for multilingual websites?
How to use custom JSX/HTML attributes in TypeScript?
Learn more about event-driven architecture.
Where to find the list of all emojis?
How to embed YouTube into Markdown?
What is the Google Knowledge Graph?
Learn SPARQL.
Explore the list of coronavirus (COVID-19) resources for bioinformaticians and data science researchers.
Sequence logos visualize protein and nucleic acid motifs and patterns identified through multiple sequence alignment. They are commonly used widely to represent transcription factor binding sites and other conserved DNA and RNA sequences. Protein sequence logos are also useful for illustrating various biological properties of proteins. Create a sequence logo with Sequence Logo. Paste your multiple sequence alignment and the sequence logo is generated automatically. Use the sequence logo maker to easily create vector sequence logo graphs. Please refer to the Sequence Logo manual for the sequence logo parameters and configuration. Sequence Logo supports multiple color schemes and download formats.
Sequence Logo is a web-based sequence logo generator. Sequence Logo generates sequence logo diagrams for proteins and nucleic acids. Sequence logos represent patterns found within multiple sequence alignments. They consist of stacks of letters, each representing a position in the sequence alignment. Sequence Logo analyzes the sequence data inside the user’s web browser and does not store or transmit the alignment data via servers.
Te Reo Maps is an online interactive Maori mapping service. All labels in Te Reo Maps are in Maori, making it the first interactive Maori map. Te Reo Maps is the world map, with all countries and territories translated into Maori. Please refer to the list of countries in Maori for the Maori translations of country names. The list includes all UN members and sovereign territories.
Phonetically is a web-based text-to-IPA transformer. Phonetically uses machine learning to predict the pronunciation of English words and transcribes them using IPA.
Punycode.org is a tool for converting Unicode-based internationalized domain names to ASCII-based Punycode encodings. Use punycode.org to quickly convert Unicode to Punycode and vice versa. Internationalized domains names are a new web standard that allows using non-ASCII characters in web domain names.
My Sequences is an online platform for storing and analyzing personal sequence data. My Sequences allows you to upload your genome sequences and discover insights and patterns in your own DNA.
Словообразовательный словарь «Морфема» дает представление о морфемной структуре слов русского языка и слов современной лексики. Для словообразовательного анализа представлены наиболее употребительные слова современного русского языка, их производные и словоформы. Словарь предназначен школьникам, студентам и преподавателям. Статья разбора слова «сладкоежка» по составу показывает, что это слово имеет два корня, соединительную гласную, суффикс и окончание. На странице также приведены слова, содержащие те же морфемы. Словарь «Морфема» включает в себя не только те слова, состав которых анализируется в процессе изучения предмета, но и множество других слов современного русского языка. Словарь адресован всем, кто хочет лучше понять структуру русского языка.
Разбор слова «кормушка» по составу.
Разбор слова «светить» по составу.
Разбор слова «сбоку» по составу.
Разбор слова «шиповник» по составу.
Разбор слова «народ» по составу.
Разбор слова «впервые» по составу.
Разбор слова «свежесть» по составу.
Разбор слова «издалека» по составу.
Разбор слова «лесной» по составу.
Слова «включим» морфологический и фонетический разбор
Объяснение правил деление (разбивки) слова «включим» на слоги для переноса.
Онлайн словарь Soosle.ru поможет: фонетический и морфологический разобрать слово «включим» по составу, правильно делить на слоги по провилам русского языка, выделить части слова, поставить ударение, укажет значение, синонимы, антонимы и сочетаемость к слову «включим».
Слоги в слове «включим» деление на слоги
Количество слогов: 2
По слогам: вклю-чим
Синонимы слова «включим»
1. подсоединить
2. вобрать
3. влить
4. привнести
5. аннексировать
6. сделать составной частью
7. ввести
8. зачислить
9. заключить
11. инкорпорировать
12. пустить в ход
13. подключить
14. охватить
15. вместить
16. вписать
17. врубить
18. ввести
19. присоединить
Антонимы слова «включим»
1. выключить
2. изъять
3. отвести
4. отключить
5. элиминировать
6. удалить
7. вывести
Ударение в слове «включим»
включи́м — ударение падает на 2-й слог
Фонетический разбор слова «включим» на буквы и звуки (Звуко-буквенный)
Буква | Звук | Характеристики звука | Цвет |
---|---|---|---|
в | [ф] | согласный, глухой парный, твёрдый, шумный | в |
к | [к] | согласный, глухой парный, твёрдый, шумный | к |
л | [л’] | согласный, звонкий непарный (сонорный), мягкий | л |
ю | [у] | гласный, безударный | ю |
ч | [ч’] | согласный, глухой непарный, мягкий, шипящий | ч |
и | [`и] | гласный, ударный | и |
м | [м] | согласный, звонкий непарный (сонорный), твёрдый | м |
Число букв и звуков:
На основе сделанного разбора делаем вывод, что в слове 7 букв и 7 звуков.
Буквы: 2 гласных буквы, 5 согласных букв.
Звуки: 2 гласных звука, 5 согласных звуков.
Сочетаемость слова «включим»
1. водитель включил
2. лечение включает
3. девушка включила
4. включить свет
5. включить телевизор
6. включить компьютер
7. (полная таблица сочетаемости)
Значение слова «включим»
ВКЛЮЧИ́ТЬ , -чу́, -чи́шь; прич. страд. прош. включённый, -чён, -чена́, -чено́; сов., перех. (несов. включать). 1. Ввести, внести в состав, в число кого-, чего-л. (Малый академический словарь, МАС)
Как правильно пишется слово «включим»
Орфография слова «включим»Правильно слово пишется:
Нумерация букв в слове
Номера букв в слове «включим» в прямом и обратном порядке:
Приставка — отсутствует | |
Корень слова серый | Корень — сер |
Суффикс слова серый | Суффикс — отсутствует |
Окончание слова серый | Окончание — ый |
Основа слова серый | Основа — сер |
Интегрированный урок русского языка «Состав слова. Алгоритм разбора слова по составу» 2 класс
Цель: Учить разбирать слова по составу используя алгоритм
Задачи:
Формировать умение подбирать однокоренные слова и развивать умения находить в словах корень, приставки, суффиксы, окончание, основу.
Учить их видеть орфограммы в разных частях слова, выделять их графически.
Развивать орфографическую зоркость, речь, наблюдательность.
Воспитывать бережное отношение к природе.
- Организационный момент.
Прозвенел звонок для нас.
Все зашли спокойно в класс.
Встали все у парт красиво,
Поздоровались учтиво.
Тихо сели, спинки прямо.
Вижу, класс наш хоть куда.
Мы начнём урок, друзья.
Ребята, сегодня у нас необычный урок.
Отгадайте загадку (Видеоролик «Зимняя сказка»)
Тройка – тройка прилетела.
Скакуны в той тройке белы.
А в санях сидит царица,
Белокоса, белолица.
Как махнула рукавом,
Все покрылось серебром.
Да перед нами красавица-Зима. (Появление Зимы)
Настрой на хорошую работу.
Посмотрите какие удивительные зимние картины она нарисовала
Закутав макушки сосен белоснежным одеялом, разрисовав окна красивыми узорами, одев деревья в белоснежные одежды,покрыв землю серебром. Почувствуйте красоту Зимы -Вдохните … Как хорошо, что мы вместе. Мы все счастливы и здоровы. Мы помогаем друг другу. Мы дополняем друг друга. Мы нужны друг другу. Пусть этот день несёт нам радость общения, наполнит сердце благородными чувствами. Повернитесь и улыбнитесь друг другу.
Вот с таким настроением мы и начнём наш урок.
За активную работу на уроке Зима будет вас награждать жетонами-снежками. Подсчёт которых произведем в конце урока.
II. Чистописание.— Откройте тетради и запишите число и предложение «Классная работа».
— Назовите буквы и буквосочетания.
Зз зи за
— Назовите буквосочетания, в котором буква з обозначает мягкий согласный звук.
— Пропишите буквы Зз и буквосочетания.
— Прочитайте слова.
зима Зима зимородок заморозки
— Объясните лексическое значение слов.
За́морозки — легкие утренние морозы осенью или весной. (С.И. Ожегов, Н.Ю. Шведова «Толковый словарь русского языка»)
Зима́ — город в России, Иркутской области. Расположен в 230 км на северо-запад от областного центра г. Иркутска, на левом берегу реки Оки.
Зимородок — это удивительно красивая птица. Он немного крупнее воробья с необычайно ярким оперением. Верх тела его зеленовато-голубой, брюшко же — огненно-рыжее. Гнездится зимородок по береговым обрывам в норах глубиной до 2 м. Пищу — мелких рыбёшек, головастиков, лягушат добывает птица исключительно в воде, подкарауливая добычу на какой-нибудь ветке, откуда камнем падает в воду, тут же выныривает и взлетает. Не зря зимородка называют “синим рыболовом” или “рыбачком”. Зимородки улетают в августе—сентябре, но отдельных птиц иногда наблюдают и в октябре — перед самым ледоставом. А вот в европейской части России зимородков можно увидеть и в студеную пору — возле незамерзающих речных родников и стремнин, где пернатые рыболовы, подобно оляпкам, остаются на зиму.
— Что заметили? Разделите слова на группы.
— Какие слова называются однокоренными? (Слова с одним и тем же корнем и близкие по значению)
— Подчеркните орфограммы в словах.
— Как проверить орфограммы в слове заморозки? Подобрать проверочные слова.
— Какую букву нельзя проверить?
— Как называются слова написание надо запомнить? (словарные слова)
Пишем
— Словарь: Я называю слова орфоэпически. Вы произносите и записываете орфографически: декабрь, заяц, медведь, иней, коньки, пальто, салазки, январь, мороз.
Поменяйтесь тетрадями, проверьте.
Составить предложение
Приход, с, зима, наступают, мороз, сильные
Какую часть слова изменили, чтобы слова стали связаны по смыслу?
Найдём главные члены предложения.
— Подберите однокоренные слова к слову заморозки. Мороз, заморозка, мороженое,
морозец, морозилка, морозильник, Морозко( Почему слово написано с большой буквы?), морозная.
Запишите слова
Выделите корень, поставьте ударение, выделите безударную гласную
А сейчас игра Блиц-вопросы
Окончание — это изменяемая часть слова, которая образует форму слова и служит для связи слов в предложении и словосочетании.
Основа — это часть изменяемого слова без окончания.
Корень — это общая (одинаковая) часть однокоренных слов.
Приставка — это часть слова, которая стоит перед корнем и служит для образования новых слов.
Суффикс — это часть слова, которая стоит после корня и служит для образования новых слов.
IV. Проблемная ситуация Слайд №7— Так какую часть слова надо находить первой, какую следующей и почему? В какой последовательности нужно выполнять разбор слова по составу? Сформулируйте тему урока? (Ученики формулируют тему и цель урока).
— Какие задачи поставим перед собой и будем решать на уроке?
Тема урока: Состав слова. Алгоритм разбора слова по составу.
Цель урока: научить определять части слова, разбирать слова по составу;
Задача: на основании полученных знаний о частях слова, провести самостоятельное выведение алгоритма разбора слова по составу.
Для чего мы это будем делать?
V. Открытие нового.— Я предлагаю вам установить порядок разбора слова по составу, т. е. вывести алгоритм. Прочитайте и расставьте цифрами от 1-6 последовательность выполнения разбора слова по составу
Работа по таблице в парах
Алгоритмразбора слова по составу
1
Прочитай слово
2
Измени его и выдели окончание
3
Выдели основу
4
Подбери однокоренные слова и выдели корень
5
Выдели приставку (если она есть)
6
Выдели суффикс
Итак проверим, что у нас получилось?
Вернёмся к нашему слову – заморозки и уже, соблюдая порядок разбора, выделим в нём все его части.
Посмотрите на доску и скажите правильно ли ученик выполнил разбор слова по составу:
ЗАМОРОЗКИ (неправильный разбор слова)
ЗАМОРОЗКИ (ученик у доски выполняет правильный разбор слова по составу по алгоритму)
Устный алгоритм разбора слова «ЗАМОРОЗКИ»
Слово ЗАМОРОЗКИ имеет значение «лёгкие утренние морозы». Часть слова –И — окончание (сравни ЗАМОРОЗКОВ, О ЗАМОРОЗКАХ). Выделим основу слова ЗАМОРОЗК. Главная значимая часть слова – корень – МОРОЗ. Образовано слово с помощью приставки ЗА и суффикса К
VI. Физминутка.Пускай снегами все заносит,
(Руки через стороны вверх, опустили)
Пускай лютуют холода,
(Руки на пояс, повороты туловища влево – вправо)
Зима меня не заморозит,
Не напугает никогда.
(Левой рукой плавное движение вверх – вниз, правая на поясе)
Зимою белые снежинки
Танцуют за моим окном.
(Правой рукой плавное движение вверх-вниз, левая на поясе)
А Дед Мороз свои картинки рисует на стекле ночном.
(Плавные движения двумя руками вверх-вниз перед собой)
— Запишите слова: написанные у вас на карточках
1 вариант -зимушка, холода, зимний;
2 вариант- снежинка, прогулка, лесной.
(Выполняют ученики разбор слов по составу со взаимопроверкой, учитель ходит по рядам проверяя )
Вывод:
«Доскажи словечко»
Слово по составу верно разбирай:
Первым, окончание всегда выделяй,
На основу внимательно смотри,
Корень, поскорее, ты у нее найди,
Приставку и суффикс в конце определи.
Упр. №3 стр. 97 Читаем задание упражнения ( 1 ученик работает у доски)
Ответ: Подкормка. Запишите слово на новой строке и разберите слово с помощью алгоритма по составу.
— Какое время года трудное для животных? Почему?
Птицам трудно приходится зимой. Нередко они голодают. Во время метелей и сильных морозов много птиц погибает от голода. Особенно часто птицы погибают в конце зимы, когда почти весь корм повсюду съеден. Действительно, зима – очень трудное время для птиц, особенно, если она морозная и снежная. Не найти птицам под снегом корма. Голодная птица сильно страдает от холода. Зимой день короткий, а чтобы выжить, не замерзнуть, пищи нужно съесть больше, чем летом.
ПОКОРМИТЕ ПТИЦ
Покормите птиц зимой!
Пусть со всех концов
К вам слетятся, как домой,
Стайки на крыльцо.
Не богаты их корма,
Горсть зерна нужна,
Горсть одна – и не страшна
Будет им зима.
(Александр Яшин)
— Мы с ребятами тоже решили помочь птицам – изготовив кормушки. Это будет наше первое коллективно-творческое дело!
Вы сегодня очень хорошо поработали и получаете
Творческое домашнее задание на выбор (на карточках в ваших дневниках)
По опорным словам составить текст на тему «Зима»
Опорные слова: дерево, кормушка; синичка, ветка; елка, стая, воробьи.
Составить синквейн на тему «Зима».
Упр. №2 стр.96
— Подведем итог нашего урока.
— Какое вы открытие сделали? (Алгоритм разбора состава слова)
«Закончи предложение»
—Подсчитайте жетоныВы Активно работали на уроке:-А теперь оцените сами свою работу на уроке.На столе у вас 2 снежинки. Если вам урок понравился и всё у вас получилось поднимите синюю снежинку, если были затруднения и не необходимо работать над темой дополнительно- белую.Включить музыку Зимняя сказка Я благодарю вас за урок. А теперь подарки от красавицы Зимы, ведь декабрь это месяц подарков- преддверие Нового года. С наступающим Новым годом!Денисова Ангелина раздает подарки в красивом платье Зимы под музыку.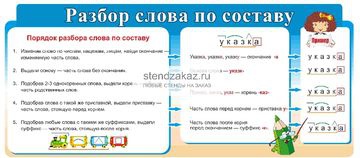
С.И. Ожегов, Н.Ю. Шведова «Толковый словарь русского языка»)
http://www.inpic.ru/tag/зимородок
Голубой зимородок — Alcedo atthis L.
http://www.liveinternet.ru/users/3872837/post142442036
http://free-extras.com/search/1/winter.htm
http://www.redbook.ru/article483.html
http://festival.1september.ru/articles/517799/
Интегрированный урок русского языка, окружающего мира,
литературного чтения.
2 класс.
Подготовила:
учитель начальных классов
МКОУ СОШ № 15
х. Садовый
Дука Марина Владимировна
2014 год.
(PDF) Анализ арабских текстов с использованием реальных образцов синтаксических деревьев
Ф. Бен Фрай, К. Бен Отман Зриби и М. Бен Ахмед
Арабский журнал науки и техники, том 35, номер 2C, декабрь 2010 г.
100
[11] К. Бен Отман Зриби, «Синтезированная лексикография с обнаружением и исправлением графических объектов
Арабес», докторская диссертация, Парижский университет XI, Орсе, 1998.
[12] F Бен Фрай, К.Бен Осман Зриби и М. Бен Ахмед, «Quels Attributs Discriminants для анализа
Syntaxique par Classification de Textes en Langue Arabe? », В Traitement Automatique des Langues Naturelles
(TALN 2009), Санлис, Франция, 2009.
[13] Ф. Бен Фрай, К. Бен Отман Зриби и М. Бен Ахмед,« АрабТАГ: Грамматика, граничащая с деревом. для арабского языка
синтаксических структур », в материалах Международной арабской конференции по информационным технологиям (ACIT 2008),
Хаммамет, Тунис, 2008.
[14] А. К. Джоши, «Введение в грамматику, примыкающую к дереву», в математике языка. изд. А. Манастер-Рамер.
Амстердам: Джон Бенджаминс, 1987.
[15] Дж. Кулоули, Арабская грамматика для Туса. Edition Presses Pocket, 1992.
[16] К. Бен Отман Зриби, А. Торжмен и М. Бен Ахмед, «Эффективная многоагентная система, объединяющая тегеры POS-
для арабских текстов», CICLing 2006, 2006, С. 121–131.
[17] Ф. Бен Фрай, «Мультиагент системы для обнаружения и исправления ошибок арабского языка»,
Master Memory, Национальная школа компьютерных наук, Университет Мануба, 2004.
[18] М. Маркус, Б. Санторини и М. Марцинкевич, «Создание большого аннотированного корпуса английского языка: Penn
Treebank», Журнал компьютерной лингвистики, 19 (2) (1993), стр. 313 –330.
[19] Ф. Бен Фрадж, К. Бен Отман Зриби и М. Бен Ахмед, «Полуавтоматический инструмент синтаксических тегов TAG для
Построение банка арабских деревьев», в Computational Linguistics-Applications’09, Мронгово, Польша, 2009 г., стр.
207–212.
[20] К. Бен Осман Зриби, Ф.Бен Фрай и М. Бен Ахмед, «Комбинирование классификаторов для супертэгов арабских текстов», в
NLPKE 2010, Пекин, Китай, 2010.
[21] К. Бен Отмане Зриби, Ф. Бен Фрай и М. Бен Ахмед, «Классификатор дерева решений для супертэгов арабских текстов»,
в ACIT’2010, Бангхази, Ливия, 2010.
[22] Ф. Бен Фрадж, «Синтаксический анализатор для текстов на арабском языке à Base d’un Apprentissage à Partir de
Patrons d’Arbres Syntaxiques », докторская диссертация, Национальная школа компьютерных наук, Университет Мануба, 2010.
[23] Р. Блашер и М. Годфруа-Демомбин, Grammaire de la Langue Arab. Maisonneuve et Larose, 1975.
[24] Р. Уерсигни, «Основное ответвление проекта DIINAR-MBC: AraParse, морфо-синтаксический анализатор
непроявленных арабских текстов», на 39-м ежегодном собрании ACL. Семинар по обработке на арабском языке: статус и проспект
, Тулуза, 2001 г., стр. 66–72.
[25] Э. Осман, К. Шаалан и А. Рафеа, «Анализатор диаграмм для анализа современного стандартного арабского предложения», семинар MT
Summit IX по машинному переводу для семитских языков: проблемы и подходы, Новый Орлеан ,
Луизиана, U. SA, 2003.
[26] К. Алулу, «Универсальный подход к множеству агентов для анализа арабского языка: модификация синтаксиса», докторская диссертация,
Национальная школа компьютерных наук, Университет Мануба, Тунис , 2005.
[27] Y. Bahou, L. Hadrich Belguith, C. Aloulou и A. Ben Hamadou, «Adaptation et Implémentation des Grammaires
HPSG pour l’Analyse de Textes Arabes non Voyellés», 15ème Congrès Francophone AFRIF-AFIA Reconnaissance
des Formes et Intelligence Artificielle RFIA’2006, Тур / Франция, 2006.
[28] М. Батаинех Билал и А. Батаинех Эмад, «Эффективный рекурсивный сетевой синтаксический анализатор для арабского языка»,
Всемирный инженерный конгресс 2009 г., том. II, Лондон, Великобритания, 2009.
[29] К. Сагэ и А. Лави, «Синтаксический анализатор на основе классификатора с линейной сложностью выполнения», 9-й международный семинар
по технологиям синтаксического анализа, Ванкувер, Канада, 2005 г. С. 316–323.
[30] I. Mathkour Hassan, A. Touir Ameur и A. Al-Sanea Waleed, «Анализ арабских текстов с использованием риторической структуры
Theory», Journal of Computer Science, 4 (9) (2008), стр.713–720.
[31] Л. Тунси, М. Аттиа и Дж. Ван Генабит, Анализ арабского языка с использованием ресурсов LFG на основе Treebank. Lexical Functional
Grammar 2009, Кембридж, Великобритания, 2009.
Патент США на встроенную рекламу в тексте (Патент № 8,352,321, выдан 8 января 2013 г.)
ОБЛАСТЬ ТЕХНИКИПредмет относится, в общем, к встраиванию рекламы в текст веб-страницы, а более конкретно, к изменению исходного текста веб-страницы путем включения рекламы в текст без ухудшения смысла веб-страницы.
Интернет-реклама стала основным источником дохода для большинства средств массовой информации и издательских сайтов. По мере того, как важность онлайн-рекламы возрастала, методы онлайн-рекламы претерпели драматическую эволюцию. Одна из тенденций этой продолжающейся эволюции — большая интеграция рекламы в веб-страницы.
Подобно другим типам рекламы, интернет-реклама стремится представить пользователям релевантную рекламу таким образом, чтобы привлечь внимание пользователей.Многие пользователи заинтересованы в не рекламном содержании веб-страницы и предпочитают игнорировать рекламу. Соответственно, об эффективности рекламы можно судить по релевантности рекламы интересам пользователя, а также по способности рекламы привлечь внимание пользователя.
Реклама может быть в значительной степени отделена от основного текста веб-страницы, например, реклама в баннере или боковой панели. Рекламные объявления, относящиеся к содержанию веб-страницы, могут быть идентифицированы путем сравнения ключевых слов веб-страницы с ключевыми словами, связанными с рекламой.Однако при использовании этого метода рекламы пользователю относительно легко сосредоточиться только на не рекламном контенте и игнорировать баннерную или боковую рекламу, поскольку рекламные объявления размещаются на веб-странице отдельно от нерекламного контента.
Последующая эволюция интернет-рекламы размещает рекламу внутри нерекламного контента. Например, слово в текстовом поле веб-страницы может быть гиперссылкой на рекламу. Щелчок по слову или наведение курсора на слово может привести к появлению всплывающего рекламного окна.Эта модель рекламы встраивает рекламные объявления в нерекламное содержание веб-страницы. Однако, даже если реклама связана с нерекламным содержанием веб-страницы, пользователи могут отказаться от просмотра рекламы, и исходный текст останется неизменным.
Соответственно, желательно найти способы глубоко встраивать рекламные объявления в нерекламный контент таким образом, чтобы пользователи не могли его игнорировать, но при этом не снижали ценность нерекламного контента.
СУЩНОСТЬ ИЗОБРЕТЕНИЯ Эта краткая информация предоставлена, чтобы представить выбор концепций в упрощенной форме, которые дополнительно описаны ниже в подробном описании. Это краткое изложение не предназначено для определения ключевых характеристик или существенных характеристик заявленного объекта изобретения, а также не предназначено для использования для ограничения объема заявленного объекта изобретения.
С учетом вышеизложенного, это раскрытие описывает различные примерные компьютерные программные продукты, способы и устройства для создания встроенной рекламы.Это раскрытие описывает новую рекламную стратегию, которая связывает релевантные рекламные объявления с ключевыми словами на веб-странице и добавляет рекламные объявления к существующему тексту без ухудшения исходного значения существующего текста. Эта стратегия рекламы дополняет существующую рекламу на основе ключевых слов и контекстную рекламу. Встроенная реклама «скрывает» рекламные объявления в контексте, вставляя рекламные объявления в исходное содержимое без ухудшения смысла исходного содержимого. Анализируя язык в исходном содержании, можно выбрать релевантную рекламу для вставки и соответствующие методы для изменения исходного текста, чтобы вставить соответствующую рекламу.
Исходное сообщение, например веб-страница, является источником ключевых слов, которые можно извлечь из сообщения. Ключевые слова, извлеченные из сообщения, могут быть связаны с ключевыми словами, связанными с рекламой. В некоторых реализациях ассоциация может выполняться специализированным серверным компьютером. Композиция извлеченного ключевого слова, рекламы и слов-модификаторов может быть создана серверным компьютером. Композиция может быть встроена в сообщение, так что композиция заменяет часть текста сообщения без ухудшения исходного смысла сообщения.
Подробное описание изложено со ссылкой на прилагаемые фигуры. На фигурах крайняя левая цифра (и) ссылочного номера идентифицирует фигуру, на которой ссылочный номер появляется впервые. Использование одних и тех же ссылочных номеров на разных фигурах указывает на аналогичные или идентичные предметы.
РИС. 1 представляет собой схему системы для встраивания рекламы в веб-страницы.
РИС. 2 — схематическая диаграмма, показывающая примерный сервер веб-страницы.
РИС. 3 — схематическая диаграмма, показывающая примерную базу данных рекламных объявлений.
РИС. 4 — схематическая диаграмма, показывающая примерный сервер композиции.
РИС. 5 — схематическая диаграмма, показывающая примерный процесс встраивания рекламы в веб-страницу.
РИС. 6 — схематическая диаграмма, показывающая текст веб-страницы до и после встраивания рекламы.
РИС. 7 — блок-схема, показывающая примерный способ визуализации веб-страницы со встроенной рекламой.
РИС. 8 — схематическая диаграмма, показывающая примерное вычислительное устройство для встраивания рекламы в текст.
ПОДРОБНОЕ ОПИСАНИЕОбзор
Это раскрытие направлено на различные примерные компьютерные программы, способы и устройства для встраивания рекламы в сообщение. Например, рекламодатель может пожелать приобрести размещение рекламы на определенной веб-странице. Веб-страница может содержать текст, изображения и другие рекламные объявления, при этом одна из текстовых областей является основной текстовой областью, например, новостной статьей.Наиболее желательное размещение рекламы — внутри новостной статьи, потому что это часть веб-страницы, которую пользователь, скорее всего, прочитает. Вместо того, чтобы просто добавлять ссылку на рекламу в новостной статье, в этом раскрытии обсуждается изменение фактического содержания новостной статьи, так что реклама включается в текст новостной статьи.
Для удобства в этом раскрытии используются термины, относящиеся к конкретной реализации, а именно текст на веб-страницах.Однако идеи этого раскрытия не ограничиваются этим, но применимы к любому сообщению, текстовому, звуковому, визуальному, анимационному или в любой другой форме. В визуальное сообщение, такое как фильм или изображение, реклама может быть встроена путем размещения изображения поверх другого изображения. Например, изображение автомобиля может быть изменено, чтобы включить логотип бренда на двери автомобиля. В слуховом сообщении звук, например дверной звонок, может быть заменен звуковым знаком рекламодателя.
Примеры систем и устройств
РИС.1 показывает систематику системы , 100, для пользователя , 102, , для взаимодействия с вычислительным устройством , 104, для просмотра веб-страниц, содержащих встроенную рекламу. Вычислительное устройство , 104, может быть подключено к сети , 106, , такой как Интернет. В этой реализации контент веб-страницы предоставляется сервером веб-страницы , 108, , рекламный контент предоставляется рекламной базой данных , 110, , и сопоставление ключевого слова с рекламой, а также создание измененной веб-страницы со встроенной рекламой осуществляется выполняется сервером композиции 112 .Серверы и базы данных могут быть реализованы отдельно или поочередно объединены для выполнения описанных функций.
РИС. 2 показана схематическая диаграмма 200 примерного сервера 108 веб-страниц. Сервер веб-страниц , 108, содержит процессор , 202, и память , 204, . Память 204 содержит содержимое веб-страницы 206 . Веб-страницы могут содержать текст, изображения, видео или другой контент. Этот контент существует в форме, созданной автором веб-страницы, без встроенной рекламы.Сервер веб-страницы 108 также содержит коммуникационные соединения 208 . Коммуникационное соединение (я) , 208, сконфигурировано для предоставления содержимого веб-страницы на сервер композиции , 112, , где реклама из базы данных объявлений , 110, может быть встроена в содержимое веб-страницы , 206, . Контент веб-страницы может быть предоставлен через сеть , 106, или другое коммуникационное соединение между сервером , 108, веб-страницы и сервером композиции , 112, .
РИС. 3 показана схематическая диаграмма 300 примерной базы данных 110 рекламы. База данных , 110, содержит множество рекламных объявлений , 302, и множество описаний, , 304, . Для каждой рекламы может быть соответствующее описание. Рекламные объявления , 302, могут включать в себя информацию о продукте, такую как название, ключевое слово, описание, логотип или ссылку на страницу продукта. Описание , 304, рекламного объявления может включать в себя текст из самого рекламного объявления или текст, сгенерированный для описания содержания рекламного объявления.Например, пометка изображения, видео или аудиоконтента текстовой строкой облегчает сопоставление этого контента с текстом веб-страницы. В некоторых реализациях описание , 304, может включать в себя звуковую, визуальную или числовую информацию, связанную с рекламой. Описание , 304, может также включать слова, которые были предложены или куплены рекламодателем. В противном случае купленные слова могут не иметь отношения к рекламе. Однако возможность назначать слова без ограничения описанию 304 позволяет явно настраивать описание 304 рекламного объявления 302 .
РИС. 4 показывает схематическую диаграмму 400 примерного сервера композиции 112 . Сервер композиции включает в себя процессор , 402, , коммуникационное соединение (я) , 404, и память , 406, . В некоторых реализациях коммуникационное соединение (я) , 404, может быть сконфигурировано для приема содержимого веб-страницы и отправки измененного содержимого веб-страницы на вычислительное устройство, такое как вычислительное устройство , 104, , показанное на фиг. 1.
Память , 406, может включать в себя модуль , 408, сканирования веб-страниц, сконфигурированный для сканирования веб-страниц в реальном времени. Веб-страницы могут включать в себя веб-страницы с сервера 108 веб-страниц. В некоторых реализациях сканирование также включает в себя создание копии просканированных веб-страниц в памяти , 406, сервера композиции , 112, .
Память , 406, может также включать в себя модуль сегментации веб-страниц , 410, .Модуль , 410, сегментации веб-страницы может быть сконфигурирован для сегментации веб-страницы на несколько блоков. Каждый из блоков может быть семантической частью веб-страницы. Исходные веб-страницы могут содержать такие элементы, как изображения, видео, баннеры, контактную информацию, руководства по навигации и другую рекламу. Пользователи рассматривают веб-страницу как несколько различных семантических объектов, а не как один объект. Пространственные и визуальные подсказки позволяют пользователям разделить веб-страницу на несколько семантических частей. Многочисленные элементы могут мешать машинному анализу основного текста веб-страницы. Однако машина может также разделить веб-страницу на семантические части, используя информацию, закодированную на языке разметки, лежащем в основе веб-страницы.
В одной реализации модуль 410 сегментации веб-страницы использует алгоритм сегментации страницы на основе видения (VIPS) для сегментации веб-страницы на блоки. Алгоритм VIPS настроен для извлечения семантической структуры веб-страницы на основе визуального представления веб-страницы. Алгоритм VIPS извлекает структурную информацию из дерева объектной модели документа веб-страницы.Дерево объектной модели документа веб-страницы идентифицирует дискретные объекты на веб-странице на основе кодировки или тегов на языке разметки, который описывает веб-страницу. Далее находятся разделители между блоками экстракции. Разделители могут обозначать горизонтальные или вертикальные линии на веб-странице, которые не пересекают ни один из блоков. Таким образом, веб-страница может быть представлена семантическим деревом, в котором каждый листовой узел дерева соответствует блоку веб-страницы. Блок веб-страницы можно разделить на более мелкие блоки.Таким образом, реализации алгоритма VIPS могут повторяться для рекурсивного разделения веб-страницы из более крупных блоков на более мелкие блоки.
Модуль 410 сегментации веб-страницы может также идентифицировать основной текстовый блок веб-страницы. Основной текстовый блок может быть текстовым блоком, который содержит больше слов, чем другой текстовый блок на той же веб-странице, и находится в фокусе веб-страницы. Фокус веб-страницы может быть определен путем идентификации текстового блока, ближайшего к центру веб-страницы.В других реализациях фокус веб-страницы может определяться тегами или метками на языке разметки, связанном с блоком. В некоторых реализациях основной текстовый блок идентифицируется путем фильтрации других блоков, таких как блоки, содержащие изображения, рекламные объявления или нетекстовый контент. Блоки, содержащие изображения или рекламу, могут быть идентифицированы путем анализа меток, ссылок или аналогичной информации на языке разметки, описывающем блоки.
Память , 406, может также включать в себя модуль извлечения предложений , 412, .Модуль извлечения предложений , 412, идентифицирует отдельные предложения из текста веб-страницы. В некоторых реализациях предложения извлекаются только из текста основного текстового блока. После получения всех предложений из блока веб-страницы, модель статистического анализа применяется к каждому из предложений, чтобы сгенерировать лексическое дерево синтаксического анализа для каждого предложения. В целом модель статистического анализа определяет условную вероятность для каждого дерева синтаксического анализа кандидатов, созданного на основе предложения.Сам синтаксический анализатор — это алгоритм, который ищет лучшее дерево, которое максимизирует вероятность результирующего предложения.
Память , 406, также может включать в себя модуль , 414, сопоставления ключевых слов объявления. Модуль , 414, сопоставления рекламных и ключевых слов принимает ключевые слова с веб-страницы и сопоставляет эти ключевые слова с рекламой. В некоторых реализациях ключевые слова веб-страницы идентифицируются путем анализа текста веб-страницы. В частности, анализируемый текст веб-страницы может быть текстом основного текстового блока.Модуль , 414, сопоставления рекламных объявлений и ключевых слов связывает описания 304 рекламных объявлений 302 , хранящиеся в базе данных объявлений , 110, , с ключевыми словами с веб-страницы на основе журналов пользователей, кластеризации слов или правил торгов.
Журналы пользователей могут быть записями других веб-страниц, просмотренных тем же пользователем. Использование журналов пользователя для идентификации потенциально релевантных рекламных объявлений может быть лучше, чем использование одних только ключевых слов, поскольку пул информации, используемый для идентификации потенциально релевантных рекламных объявлений, основан на фактическом поведении пользователя. Если пользователь при просмотре веб-страницы определенного типа выбрал рекламу, вполне вероятно, что аналогичная реклама будет интересна пользователю, когда пользователь снова просматривает веб-страницу этого типа. Например, если пользователь нажал на рекламу авиакомпании при просмотре веб-страницы о путешествиях, вероятно, что пользователя заинтересует реклама авиакомпаний при просмотре других веб-страниц о путешествиях.
Журналы пользователей могут быть проанализированы для определения взаимосвязей между веб-страницами и рекламными объявлениями, представляющими интерес для пользователя.В некоторых реализациях метод кластеризации на основе плотности используется для кластеризации информации, содержащейся в журналах пользователя. Одним из примеров метода кластеризации на основе плотности является алгоритм пространственной кластеризации приложений с шумом на основе плотности (DBSCAN). Алгоритм DBSCAN использует структуру пространственной индексации для поиска точек в данных на определенном расстоянии от центральной точки кластера. Все кластеры, состоящие из менее чем минимального количества точек, считаются «шумом» и отбрасываются.
Кластеризация слов определяет вероятную связь между двумя словами на основе частоты, с которой эти слова встречаются вместе в справочном корпусе текста. Из справочного корпуса текста может быть вычислено попарное сходство между всеми существительными, всеми глаголами и всеми прилагательными / наречиями. Справочным корпусом текста могут быть, например, архивные газетные статьи. Список похожих слов, включая меру сходства, создается для каждого слова в корпусе текста на основе попарного сходства.Рекламное объявление может быть связано с ключевым словом с веб-страницы, когда слова в описании рекламы находятся среди слов, идентифицируемых как похожие на ключевое слово.
Правила ставок могут связать рекламу с ключевым словом в зависимости от того, сколько денег рекламодатель готов заплатить за привязку к данному ключевому слову. В одной реализации правил ставок рекламодатель, который делает самую высокую ставку, может создать связь между ключевым словом и рекламой.
Связь между ключевым словом и рекламным объявлением может быть основана на сочетании пользовательских журналов, кластеризации слов и правил торгов.Анализ каждого из пользовательских журналов, кластеризации слов и правил ставок может генерировать три различных значения силы ассоциации. Каждое из трех значений силы ассоциации может быть объединено в линейно взвешенное или среднее слияние для создания единого комбинированного значения силы ассоциации. В некоторых реализациях весовой коэффициент может быть изменен на основе эмпирического наблюдения соответствия рекламы и ключевых слов. Рекламное объявление с наивысшим комбинированным значением силы ассоциации может быть связано с ключевым словом.В некоторых реализациях более одной рекламы может быть связано с одним ключевым словом, например, когда более одной рекламы имеет одинаковое значение силы ассоциации. Также возможно создать ассоциацию между ключевым словом и множеством рекламных объявлений, имеющих, например, первое, второе и третье наивысшие комбинированные значения силы ассоциации.
Память , 406, также может включать в себя модуль , 416, составления рекламного предложения. Рекламное объявление, выбранное для вставки в предложение, может быть рекламным объявлением, идентифицированным модулем , 414, сопоставления рекламных и ключевых слов.Предложение уже было разложено в дерево синтаксического анализа модулем 412 извлечения предложения. Следовательно, задача модуля , 416, составления рекламного предложения состоит в том, чтобы определить, как объединить предложение и рекламное объявление. Реклама может содержать элементы, отличные от текста; однако с целью определения того, как объединить предложение с рекламой, анализируются только текстовые элементы рекламы или рекламный текст. Рекламный текст вставляется в дерево синтаксического анализа, и создается новое дерево синтаксического анализа.Это повторяется для создания нескольких деревьев синтаксического анализа, каждое дерево синтаксического анализа соответствует вставке рекламного текста в другое место в исходном предложении. Благодаря этому процессу создается множество различных композиций.
Память , 406, также может включать в себя модуль 418 фильтрации состава. Модель 418 фильтрации композиции выбирает одну композицию из множества различных композиций, созданных модулем 416 композиции рекламного предложения.Фильтрация основана на методах сглаживания. Каждое новое дерево синтаксического анализа, созданное путем вставки рекламного текста в исходное предложение, имеет вероятность быть естественным и грамматически правильным предложением. Вероятность слов и словосочетаний определяется путем сравнения с языковой моделью. Произведение вероятности слов и фраз каждого нового синтаксического дерева — это вероятность предложения в целом. Из множества потенциальных композиций композиция с наибольшей вероятностью по сравнению с другими потенциальными композициями, вероятно, будет соответствовать естественному и грамматически правильному предложению. Таким образом, модуль , 418, фильтрации состава выбирает новое дерево синтаксического анализа с наибольшей вероятностью. Таким образом, сервер композиции , 112, определяет, где в предложении вставить рекламу.
Память , 406, также может включать в себя модуль 420 визуализации веб-страниц. Этот модуль 420 отображает окончательные результаты встраивания рекламы. Текст веб-страницы изменяется так, что композиция, идентифицированная модулем 418 фильтрации состава, отображается пользователю , 102, вместо отображения исходного текста веб-страницы.В некоторых реализациях это достигается за счет того, что сервер композиции , 112, перехватывает передачи веб-страниц с сервера , 108, веб-страниц и модифицирует веб-страницу путем встраивания рекламы перед доставкой веб-страницы на вычислительное устройство , 104, .
В некоторых реализациях сервер композиции , 112, содержит языковую модель , 422, . Языковая модель , 422, может содержать большой корпус текста, такой как корпус ссылок, описанный выше.В некоторых реализациях большой корпус текста автоматически собирается с других веб-страниц. Этот корпус текста предоставляет набор данных, которые могут быть проанализированы для определения статистической вероятности порядка слов и отношений между словами. Если сочетание слов или фразы встречается в корпусе текста, вполне вероятно, что сочетание слов или фразы является естественным сочетанием. Обращаясь к языковой модели 422 , машина может создавать новые предложения и порядки слов, которые могут быть естественными и грамматически правильными.Языковая модель , 422, может зависеть от темы. Корпус текста, основанный на экономических публикациях, может дать более точные оценки естественных и грамматических предложений для содержания веб-страницы, связанного с экономикой, чем корпус текста, основанный на общих новостных статьях. Например, сервер композиции , 112, может содержать множество языковых моделей, каждая из которых относится к разным темам. В некоторых реализациях сервер композиции , 112, может идентифицировать тему веб-страницы и выбирать языковую модель, которая соответствует теме.
Иллюстративный процесс
Показанный на фиг. 5 — процесс 500 для встраивания рекламы в предложение с веб-страницы. Сервер веб-страниц 108 является источником исходной веб-страницы 502 . Исходная веб-страница , 502, может содержать блок панели инструментов, несколько рекламных блоков Ad 1 , Ad 2 и несколько текстовых блоков. Исходная веб-страница , 502, может быть сегментирована на блоки с помощью процесса, такого как описанный выше в отношении модуля 410 сегментации веб-страниц.Сегментация веб-страницы , 504, является преимуществом, поскольку она может идентифицировать отдельный текстовый блок для дальнейшего анализа, таким образом уменьшая объем информации, которая должна быть обработана, чтобы встроить рекламное объявление в предложение.
Предложение извлечено с веб-страницы 506 . Проиллюстрировано извлечение только одного предложения; тем не менее, более одного предложения или даже все предложения с веб-страницы могут быть извлечены и проанализированы отдельно.
Затем процесс 500 переходит к созданию статистического дерева синтаксического анализа предложения 508 .На этой иллюстрации первые пять слов предложения (например, другие / популярные / коммуникации / системы / подобные) и слово «есть» показаны как узлы дерева. Каждый узел в дереве обозначает одно слово. Статистический древовидный анализ предложения комбинируется с рекламой из базы данных объявлений , 110, . Рекламное объявление или рекламные объявления, выбранные из рекламной базы данных , 110, для комбинации с предложением из анализа , 508, статистического дерева, могут быть выбраны способом, аналогичным методу, выполняемому модулем , 414, сопоставления рекламных объявлений и ключевых слов. На этой иллюстрации реклама представлена словом «Microsoft».
При вставке рекламного объявления рядом с узлом для слова «подобное» создается модифицированное статистическое древовидное представление предложения. Эта позиция для вставки рекламы выбирается через предложение-рекламу фильтрации 512 . Процесс фильтрации 512 предложения-рекламы может быть аналогичен процессу, выполняемому модулем 418 фильтрации состава.Языковая модель , 514, предоставляет критерий фильтрации для фильтрации предложения-рекламы 512 . Другими словами, место вставки для «Microsoft» основано на том, где в извлеченном предложении это наиболее вероятно согласно языковой модели 514 . Языковая модель , 514, , показанная на фиг. 5 может быть такой же, как языковая модель 422 , показанная на фиг. 4. В некоторых реализациях языковая модель может существовать отдельно от сервера композиции , 112, .
РИС. 6 показывает более подробно статистический анализ дерева , 508, и фильтрацию рекламы предложений 512 на фиг. 5. Процесс , 600, , показанный на фиг. 6 начинается с предложения 602 , извлеченного с веб-страницы. Это то же предложение, что и на фиг. 5. Лексическое дерево синтаксического анализа 604 предложения создается с помощью модели синтаксического анализа, такой как процесс Маркова порядка 0 -го порядка . Модель синтаксического анализа идентифицирует лексическое заглавное слово или заглавное слово.В некоторых реализациях заглавным словом является ключевое слово из предложения. На этой иллюстрации заглавным словом является «коммуникация», которое показано сидящим на вершине лексического дерева синтаксического анализа 604 . Вероятность заглавного слова — P H (H | P, h). Где H — это начальный дочерний элемент фразы, P H — это вероятность H, P — родительский элемент заголовочного слова, а h — заголовочное слово.
Модификаторы генерируются справа от заголовка с вероятностью, показанной как:
∏i = 1… m + 1PH (Ri (ri) | P, h, H) .
R m + 1 (r m + 1 ) определяется как STOP — символ STOP добавляется к словарю нетерминалов, и модель анализа перестает генерировать правые модификаторы, когда генерируется символ STOP. Р 1 . . . R m — правые модификаторы H. Модификаторы также генерируются слева от заглавного слова с вероятностью, показанной как:
∏i = 1… n + 1PL (Li ( li) | P, h, H).
Для модификаторов, созданных слева, L n + 1 (l n + 1 ) определяется как STOP.Л 1 . . . L n являются левыми модификаторами H. Либо n, либо m может быть 0, а n = 1 = 0 для унарных правил.
Учитывая лексическое дерево синтаксического анализа 604 , полученное для предложения 602 и рекламное слово (например, Microsoft), рекламное слово вставляется рядом с узлом в лексическом дереве синтаксического анализа 604 . В некоторых реализациях рекламное слово вставляется рядом с каждым узлом в дереве лексического синтаксического анализа , 604, . Рекламное слово может быть вставлено рядом с узлом, соответствующим слову «подобное» , 606, , узлом, соответствующим слову «другой», , 608, , узлом, соответствующим слову «системы», , 610, или любым другим узел 612 .Детали других возможных лексических деревьев синтаксического анализа не показаны на фиг. 6 для краткости.
Одно дерево синтаксического анализа выбирается из нескольких деревьев синтаксического анализа 606 — 612 , которые создаются путем объединения предложения и рекламного слова. В одной реализации выбор основан на вероятности вставки, рассчитанной с помощью языковой модели n-граммов. В этом примере выбранное дерево синтаксического анализа является лексическим деревом синтаксического анализа , 606, в левой части фиг. 6.
В модели языка n-грамм вероятность предложения P (s) выражается как произведение вероятности слов, составляющих предложение, причем вероятность каждого слова зависит от идентичности последних n-I слов.Таким образом, для предложения s, содержащего слова w i . . . w l , вероятность предложения P (s) отображается как:
P (s) = ∏i = 1lP (wi | w1i-1) ≈∏i = 1l P (wi | w1-n + 1i-1)
Обычно n принимается равным 2 или 3, что соответствует модели биграмм или триграмм соответственно. Каждое возможное предложение, сгенерированное путем вставки рекламного слова в дерево лексического синтаксического анализа , 604, , будет иметь вероятность, связанную с этим предложением. Из возможных предложений в этом примере 606 — 612 , лексическое дерево синтаксического анализа 606 в левой части фиг.6 имеет вероятность выше, чем любое из других возможных деревьев лексического синтаксического анализа 608 — 612 . Таким образом, этот алгоритм выбирает точку вставки для рекламного слова с максимальным значением P (s). В этом примере «Другие популярные системы связи, такие как Microsoft. . . « 606 более вероятен, чем другие популярные системы связи Microsoft, такие как. . . » 608 или« Другие популярные системы связи, подобные Microsoft. . . ” 610 .
Как обсуждалось ранее, реклама может быть больше, чем просто рекламное слово.В этом примере рекламным словом является «Microsoft», но реклама содержит текст «Microsoft Outlook, MSN Messenger» и изображения, представляющие MICROSOFT OUTLOOK ™ и MSN MESSENGER ™. Реклама также содержит гиперссылки или ссылки на другой веб-сайт. Ссылки обозначаются подчеркиванием «Microsoft Outlook» и «MSN Messenger». Исходное предложение , 602, модифицируется путем включения рекламного объявления в позицию, указанную языковой моделью n-граммов, в результате чего получается новое предложение , 614, , которое представляет собой композицию исходного предложения , 602, и рекламного объявления.
Примерная блок-схема
Показанная на фиг. 7 является примерной блок-схемой процесса , 700, для создания веб-страницы со встроенной рекламой. Для простоты понимания способ , 700, размечен как отдельные этапы, представленные как независимые блоки на фиг. 7. Однако эти отдельно очерченные этапы не следует истолковывать как обязательно зависящие от порядка их выполнения. Порядок, в котором описан процесс, не предназначен для толкования как ограничение, и любое количество описанных блоков процесса может быть объединено в любом порядке для реализации метода или альтернативного метода.Более того, также возможно, что один или несколько из предусмотренных этапов будут пропущены.
Блок-схема для процесса 700 предоставляет пример метода, который может выполняться сервером композиции 112 . В других реализациях процесс может выполняться другим специализированным устройством, отличным от сервера композиции , 112, . В качестве альтернативы процесс , 700, также может выполняться на множестве устройств.
Показанный на этапе 702 процесс 700 идентифицирует веб-страницу.Веб-страница может храниться в памяти сервера веб-страниц, такого как сервер , 108, веб-страницы, показанный на фиг. 2.
Показанный в блоке 704 процесс 700 сканирует веб-страницу. Сканирование веб-страницы может выполняться любой программой или сценарием, которые просматривают веб-страницы коллекции, такие как World Wide Web, методическим, автоматическим способом. Некоторые примеры поисковых роботов — это программы, используемые для автоматического индексирования веб-страниц для поисковых систем.
Показанный на этапе 706 процесс 700 сегментирует веб-страницу.Веб-страница может быть сегментирована с помощью алгоритма машинного зрения, такого как описанный выше в отношении модуля , 410, сегментации веб-страницы. В некоторых реализациях алгоритмом машинного зрения является алгоритм VIPS.
Показанный в блоке 708 процесс 700 идентифицирует основной текстовый блок. Основной текстовый блок может быть идентифицирован после сегментации веб-страницы. В других реализациях, которые не сегментируют веб-страницу, основной текстовый блок может быть извлечен с веб-страницы без сегментации.Идентификация основного текстового блока может выполняться аналогично тому, как описано выше в отношении модуля , 410, сегментации веб-страницы.
Показанный в блоке 710 процесс 700 извлекает предложения из основного текстового блока. Извлечение предложений может быть аналогично извлечению предложения 506 , показанному на фиг. 5. В некоторых реализациях отдельные предложения идентифицируются по меткам окончания предложения (например,грамм. «.», «?», «!» И т. Д.) И начальные заглавные буквы. Извлечение предложений может включать в себя выделение отдельных предложений как отдельных элементов для дальнейшей обработки или анализа.
Показанный на этапе 712 процесс 700 анализирует предложения. В некоторых реализациях синтаксический анализ предложений включает идентификацию отдельных слов в предложениях. Первым шагом синтаксического анализа предложений может быть разложение предложений в дерево с использованием языковой модели.Синтаксический анализ предложений также может быть выполнен путем создания дерева синтаксического анализа предложения, аналогичного лексическому дереву синтаксического анализа , 604, , обсужденному выше в отношении фиг. 6.
Показанный на этапе 714 процесс 700 идентифицирует пару ключевых слов объявления. Идентификация пары рекламно-ключевое слово может выполняться аналогично тому, как это делает модуль , 414, сопоставления рекламно-ключевого слова. В некоторых реализациях ключевое слово связано с конкретным предложением, и, таким образом, связывание рекламного объявления с ключевым словом также связывает рекламное объявление с предложением.Если данное ключевое слово встречается в предложении или блоке текста более одного раза, реклама может иметь связи с несколькими местами в предложении или несколькими предложениями в блоке текста. Подобно другим типам рекламы, с помощью встроенной рекламы можно показывать одну и ту же рекламу несколько раз на одной веб-странице.
Показанный на этапе 716 процесс 700 создает композиции из рекламного объявления и предложения. Композиции могут быть созданы способом, аналогичным описанному выше в отношении модуля , 416, составления рекламного предложения.Обычно, но не всегда, для данной пары ключевое слово-предложение создается несколько композиций. Композиции могут создавать новые предложения, вставляя рекламу в различные места в исходном предложении, аналогичном тому, что проиллюстрировано на фиг. 6. Выбор того, где и как вставить рекламу в исходные предложения, может производиться случайным образом и / или без знания грамматики или правил построения предложений. Таким образом, вполне возможно, что многие композиции покажутся неестественными или грамматическими для человеческого читателя.
Показанный на этапе 718 процесс 700 выбирает грамматическую композицию. Если создается только одно сочинение, оно будет автоматически выбрано как грамматическое. Если есть несколько композиций для выбора, процесс выбора может быть аналогичен тому, который выполняется модулем 418 фильтрации композиции. Процесс выбора грамматической композиции пытается выбрать одно предложение, которое будет казаться грамматическим и естественным для человеческого читателя, из нескольких предложений, созданных с помощью алгоритма, реализованного на вычислительном устройстве.
Показанный на этапе 720 процесс 700 визуализирует веб-страницу со встроенной рекламой. Рендеринг может быть аналогичен рендерингу, выполняемому модулем , 420, рендеринга веб-страницы. Встраивание рекламы в исходный текст веб-страницы создает встроенную рекламу. Реклама представляется пользователю в части веб-страницы, которую пользователь, вероятно, будет просматривать, и реклама интегрирована с содержимым веб-страницы, так что пользователю трудно игнорировать рекламу.Как обсуждалось в отношении изменения предложения 614 , текст, изображения, видео, гиперссылки или другая реклама могут быть встроены в веб-страницу.
Примерное вычислительное устройство
Фиг. 8 — это блок-схема, показывающая примерное вычислительное устройство , 800, для создания встроенной рекламы. Вычислительное устройство , 800, может быть сконфигурировано как любая подходящая система, способная создавать композицию рекламного предложения. В одной примерной конфигурации система содержит по меньшей мере один процессор , 802 и память , 804, .Процессор , 802, может быть реализован соответствующим образом в аппаратных средствах, программном обеспечении, встроенном программном обеспечении или их комбинациях. Программные или программно-аппаратные реализации процессора , 802, могут включать в себя выполняемые компьютером или машиной инструкции, написанные на любом подходящем языке программирования, для выполнения различных описанных функций.
Например, вычислительное устройство , 800, иллюстрирует архитектуру этих компонентов, находящихся в одной системе или одном сервере, которые могут быть коммуникативно связаны с сетью, такой как сеть , 106, , показанная на фиг.1. В качестве альтернативы, эти компоненты могут находиться в нескольких других местах, на серверах или системах. Например, все компоненты могут существовать на стороне клиента. Кроме того, два или более из проиллюстрированных компонентов могут объединяться в один компонент в одном месте. Проиллюстрированные компоненты могут также находиться в вычислительном устройстве без подключения к сети, например в автономной базе данных. Вычислительное устройство , 800, не ограничивается сервером композиции , 112, .В некоторых реализациях вычислительное устройство , 800, может быть аналогично вычислительному устройству , 104, , показанному выше на фиг. 1.
Память 804 может хранить программы инструкций, которые могут быть загружены и исполняться на процессоре, а также данные, сгенерированные во время выполнения этих программ. В зависимости от конфигурации и типа вычислительного устройства , 800, , память , 804, может быть энергозависимой (например, ОЗУ) и / или энергонезависимой (например, ПЗУ, флэш-память и т. Д.).). Вычислительное устройство , 800, может также включать в себя дополнительное съемное хранилище , 806, и / или несъемное хранилище , 808, , включая, но не ограничиваясь, магнитное хранилище, оптические диски и / или ленточное хранилище. Дисковые накопители и связанные с ними машиночитаемые носители могут обеспечивать энергонезависимое хранилище машиночитаемых инструкций, структур данных, программных модулей и других данных для устройств связи.
Обращаясь к содержимому памяти , 804, более подробно, память , 804, может включать в себя операционную систему , 810, и модуль , 416, составления рекламных предложений для создания композиций рекламных объявлений и предложений.Память , 804, также может, в некоторых реализациях, содержать языковую модель , 812, , которая может быть аналогичной языковой модели , 422, или языковой модели , 514, . Множественные языковые модели, такие как языковая модель 812 , языковая модель 422 и языковая модель 514 , могут быть одинаковыми или разными языковыми моделями. Также вычислительное устройство , 800, может включать в себя базу данных, размещенную на процессоре , 802 (не показан).
Компьютерные носители данных включают в себя энергозависимые и энергонезависимые, съемные и несъемные носители, реализованные с помощью любого метода или технологии для хранения информации, такой как машиночитаемые инструкции, структуры данных, программные модули или другие данные. Память , 804, , съемное хранилище и несъемное хранилище — все это примеры компьютерных носителей. Дополнительные типы компьютерных носителей информации, которые могут присутствовать, включают, помимо прочего, RAM, ROM, EEPROM, флэш-память или другие запоминающие устройства, CD-ROM, универсальные цифровые диски (DVD) или другое оптическое хранилище, магнитные кассеты, магнитные лента, запоминающее устройство на магнитном диске или другие магнитные запоминающие устройства, или любой другой носитель, который может использоваться для хранения желаемой информации и к которому может получить доступ вычислительное устройство 800 .
Вычислительное устройство , 800, может также содержать коммуникационное соединение (я) , 812, , которое позволяет процессору , 802 связываться с серверами, пользовательскими терминалами и / или другими устройствами в сети. Коммуникационное соединение (я) 812 является примером коммуникационной среды. Коммуникационные среды обычно включают машиночитаемые инструкции, структуры данных и программные модули. В качестве примера, но не ограничения, коммуникационные среды включают в себя проводные среды, такие как проводная сеть или прямое проводное соединение, и беспроводные среды, такие как акустические, радиочастотные, инфракрасные и другие беспроводные среды.Термин «машиночитаемые носители», используемый в данном документе, включает как носители данных, так и средства связи.
Вычислительное устройство 800 может также включать в себя устройство (а) ввода 814 , такое как клавиатура, мышь, перо, устройство голосового ввода, сенсорное устройство ввода, перо и т.п., а также устройство (а) вывода, 816 , например, дисплей, монитор, динамики, принтер и т. Д. Все эти устройства хорошо известны в данной области техники и не нуждаются в подробном обсуждении.
Предмет, описанный выше, может быть реализован в аппаратном обеспечении, программном обеспечении или в аппаратном и программном обеспечении.Хотя реализации встроенной рекламы были описаны на языке, характерном для структурных особенностей и / или методологических действий, следует понимать, что предмет, определенный в прилагаемой формуле изобретения, не обязательно ограничивается конкретными функциями или действиями, описанными выше. Скорее, конкретные особенности и действия раскрыты как примерные формы примерных реализаций создания встроенной рекламы. Например, методологические действия не обязательно должны выполняться в порядке или комбинациях, описанных в данном документе, и могут выполняться в любой комбинации из одного или нескольких действий.
Пишем ясно и лаконично | UAGC Writing Center
Хороший стиль письма предполагает не только использование правильной пунктуации, грамматики и структуры (синтаксиса) предложения, но и четкое и лаконичное письмо.
Четкое письмо
Выберите слово, которое наиболее четко передает ваш смысл
Английские слова обычно имеют два типа значений: денотативное значение (описательное словарное определение слова) и коннотативное значение (эмоциональное воздействие слова).Коннотация может быть положительной или отрицательной. Например, слова «стройный», «тонкий» и «тощий» имеют одно и то же денотативное значение, но очень разные коннотации.Всегда следуйте этому, тому, этим и тем, у кого есть существительное
- Непонятно: возьму. (Это что?)
- Ясно: я возьму эту собаку.
- Непонятно: я не хочу этого. (Что хотите?)
- Ясно: я не хочу эту книгу.
Лаконичное письмо
Избавьтесь от ненужных фраз и повторов.
- Верно: мы будем дома через десять дней.
- Неправильно: мы будем дома через десять дней.
Используйте ясный и простой язык.
- Верно: я заметил много сорняков вокруг здания.
- Неправильно: Мое внимание привлекло огромное количество нежелательной растительности, окружающей периферию этого объекта.
Пишите активным голосом.
- Правильно: на этой неделе комитет решил проголосовать по этому вопросу.
- Неправильно: решение о голосовании по вопросу было принято комитетом на этой неделе.
Сокращайте многословные фразы.
- Правильно: Джон позвонит мне, если сможет пойти.
- Неправильно: Джон сообщит мне, если сможет уйти и отправиться в путь.
Избегайте начинать предложения с «есть», «есть» или «это есть».
- Верно: четыре офицера подчиняются капитану.
- Неправильно: четыре офицера подчиняются капитану.
Исключите лишние существительные.
- Правильно: Луиса интересовала обработка данных.
- Неправильно: Луиса интересовала область обработки данных.
Удалите слова-заполнители, такие как «то», «из» или «вверх».
- Правильно: я сказал, что устал.
- Неправильно: я сказал, что устал.
- Правильно: я сошел с тротуара.
- Неправильно: я сошел с обочины.
- Правильно: я поднялся по лестнице.
- Неправильно: я поднялся по лестнице.
Выпуск данных о поверхностных осадочных породах восточного побережья, упрощенное описание обработки dbSEABED
Серия данных геологической службы США 118, usSEABED: Выпуск данных об отложениях на шельфе Атлантического побережья, версия 1.0
На главную | Содержание | Карта сайта | Введение | насморское дно | dbSEABED | Каталог данных | Цитированные ссылки | Контакты | Благодарности | Часто задаваемые вопросы
Упрощенное описание обработки dbSEABED
Особенностью dbSEABED является его способность анализировать словесные описательные данные, такие как «коричневый мелкий песок с обильными ракушками; водоросли и небольшая галька; запах h3S.Эти типы данных хранятся в их исходных терминах, хотя некоторые сокращения и кодирование необходимы. Таким образом, dbSEABED не является анализатором естественного языка даже для конструкций именных фраз, таких как приведенное выше описание. Ниже приводится упрощенное описание функций синтаксического анализа.
Большинство описаний состоят из кванторов, модификаторов и объектов (qmO) и могут быть записаны в виде линейных выражений:
q 1 * [м 1 ] O 1 + q 2 * [m 2 ] O 2 + q 3 * [м 3 ] O 3 … = образец
В большинстве случаев фракция отложений представляет собой всю пробу, но dbSEABED явно записывает, где это, а где нет, в выходных данных. В предыдущем примере, который в кодировке dbSEABED равен
brn fne- snd wi_ab / shls seagrs + сом / pbls; whif_of / h3s
, где «-» в модификаторах указывает на измененный объект, а «/» в квантификаторах указывает на количественно определенный объект. Использование сокращений помогает отличать данные от метаданных в файлах данных и делает описания короче, проще в обработке и удобнее для чтения.Код qmO для текстуры тогда
m (fne) O (snd) + q (wi_ab) O (shls) + q (som) O (pbls)
, где «коричневый», «водоросли» и «h3s» не показаны, потому что они нейтральны по текстуре. Каждому текстурному объекту назначается размер зерна, который может быть представлен как членство в нечетком наборе для диапазона размеров зерна или может быть процентным соотношением одного размера (четкий набор). Определение размера зерен берется из словаря и обычно основывается на опубликованных шкалах, таких как (Wentworth, 1922) и Единая система классификации почв (USCS)), на анализе компонентов отложений региона или на седиментологическом опыте одного или больше людей.Размер зерна — это средний размер зерна, наблюдаемый для отложений, которые были помечены просто как «песок», что составляет 1,5 фи. При применении модификатора размер зерна регулируется. В случае модификатора «мелкий» средний размер зерна песка становится 2,5 фунта на квадратный дюйм. «Ракушки» имеют текстурное значение, обычно мелкий гравий — 2 фи, но, конечно, в зависимости от вида и сохранности. «Гальке» присвоен размер зерна по шкале Вентворта.
Кванторы «изобилие» и «некоторые» присваивают веса частям «скорлупа» и «галька» и регулируют принадлежность назначенных размеров зерен фи.Эти принадлежности указаны в словаре: «обильный» 0,5, «некоторый» 0,3. Неопределенному количеству «песок» обычно присваивается 1.0. После нормализации до 1,0 компоненты «песок», «ракушка» и «галька» имеют веса 0,56, 0,28 и 0,17 соответственно. (Примечание: нормализация зависит от синтаксиса, будь то синтаксис ODP , значимый сзади, с увеличением веса вправо; значимые военно-морские данные спереди; или синтаксис с постоянным значением, распространенный в экологических исследованиях. указан в dbSEABED.) Для стандартных классов текстуры доля гравия («ракушка + галька») составляет 0,4, песок 0,6 и грязь 0,0. (Примечание: пропорции ила и глины не могут быть определены по визуальным описательным данным.)
Для оценки среднего размера зерна для всего образца
0,56 * (2,5 фи) + 0,28 * (- 2 фи) + 0,17 * (- 3,5 фи)] = 0,25 фи
Об этом сообщается проанализированным выходам (_ PRS ) . Существование ракушки и камешков также отмечается в выходных данных компонента «shell» (_CMP) с относительной принадлежностью 0.28 и 0,17.
Аналогичный процесс выполняется последовательно для других параметров, для уплотнения (здесь нет информации, осадок будет считаться рыхлым), таких свойств, как «водоросли» и цвета. «Коричневый» вызовет вывод цветового кода Манселла 5YR 4/4 посредством калиброванных процессов, описанных в Jenkins, 2002.
Цифры и результаты, приведенные выше, являются только примерами, и их объяснение упрощено. Итерационные сравнения сотен словесных описаний друг с другом и с результатами лабораторных анализов показали, что точность составляет около 1 стандартного отклонения в диапазоне +/- 2 фи.
Большинство исходных лингвистических данных достаточно хорошо организованы, хотя существует множество описательных лингвистических структур, знакомых геологам, экологам, биологам, водолазам и другим исследователям морского дна. Для использования в dbSEABED описания отложений и данные анализа не должны быть точными или соответствовать определенной схеме. Программа dbSEABED справляется с широким набором словарей (например, иностранный язык, коды USACE , коды типа NOS Bottom Type), а также с множеством лингвистических структур и способов присоединения величин.В настоящее время в словаре синтаксического анализа определено более 5300 терминов.
Кроме того, данные не обязательно должны быть абсолютно полными. Программа dbSEABED анализирует, какие данные есть, выдавая результаты только в том случае, если данные достаточно полны, чтобы быть надежными, при этом отклоняя (и сообщая для будущего внимания) неполные или ошибочные структуры.
Каждое описание близко к своей исходной форме и структуре, но закодировано в фонетически понятных терминах, которые включают связи между квантификаторами, модификаторами и объектами.Кодировщики данных выбирают между различными типами данных для организации данных, поэтому программа dbSEABED может быстро преобразовывать слова в значения. В следующей таблице представлены типы данных, используемые в выходных файлах usSEABED:
Таблица A: Ключ к темам данных в выходных файлах usSEABED |
Сокращение | Значение |
---|---|
ACU | Акустические свойства |
БИО | Биота |
CMP | Анализ состава донных отложений |
COL | Цвет |
GRZ | Результаты анализа крупности |
GTC | Геотехнические свойства |
LTH | Литология |
MSL | Мультисенсорный регистратор керна |
ПЭТ | Петрология зерна |
SFT | Описание типов морского дна |
TXG | Статистика графических текстур |
TXR | Статистика текстуры |
Программа dbSEABED содержит тезаурус, в котором различные термины, используемые для описания морского дна, имеют литологические, текстурные и биологические классы и веса.Модификаторам и квантификаторам присваиваются относительные веса, а значения присваиваются другим категориям по мере необходимости.
Обслуживаются несколько специальных семантических структур, в частности, совместная структура изобилия, такая как: «snd som / {pbl + shl + blk- nods}», где галька, раковина и конкреции составляют 0,3 в количестве («сом»). Кроме того, компонент может быть в виде доли какой-то специальной фракции, такой как: «snd // acid_residu», что фактически означает долю некарбонатного песка.
В парсинг входит ряд устройств контроля качества.Если термин не распознан в словаре, процесс прерывается. Процесс также прерывается, если взвешивание компонентов из определенных наблюдений, таких как подсчет зерен, не дает в сумме 100%. Если описание слишком сложное (в настоящее время определяется как более 32 терминов), оно не анализируется. О встречающихся проблемах сообщается в файл диагностики для последующего внимания и, возможно, исправления. Различают такие омонимические термины, как «плотный» для консолидации и «изобилие» (как «dens (phy)», «dens (ab)»). термины, помеченные в словаре как «значение неизвестно», приведут к сбою синтаксического анализа.Термины, которые имеют особое значение в одном опросе, такие как «железо» в данных DSDP , также специально отмечены («железо (dsdp)»). Данные XRD не анализируются, поскольку они не считаются достаточно надежными по сравнению с петрологическим подсчетом или визуальным описанием. В настоящее время расположение структур в ядре (например, «ниже желтого слоя») и градиенты (например, «повышение до») не анализируются.
Дополнительную информацию о программном обеспечении dbSEABED можно получить из ряда статей, перечисленных в разделе «Часто задаваемые вопросы».
Образный язык, использованный в скромном предложении
Свифт, Джонатан. «Скромное предложение». В Линн З. Блум, изд. The Essay Connection: Readings for Writers: Heath, 1991. Voltaire, Candide, or, Optimism. Пер. Тео Каффе. Penguin Classics Deluxe изд. Penguin, 2005. Задачи: Студенты продемонстрируют способность: Студенты писать интерпретацию литературного произведения, основанную на
. Ваше предложение должно быть на 1-2 страницах в форме критического эссе с цитатами в тексте, если это необходимо.Эссе должно иметь введение, как минимум, три основных абзаца и заключение. Ваше предложение должно демонстрировать эксперименты со стилем и юмором. Например, вы можете использовать повторение или восклицательный знак.
Как Джонатан Свифт использует этос, логотипы и пафос в «Скромном предложении»? … Строки речи богаты образным языком, включая метафоры: «Стена огня поднимается, и в пепле я вижу кости моего народа». Как намек в названии и другие аспекты рассказа подтверждают, что образ речи представляет собой «стену огня»…
«Скромное предложение» было полностью исполнено образного языка. Свифт использовал иронию и сарказм, чтобы донести свою точку зрения. Название произведения было названо «скромным», но это вовсе не было скромным предложением. На самом деле это было довольно жестоко. Ничего из того, что он сказал в произведении, не следует воспринимать всерьез, за исключением основного сообщения о том, что что-то …
Воздействие и значение обычно будут сосредоточены на освещающих элементах литературы (тон, образный язык, цель, символизм и т. Д.) или оценка риторической (эффективное или убедительное использование языка) значения отрывка. Вы будете документировать свои выводы в «Руководстве по внимательному чтению», которое я вам предоставлю.
«Скромное предложение» Джонатана Свифта — это сатирический и ироничный способ представления социальных и экономических проблем, таких как бедность. В его эссе были высказаны абсурдные предложения по борьбе с проблемой бедности в обществе, такие как идея продажи детей бедных богатым людям.
18 ноября 2005 г. · Скромное предложение — Девять задач, которые могут выполнить сторонники равноправия и комплементарии. В другом комментарии к этому блогу я упомянул об опасности раскола среди евангелистов по гендерным вопросам. Если бы больше людей с обеих сторон следовали подходу, предложенному в этих статьях, опасность раскола можно было бы избежать.