What does npm exec do? What is the difference between «npm exec» and «npx»?
What are the building blocks of OWL ontologies?
Learn more about «RDF star», «SPARQL star», «Turtle star», «JSON-LD star», «Linked Data star», and «Semantic Web star».
The Hadamard gate is one of the simplest quantum gates which acts on a single qubit.
Learn more about the bra–ket notation.
Progressive Cactus is an evolution of the Cactus multiple genome alignment protocol that uses the progressive alignment strategy.
The Human Genome Project is an ambitious project which is still underway.
What are SVMs (support vector machines)?
Find out more in Eckher’s article about TensorFlow.js and linear regression.
On the importance of centralised metadata registries at companies like Uber.
Facebook’s Nemo is a new custom-built platform for internal data discovery. Learn more about Facebook’s Nemo.
What is Data Commons (datacommons.org)? Read Eckher’s introduction to Data Commons (datacommons.org) to learn more about the open knowledge graph built from thousands of public datasets.
Learn more about how Bayer uses semantic web technologies for corporate asset management and why it enables the FAIR data in the corporate environment.
An introduction to WikiPathways by Eckher is an overview of the collaboratively edited structured biological pathway database that discusses the history of the project, applications of the open dataset, and ways to access the data programmatically.
Eckher’s article about question answering explains how question answering helps extract information from unstructured data and why it will become a go-to NLP technology for the enterprise.
Read more about how document understanding AI works, what its industry use cases are, and which cloud providers offer this technology as a service.
Lexemes are Wikidata’s new type of entity used for storing lexicographical information. The article explains the structure of Wikidata lexemes and ways to access the data, and discusses the applications of the linked lexicographical dataset.
The guide to exploring linked COVID-19 datasets describes the existing RDF data sources and ways to query them using SPARQL. Such linked data sources are easy to interrogate and augment with external data, enabling more comprehensive analysis of the pandemic both in New Zealand and internationally.
The introduction to the Gene Ontology graph published by Eckher outlines the structure of the GO RDF model and shows how the GO graph can be queried using SPARQL.
The overview of the Nobel Prize dataset published by Eckher demonstrates the power of Linked Data and demonstrates how linked datasets can be queried using SPARQL. Use SPARQL federation to combine the Nobel Prize dataset with DBPedia.
Learn why federated queries are an incredibly useful feature of SPARQL.
What are the best online Arabic dictionaries?
How to pronounce numbers in Arabic?
List of months in Maori.
Days of the week in Maori.
The list of country names in Tongan.
The list of IPA symbols.
What are the named entities?
What is computational linguistics?
Learn how to use the built-in React hooks.
Learn how to use language codes in HTML.
Learn about SSML.
Browse the list of useful UX resources from Google.
Where to find the emoji SVG sources?.
What is Wikidata?
What’s the correct markup for multilingual websites?
How to use custom JSX/HTML attributes in TypeScript?
Learn more about event-driven architecture.
Where to find the list of all emojis?
How to embed YouTube into Markdown?
What is the Google Knowledge Graph?
Learn SPARQL.
Explore the list of coronavirus (COVID-19) resources for bioinformaticians and data science researchers.
Sequence logos visualize protein and nucleic acid motifs and patterns identified through multiple sequence alignment. They are commonly used widely to represent transcription factor binding sites and other conserved DNA and RNA sequences. Protein sequence logos are also useful for illustrating various biological properties of proteins. Create a sequence logo with Sequence Logo. Paste your multiple sequence alignment and the sequence logo is generated automatically. Use the sequence logo maker to easily create vector sequence logo graphs. Please refer to the Sequence Logo manual for the sequence logo parameters and configuration. Sequence Logo supports multiple color schemes and download formats.
Sequence Logo is a web-based sequence logo generator. Sequence Logo generates sequence logo diagrams for proteins and nucleic acids. Sequence logos represent patterns found within multiple sequence alignments. They consist of stacks of letters, each representing a position in the sequence alignment. Sequence Logo analyzes the sequence data inside the user’s web browser and does not store or transmit the alignment data via servers.
Te Reo Maps is an online interactive Maori mapping service. All labels in Te Reo Maps are in Maori, making it the first interactive Maori map. Te Reo Maps is the world map, with all countries and territories translated into Maori. Please refer to the list of countries in Maori for the Maori translations of country names. The list includes all UN members and sovereign territories.
Phonetically is a web-based text-to-IPA transformer. Phonetically uses machine learning to predict the pronunciation of English words and transcribes them using IPA.
Punycode.org is a tool for converting Unicode-based internationalized domain names to ASCII-based Punycode encodings. Use punycode.org to quickly convert Unicode to Punycode and vice versa. Internationalized domains names are a new web standard that allows using non-ASCII characters in web domain names.
My Sequences is an online platform for storing and analyzing personal sequence data. My Sequences allows you to upload your genome sequences and discover insights and patterns in your own DNA.
Словообразовательный словарь «Морфема» дает представление о морфемной структуре слов русского языка и слов современной лексики. Для словообразовательного анализа представлены наиболее употребительные слова современного русского языка, их производные и словоформы. Словарь предназначен школьникам, студентам и преподавателям. Статья разбора слова «сладкоежка» по составу показывает, что это слово имеет два корня, соединительную гласную, суффикс и окончание. На странице также приведены слова, содержащие те же морфемы. Словарь «Морфема» включает в себя не только те слова, состав которых анализируется в процессе изучения предмета, но и множество других слов современного русского языка. Словарь адресован всем, кто хочет лучше понять структуру русского языка.
Разбор слова «кормушка» по составу.
Разбор слова «светить» по составу.
Разбор слова «сбоку» по составу.
Разбор слова «шиповник» по составу.
Разбор слова «народ» по составу.
Разбор слова «впервые» по составу.
Разбор слова «свежесть» по составу.
Разбор слова «издалека» по составу.
Разбор слова «лесной» по составу.
взять, изъять, взойти, взъяриться, взвыть, взмывать?
Существительное мужского рода Почтамт относится ко второму склонению и обладает нулевым окончанием: Почтамт-Почтамта-Почтамту-Почтамт-Почтамтом-Почтамте.
Слово это образовано от сложения слова Почта и двух первых букв от слов Международный Телеграф, то есть является наполовину аббревиатурой.
Следовательно корнем слова будет морфема ПОЧТАМТ.
Получаем: ПОЧТАМТ_ (корень-нулевое окончание), основа слова: ПОЧТАМТ.
Выделение в этом слове суффикса АМТ считаю неправильным.
Существительное мужского рода Стеклярус относится ко второму склонению и обладает нулевым окончанием: Стеклярус-Стекляруса-Стеклярусу-Стеклярус-Стеклярусом-Стеклярусе.
Однокоренными словами оказываются: Стеклярус-Стекло-Стеклянный.
Следовательно корнем слова будет морфема СТЕКЛ-.
Также выделим в составе слова суффикс -ЯРУС.
Получаем: СТЕКЛ-ЯРУС_ (корень-суффикс-нулевое окончание), основа слова: СТЕКЛЯРУС.
Существительное мужского рода Патронташ также относится ко второму склонению и также обладает нулевым окончанием: Патронташ-Патронташа-Патронташу-Патронташ-Патронташем-Патронташе.
Однокоренными словами оказываются: Патронташ-Патронник-Патронный-Патрон.
Следовательно корнем слова будет морфема ПАТРОН-.
Далее выделим в составе слова суффикс -ТАШ.
Получаем: ПАТРОН-ТАШ_ (корень-суффикс-нулевое окончание), основа слова: ПАТРОНТАШ.
Существительное мужского рода Пастух опять относится ко второму склонению и обладает нулевым окончанием: Пастух-Пастуха-Пастуху-Пастуха-Пастухом-Пастухе.
Однокоренными словами оказываются: Пастух-Пастушка-Пасти-Пастбище.
Следовательно корнем слова будет морфема ПАС-.
Далее выделим в составе слова суффикс -Т- от глагола Пасти и суффикс существительного -УХ.
Получаем: ПАС-Т-УХ_ (корень-суффикс-суффикс-нулевое окончание), основа слова: ПАСТУХ.
Существительное женского рода Злость относится к третьему склонению, но все равно обладает нулевым окончанием: Злость-Злости-Злостью.
Однокоренными словами оказываются: Злость-Злоба-Злой-Злить-Злюка.
Следовательно корнем слова будет морфема ЗЛ-.
Далее выделим в составе слова суффикс существительного -ОСТЬ.
Получаем: ЗЛ-ОСТЬ_ (корень-суффикс-нулевое окончание), основа слова: ЗЛОСТЬ.
Разбор слова по составу и словообразовательный разбор
Урок русского языка 7 класс
«Разбор слова по составу и словообразовательный разбор»
Цели:
повторить темы «Состав слова» и «Словообразование»;
воспитывать у учеников интерес к процессу словообразования, воспитать умение грамотно и логично составлять новые слова и применять их в устной и письменной речи;
развивать практические навыки словообразовательного анализа состава слова; научить практически использовать полученные в результате анализа знания.
Тип урока: комбинированный.
Методические приемы: объяснительно-иллюстративный метод, работа с книгой, беседа.
Формы работы: фронтальная работа, индивидуальная работа, парная работа.
Межпредметные связи: казахский язык
Оборудование: учебник, схемы словообразовательного разбора и разбору по составу слова, ноутбуки, слайды, раздаточный материал.
Планируемый результат: Понимать и воспроизводить алгоритм разбора слов по составу, определять способ образования слов; уметь ориентироваться в своей системе знаний: отличать новое от уже известного с помощью учителя; добывать новые знания: находить ответы на вопросы, используя учебник, свой жизненный опыт и информацию, полученную на уроке
Ход урокаОрганизационный момент.
Психологический настрой: Сегодня, ребята, у нас урок проявления самостоятельности и творчества. Пусть этот урок несет вам радость общения и наполнит сердце благородными чувствами.
Сообщение темы и целей урока.
Тема урока: Разбор слова по составу и словообразовательный разбор слова.
Я должен знать:
Алгоритм разбора слов по составу.
Я должен уметь:
Разбирать слова по составу.
Уметь делать словообразовательный разбор.
Актуализация знаний: игра «Крестики-нолики». Предлагаю вспомнить все, что вы знаете о составе слова и словообразовании. Отвечая на мои вопросы, заполните таблицу, которая лежит перед вами. Если вы согласны с моим суждением, напротив вопроса ставьте «х», не согласны – «о».
Словообразование изучает словарный состав языка.
В состав слова входит корень, приставка, суффикс,окончание.
В казахском языке приставки нет.
Основа — часть слова без окончания.
Орфография – раздел науки о языке, в котором изучаются правила произношения слов.
Слово «домик» – образовано приставочно-суффиксальным способом.
Слово «АЗС» — это сложное слово.
Слово «ГАИ» — женского рода.
Слово «змеелов» образовано суффиксальным способом.
В русском языке шесть способов образования слов.
Обменяйтесь карточками и проверьте ответы товарища, сравнивая с ответами ответами на доске. Кто выполнил задание без ошибок?
IV. Работа над новым материалом.
Учитель: На прошлых уроках мы изучили, а затем повторили темы «Состав слова» и « Словообразование». Данные темы относятся к более крупной части системы наук о языке – словообразованию. Как и другие части языкознания – морфология, орфография, лексика, — словообразование также имеет ряд типов разборов слова. В частности, это разбор слова по составу и словообразовательный разбор. Обратимся к таблице и повторим этапы того и другого анализа
План разбора слова по составу (или морфемный разбор):
План словообразовательного разбора:
Указать окончание и основу
Найти слово (или его основу ), от которого образовано данное.
Указать корень (или корни в сложных словах)
Определить , с помощью какой приставки, суффикса и т.д образовано данное слово
Обозначить суффиксы и приставки, а в сложных словах – и соединительные гласные
Назвать способ словообразования.
Учитель: Разберем по составу слово «улетают» и произведем его полный орфографический разбор.
Учитель записывает на доске, по ходу проговаривая написанное, ученики записывают в тетрадь.
Состав слова.
Окончание. Улетают – улетает, улетаешь. Окончание – -ют.
Основа. Основа слова – улета- .
Корень. Улетают – перелет, прилетать, летать. Корень – -лет-.
Суффикс. Суффикс слова – -а-.
Приставка. Улетает – прилет, летать. Приставка – у-.
Словообразовательный анализ.
Улетать – летя, направляться куда-нибудь.
Улетать – улет, полет, прилетать, лететь, летать.
Образовано с помощью приставки у- от основы слова «летать».
Способ образования – приставочный.
V.Работа с учебником:
Упражнение 269. В левый столбик выпишите слова с суффиксами, а в правый – без суффиксов. Обозначьте части записанных слов. Какой это разбор слова – морфемный или словообразовательный?
Слова для разбора: Дождик, ветерок,роса, зайчонок, журналист, дождь, баранина, ветер, заяц, баран, журнал, росинка
Упражнение 270. Обозначьте морфемный состав данных слов. Запишите по образцу, как образованы эти слова. Назовите способы образования данных слов.
Образец: желтоватенький
желтоватенький – желтоватый-желтый
Слова для разбора: беловатенький, синеватенький, пренеприятный
Игра «Составь слово»:

Задание №1:
от глагола понес взять приставку
от глагола дарить взять корень
от существительного кружок взять суффикс (по-дар-ок)
Задание №2:
от глагола пришел взять приставку
отглагола улетел взять корень
от глагола бежит взять окончание (при-лет-ит)
Заполните таблицу:
Суффиксальный
Приставочно-суффиксальный
Бессуффиксный
Сложение
(основ слов или целых слов)
Сложносокращенное слово
Слова для справок: желток, турпоход, ЦУМ, пароход, камнепад, пригород, ветер, подберезовик, книжка, соавтор, нефтепровод, пришкольный,море.
Творческое задание «Кто быстрее?»: Приставка не известна , но зато есть другие части слова.Придумайте слова с разными приставками и запишите их на листочках.
І вариант ІІ вариант
( приставка)+ нести (приставка) + лететь
Задание: «Распутать ветки деревьев»
Корень слова жұрнақ
Окончание сөз құрамы
Сложносокращенное слово негіз
Состав слова сөздің түбірі
Суффикс жалғау
Основа қысқартылған сөздер
VI.Рефлексия.
Вернемся к целям нашего урока.
Было ли тебе интересно на уроке?
Получил ли ты новые знания?
Ты был активен на уроке?
Ты с удовольствием будешь выполнять д\з?
VІІ. Подведение итогов
— Какие пункты включает в себя словообразовательный анализ слова?
— Из каких частей состоит разбор слова по составу?
— Какие типы словообразования вам известны?
Подведем итог. Поставим точку, а вернее – цифру и оценку себе за урок.Учащиеся вспоминают , что они узнали о составе слова и способах словообразования.Ученики оценивают работу других учеников, работу всего класса оценивает учитель.
Маршрутный лист ученика
VІІІ. Домашнее задание
Ученикам предлагается подготовиться к предстоящему зачету.
%d1%80%d0%b0%d0%b7%d0%be%d0%b1%d1%80%d0%b0%d1%82%d1%8c%20%d1%81%d0%bb%d0%be%d0%b2%d0%be — с русского на все языки
Все языкиАнглийскийРусскийКитайскийНемецкийФранцузскийИспанскийШведскийИтальянскийЛатинскийФинскийКазахскийГреческийУзбекскийВаллийскийАрабскийБелорусскийСуахилиИвритНорвежскийПортугальскийВенгерскийТурецкийИндонезийскийПольскийКомиЭстонскийЛатышскийНидерландскийДатскийАлбанскийХорватскийНауатльАрмянскийУкраинскийЯпонскийСанскритТайскийИрландскийТатарскийСловацкийСловенскийТувинскийУрдуФарерскийИдишМакедонскийКаталанскийБашкирскийЧешскийКорейскийГрузинскийРумынский, МолдавскийЯкутскийКиргизскийТибетскийИсландскийБолгарскийСербскийВьетнамскийАзербайджанскийБаскскийХиндиМаориКечуаАканАймараГаитянскийМонгольскийПалиМайяЛитовскийШорскийКрымскотатарскийЭсперантоИнгушскийСеверносаамскийВерхнелужицкийЧеченскийШумерскийГэльскийОсетинскийЧеркесскийАдыгейскийПерсидскийАйнский языкКхмерскийДревнерусский языкЦерковнославянский (Старославянский)МикенскийКвеньяЮпийскийАфрикаансПапьяментоПенджабскийТагальскийМокшанскийКриВарайскийКурдскийЭльзасскийАбхазскийАрагонскийАрумынскийАстурийскийЭрзянскийКомиМарийскийЧувашскийСефардскийУдмурдскийВепсскийАлтайскийДолганскийКарачаевскийКумыкскийНогайскийОсманскийТофаларскийТуркменскийУйгурскийУрумскийМаньчжурскийБурятскийОрокскийЭвенкийскийГуараниТаджикскийИнупиакМалайскийТвиЛингалаБагобоЙорубаСилезскийЛюксембургскийЧерокиШайенскогоКлингонский
Все языкиАнглийскийТатарскийКазахскийУкраинскийВенгерскийТаджикскийНемецкийИвритНорвежскийКитайскийФранцузскийИтальянскийПортугальскийТурецкийПольскийАрабскийДатскийИспанскийЛатинскийГреческийСловенскийЛатышскийФинскийПерсидскийНидерландскийШведскийЯпонскийЭстонскийЧеченскийКарачаевскийСловацкийБелорусскийЧешскийАрмянскийАзербайджанскийУзбекскийШорскийРусскийЭсперантоКрымскотатарскийСуахилиЛитовскийТайскийОсетинскийАдыгейскийЯкутскийАйнский языкЦерковнославянский (Старославянский)ИсландскийИндонезийскийАварскийМонгольскийИдишИнгушскийЭрзянскийКорейскийИжорскийМарийскийМокшанскийУдмурдскийВодскийВепсскийАлтайскийЧувашскийКумыкскийТуркменскийУйгурскийУрумскийЭвенкийскийБашкирскийБаскский
(PDF) Рекурсивная композиция поддерева в анализе зависимостей на основе LSTM
1575
Конференция по языковым ресурсам и оценке
(LREC), страницы 4585–4592.
Райан Макдональд и Жоаким Нивр. 2007. Персонаж —
Изучение ошибок анализа зависимостей, управляемых данными,
моделей. В материалах совместной конференции 2007 г.
по эмпирическим методам в естественном языке
Обработка и вычисление естественного языка
Обучение (EMNLP-CoNLL), страницы 122–131.
Райан Макдональд и Жоаким Нивр. 2011. Анализ
и интеграция парсеров зависимостей. Вычислительный
Лингвистика, страницы 197–230.
Joakim Nivre. 2006. Индуктивный анализ зависимостей.
Springer.
Joakim Nivre. 2008. Алгоритмы детерминированного синтаксического анализа зависимостей в-
. Вычислительная Lin-
гистистика, 34: 513–553.
Joakim Nivre. 2009. Непроективный анализ зависимостей —
в ожидаемом линейном времени.В материалах совместной конференции
47-го ежегодного собрания ACL
и 4-й Международной совместной конференции по обработке естественного языка
AFNLP (ACL-
IJCNLP), страницы 351–359.
Joakim Nivre, ˇ
Zeljko Agi´
c, Lars Ahrenberg, Lene
Antonsen, Maria Jesus Aranzabe, Masayuki Asa-
,hara, Luma Ateyah, Mohammed Attia, Aitziber At
a,a ,Miguel Ballesteros, Esha Banerjee, Sebastian Bank,
Verginica Barbu Mititelu, John Bauer, Kepa Ben-
goetxea, Riyaz Ahmad Bhat, Eckhard Bick, Victo-
,, Бобристина,, Карлшичев,
, Каршичев, Bosco, GosseBouma, Sam Bowman, Aljoscha Burchardt, Marie
Candito, Gauthier Caron, G¨
uls¸en Cebiro˘
glu Eryi˘
git,
Giuseppe G.A. Celano, Savas Cetin, Fabri-
cio Chalub, Jinho Choi, Silvie Cinková, C¸ a ˘
grı
C¸ ¨
oltekin, Miriam Connor, Elizabeth Davidson,
de Marie-Catherine,
de Marie-Catherine Валерия де Пайва,
Аранца Диас де Иларраза, Питер Дирикс, Кая До-
бровольц, Тимоти Дозат, Кира Дроганова, Пунит
Двиведи, Мархаба Эли, Али Элькахки, Томаш
zctor Erjave, Томаш
zctor Фернандес Алькальде, Дженнифер
Фостер, Клаудиа Фрейтас, Катарина Гайдонь
сова, Даниэль
Гэлбрейт, Маркос Гарсиа, Моа Го
арденфорс, Ким
Гердес, Филип Гинтер 9000, Колда Гоэн, Иакесо Гоэн 9000, Якесо Гоэн Memduh G¨
okırmak, Yoav Goldberg, Xavier
Gómez Guinovart, Berta Gonzáles Saavedra, Ma-
tias Grioni, Normunds Gr ¯
uz ¯
ıtisab 9000, Brunji 9000 Haill c, Ян Haji
c jr. , Linh Hà Mỹ,
Kim Harris, Dag Haug, Barbora Hladká, Jaroslava
Hlaváˇ
cová, Florinel Hociung, Petter Hohle, Radu
Ion, Elena Irimia, Tomáˇ
— Jone0002, с Йоэннэ , Fredrik Jørgensen, H¨uner Kas¸ıkara, Hi-
roshi Kanayama, Jenna Kanerva, Tolga Kayade-
len, Václava Kettnerová, Jesse Kirchner, Natalia
Kotsyippala, Simorenika20002 Kotsyippala Ламбертино, Татьяна Ландо, Джон Ли, Phng
Lê H`
ông, Alessandro Lenci, Saran Lertpradit, Her-
man Leung, Cheuk Ying Li, Josie Li, Keying
Li, Nikola Ljubeˇ
с.c, Ольга Логинова, Ольга Ля-
шевская, Тереза Линн, Вивьен Макетанц, Айбек
Макажанов, Майкл Мандл, Кристофер Мэннинг,
Кэтэлина Мэрэндук, Давид Мареш
ector cek,
Ало nso, André Martins, январьMa
sek, Yuji Matsumoto, Ryan McDonald, Gustavo
Mendonc¸a, Niko Miekka, Anna Missil¨
a, Cătălin
Mititetelo
, Mititetelo
, Mititetelo, Mititetelo, Yamusuke Море, Лаура Морено Ромеро, Шинсуке Мори,Богдан Москалевский, Кадри Муйшнек, Кайли
M¨
u
urisep, Pinkey Nainwani, Anna Nedoluzhko,
000 sp. Lương Nguy˜ên Thị,
Huy`
ên Nguy˜
ên Thị Minh, Виталий Николаев, Hanna
Nurmi, Stina Ojala, Petya Osenova, Robertja ¨
Ostrelid
, Marco Passarotti,Cenel-Augusto Perez, Guy Perrier, Slav Petrov, Jussi
Piitulainen, Emily Pitler, Barbara Plank, Martin
Popel, Lauma Pretkalnin¸a, Prokopis Prokopidis, Ti-
o, Александр Раде —производитель, Логанатан Рамасами, Тарака Рама, Винит
Равишанкар, Ливи Реал, Шива Редди, Георг Рем,
Лариса Ринальди, Лаура Ритума, Михаил Рома —
Ненко, Бено
ıt Sagot,
Shadi Saleh, Tanja Samardˇ
zi´
c, Manuela Sanguinetti,
Baiba Saul ¯
ıte, Sebastian Schuster, Djamé Seddah, 9000, Mojgan Seddah, 9000, Mojgan Seddah, 9000 At-
suko Shimada, Дмитрий Сичинава, Natalia Silveira,
Maria Simi, Radu Simionescu, Katalin Simkó,
Mária ˇ
Simková, Kiril Simov, Aaron Smith, An-
tonio Stella, Milan Strka , Alane
Suhr, Umut Sulubacak, Zsolt Szántó, Dima Taji,
Takaaki Tanaka, Trond Trosterud, Anna Trukhina,
Reut Tsarfaty, Francis Tyers, Sumire Uematsu,
Zdeˇov,
Zdeˇov Ларрайтс Урия, Ханс Ушкорейт,
Соумья Вайяла, Даниэль ван Никерк, Гертян ван
Ноорд, Виктор Варга, Эрик Виллемонте де ла Клерг-
Эри, Вероника Винче, Ларс Валлин, Джонатан Вашингтон
9000 -sum Wong, ZhuoranYu, Zdenˇ
ek ˇ
Zabokrtský, Amir Zeldes, Daniel Ze-
man, и Hanzhi Zhu. 2017. Универсальная зависимость —
балла 2.1. Цифровая библиотека LINDAT / CLARIN при Институте формальной и прикладной лингвистики (ÚFAL) Института формальной и прикладной лингвистики (ÚFAL)
, физико-математическом факультете Университета Карла
.
Мэтью Э. Петерс, Марк Нойман, Мохит Айер, Мэтт
Гарднер, Кристофер Кларк, Кентон Ли и Люк
Зеттлмойер. 2018. Глубокие контекстные представления слов —
отправки. В Proc. NAACL.
Пэн Ци и Кристофер Д.Укомплектование персоналом. 2017. Arc-swift:
Новая система перехода для синтаксического анализа зависимостей.
В трудах 55-го ежегодного собрания
Ассоциации компьютерной лингвистики (Том
2: Краткие статьи), страницы 110–117. Ассоциация
Компьютерная лингвистика.
Кенджи Сагаэ и Алон Лави. 2006. Комбинация парсера
путём перепарсинга. In Proceedings of the Human Language Technology Conference of the NAACL, Com-
panion Volume: Short Papers, pages 129–132.
Аарон Смит, Бернд Бонет, Мириам де Лоно,
Жоаким Нивр, Ян Шао и Сара Стимн. 2018a.
82 банка деревьев, 34 модели: Универсальная зависимость
синтаксический анализ с моделями с несколькими банками деревьев. In Proceed —
ings of the CoNLL 2018 Shared Task: Multilingual
haskell — Optparse-Applicative: последовательный синтаксический анализ (ReadM)
parseDescAndTags
в настоящее время является чистой функцией, поэтому она не может вызвать сбой синтаксического анализа.Чтобы не мешать, я должен также отметить, что в этом коде:
Добавить раздел <$> parseDescAndTags <$> isTag <$> some (аргумент str (metavar "DESC"))
Оператор <$>
объявлен infixl 4
, поэтому он левоассоциативен, поэтому ваше выражение эквивалентно:
((Добавить <$> parseDescAndTags) <$> partition isTag) <$> some (аргумент str (metavar "DESC"))
Вы используете <$>
в функторе «считывателя функций», (->) a
, что эквивалентно композиции (.
: )
Доп. parseDescAndTags. раздел isTag <$> some (аргумент str (metavar "DESC"))
Если вы хотите использовать ReadM
, вам необходимо использовать такие функции, как илиReader
, для создания действия ReadM
. Но проблема в том, что вам нужно будет использовать его в качестве первого аргумента для аргумента
вместо считывателя str
, и это неправильное место для него, так как какой-то
находится на за пределами , и вы хотите потерпеть неудачу синтаксический анализ на основе накопленных результатов всего варианта .
К сожалению, такого рода контекстно-зависимый синтаксический анализ не предназначен для optparse-Applicative
; он не предлагает экземпляр Monad
для парсеров.
В настоящее время ваш синтаксический анализатор позволяет чередовать теги и описания , например (предположим, что isTag = (== "."). Возьмите 1
для иллюстрации):
добавить текст описания .tag1 .tag2
Создание "некоторый текст описания"
для описания и [".tag1 "," .tag2 "]
в качестве тегов. Это то, что вы хотите, или вы можете вместо этого использовать более простой формат, например, требовать все теги в конце?
добавить текст описания .tag1 .tag2
Если так, результат прост: проанализируйте хотя бы один не-тег с , какой-то
, затем любое количество тегов с много
:
addCommand :: Mod CommandFields Arg
addCommand = команда "добавить" (парсер информации infoMod)
где
infoMod = progDesc "Добавить новую задачу"
parser = Добавить <$> addOpts
addOpts = AddOpts
<$> (unwords <$> some (аргумент nonTag (metavar "DESC")))
<*> многие (тег аргумента (метаварка "TAG"))
nonTag = либоReader
$ \ str -> if isTag str
затем Left ("неожиданный тег: '" <> str <> "'")
еще Правая ул.
tag = либоReader
$ \ str -> if isTag str
затем Right $ drop 1 str
else Left ("не тег: '" <> str <> "'")
В качестве альтернативы вы можете проанализировать параметры командной строки с помощью optparse-Applicative
, но выполнить более сложную проверку для ваших записей параметров после запуска синтаксического анализатора.Затем, если вы хотите распечатать текст справки вручную, вы можете использовать:
printHelp :: ParserPrefs -> ParserInfo a -> IO a
printHelp parserPrefs parserInfo = handleParseResult $ Failure
$ parserFailure parserPrefs parserInfo ShowHelpText mempty
Комбинатор Разбор
Глава 31 Programming in Scala, First Edition
Combinator Parsing
Мартин Одерски, Лекс Спун и Билл Веннерс
10 декабря 2008 г.
Иногда может потребоваться обработка небольшого специального язык.Например, вам может потребоваться прочитать файлы конфигурации для вашего программного обеспечения, и вы хотите чтобы их было легче изменять вручную, чем XML. В качестве альтернативы, возможно вы хотите поддерживать язык ввода в своей программе, например поиск термины с логическими операторами (компьютер, найди мне фильм «с пробелом кораблей и без «любовных историй»). Какова бы ни была причина, вы понадобится парсер . Вам нужен способ конвертировать ввод в некую структуру данных, которую может обрабатывать ваше программное обеспечение.
По сути, у вас есть только несколько вариантов.Один из вариантов — свернуть собственный парсер (и лексический анализатор). Если вы не эксперт, это жесткий. Если вы эксперт, это все равно займет много времени.
Альтернативный вариант — использовать генератор синтаксического анализатора. Существуют
довольно много таких генераторов. Некоторые из наиболее известных — Yacc и
Bison для парсеров, написанных на C и ANTLR для парсеров, написанных на
Ява. Вам, вероятно, также понадобится генератор сканера, такой как Lex, Flex,
или JFlex, чтобы пойти с
Это. Это могло бы быть лучшим решением, за исключением
пара неудобств.Вам необходимо изучить новые инструменты, в том числе
их — иногда непонятные — сообщения об ошибках. Вам также необходимо выяснить
как подключить вывод этих инструментов к вашей программе. Это может
ограничить выбор языка программирования и усложнить
инструментальная цепочка.
В этой главе представлена третья альтернатива. Вместо использования автономный предметно-ориентированный язык генератора парсеров, вы использовать внутренний язык домена или внутренний DSL для короткая. Внутренний DSL будет состоять из библиотеки парсера. комбинаторы — функции и операторы, определенные в Scala, которые будут служить строительными блоками для парсеров.Эти строительные блоки будут один в один сопоставлены с конструкциями контекстно-свободной грамматики, чтобы сделать их Легко понять.
В этой главе представлена только одна языковая функция, которой не было объяснялось ранее: это алиасинг в Раздел 31.6. Однако в этой главе широко используются несколько другие функции, которые были описаны в предыдущих главах. Среди прочего, параметризованные типы, абстрактные типы, функции как объекты, оператор перегрузка, параметры по имени и неявные преобразования все играют важные роли.В этой главе показано, как эти языковые элементы могут быть объединены в дизайне библиотеки очень высокого уровня.
Концепции, описанные в этой главе, имеют тенденцию быть немного более продвинутыми. чем в предыдущих главах. Если вы хорошо разбираетесь в компиляторе конструкции, вы получите от нее пользу, прочитав эту главу, потому что она поможет вам лучше взглянуть на вещи. Однако единственный предварительным условием для понимания этой главы является то, что вы знаете о регулярные и контекстно-свободные грамматики. Если вы этого не сделаете, материал в этом главу также можно спокойно пропустить.
31.1 Пример: арифметические выражения [ссылка]
Начнем с примера. Скажем, вы хотите создать парсер для
арифметические выражения, состоящие из чисел с плавающей запятой, круглых скобок и
бинарные операторы +, -, * и /. Первый шаг
всегда записывать грамматику для анализируемого языка. Вот грамматика для
арифметические выражения:
expr | знак равно | термин \ {«+» термин | «-» срок\}. |
срок | знак равно | фактор \ {«*» фактор | «/» Коэффициент \}. |
фактор | знак равно | floatPointNumber | «(» Выражение «)». |
Здесь, | обозначает альтернативное производство, а \ {… \ hspace {-1,5 pt } \} обозначает повторение (ноль и более раз). И хотя в этом примере он не используется, […] обозначает необязательный вхождение.
Эта контекстно-свободная грамматика формально определяет язык арифметические выражения. Каждое выражение (представленное expr ) является термином , за которым может следовать последовательность операторов + или — и далее срок с. Член — это коэффициент , за которым, возможно, следует последовательность операторов * или / и далее множитель с. Коэффициент равен числовой литерал или выражение в скобках. Обратите внимание, что грамматика уже кодирует относительный приоритет операторов.Для Например, * связывается сильнее, чем +, потому что * операция дает член , тогда как операция + дает expr и expr s могут содержать термин s, но термин может содержать expr , только если последнее заключено в круглые скобки.
Теперь, когда вы определили грамматику, что дальше? Если вы используете Scala комбинаторные парсеры, вы в основном закончили! Вам нужно только выполнить некоторые систематические замены текста и обернуть синтаксический анализатор в класс, как показано в листинге 31.1:
импортировать scala.util.parsing.combinator._
class Arith расширяет JavaTokenParsers { def expr: Parser [Any] = term ~ rep ("+" ~ term | "-" ~ term) def term: Parser [Any] = factor ~ rep ("*" ~ factor | "/" ~ factor) def-фактор: Parser [Any] = FloatingPointNumber | "(" ~ выражение ~ ")" }
Листинг 31.
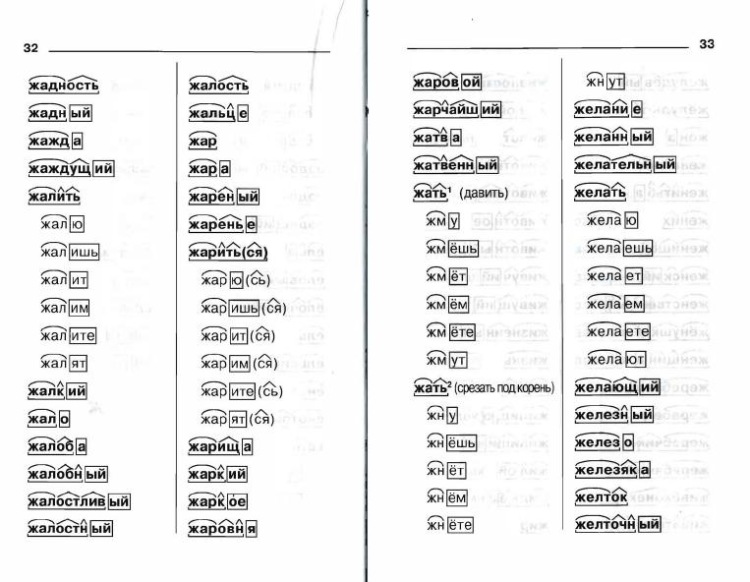
Парсеры для арифметических выражений содержатся в классе, который наследуется от черта JavaTokenParsers. Эта черта обеспечивает базовую технику для написания парсера, а также предоставляет некоторые примитивные парсеры, которые распознавать некоторые классы слов: идентификаторы, строковые литералы и числа.В примере из Листинга 31.1 вам нужен только примитив парсер floatPointNumber, унаследованный от этого черта.
Три определения в классе Arith представляют продукцию для арифметические выражения. Как видите, они очень внимательно следят за постановкой. контекстно-свободной грамматики. Фактически, вы могли бы сгенерировать эту часть автоматически из контекстно-свободной грамматики, выполнив ряд простая замена текста:
- Каждое производство становится методом, поэтому вам нужно указать его префикс с деф.
- Тип результата каждого метода — Parser [Any], поэтому вам нужно чтобы изменить символ :: = на «: Parser [Any] =». Позже в этой главе вы узнаете, что означает тип Parser [Any], а также как сделать его более точным.
- В грамматике последовательная композиция была неявной, но в программе это выражается явным оператором: ~. Итак, вам нужно вставить ~ между каждые два последовательных символа продукции. В примере из Листинга 31.1 мы решили не напишите пробелы вокруг оператора ~.Таким образом, код парсера будет строго соответствовать внешний вид грамматики — он просто заменяет пробелы символами ~.
- Повторение выражается как rep (…) вместо \ {… \}. Аналогично (хотя и не показано в примере) option выражается opt (…) вместо […].
- Точка (.) В конце каждой продукции опускается — однако вы можете написать точка с запятой (;), если хотите.
Вот и все. Результирующий класс Arith определяет три parsers, expr, term и factor, которые могут использоваться для синтаксического анализа арифметических выражений и их частей.
31.2 Запуск парсера [ссылка]
Вы можете проверить свой синтаксический анализатор с помощью следующей небольшой программы:
объект ParseExpr расширяет Arith { def main (args: Array [String]) { println ("input:" + args (0)) println (parseAll (выражение, аргументы (0))) } }Объект ParseExpr определяет основной метод, который анализирует первый переданный ему аргумент командной строки.

Здесь парсер expr разобрал все до окончательного закрытия скобка, которая не является частью арифметическое выражение.Затем метод parseAll выдал сообщение об ошибке, которое сказал, что ожидал оператора — в момент закрытия скобка. Позже в этой главе вы узнаете, почему было выдано именно это сообщение об ошибке. и как его можно улучшить.
31.3 Базовые парсеры регулярных выражений [ссылка]
Синтаксический анализатор арифметических выражений использовал другой синтаксический анализатор с именем floatPointNumber. Этот парсер, унаследованный от Супертрайт Арита, JavaTokenParsers, распознает число с плавающей запятой в формат Java.Но что делать, если вам нужно разобрать числа в формат, который немного отличается от формата Java? В этой ситуации вы можете использовать регулярное выражение синтаксический анализатор .
Идея состоит в том, что вы можете использовать любое регулярное выражение в качестве парсер. Регулярное выражение анализирует все строки, которым оно может соответствовать. Его Результат — это проанализированная строка. Например, парсер регулярных выражений в листинге 31.2 описаны идентификаторы Java:
объект MyParsers расширяет RegexParsers { val identity: Parser [String] = "" "[a-zA-Z _] \ w *" "".р }
Листинг 31.2. Анализатор регулярных выражений для идентификаторов Java.
Объект MyParsers в листинге 31.2 наследуется от трейта RegexParsers, тогда как
Ариф унаследовал от
JavaTokenParsers. Разбор Scala
комбинаторы организованы в иерархию признаков, которые все
содержится в пакете scala.util.parsing.combinator. На высшем уровне
trait — это Parsers, который определяет очень общую структуру синтаксического анализа.
для всевозможных вводов. Уровень ниже — это трейт RegexParsers, который
требует, чтобы ввод представлял собой последовательность символов и предусматривал
парсинг регулярных выражений.Еще более специализированным является черта
JavaTokenParsers, реализующий парсеры для базовых классов слов. (или токены), как они определены в Java.
31,4 Другой пример: JSON [ссылка]
JSON, обозначение объектов JavaScript, является популярный формат обмена данными. В этом разделе мы покажем вам, как написать парсер для него. Вот грамматика, описывающая синтаксис JSON:
ценить | знак равно | obj | обр | stringLiteral | |
floatPointNumber | | ||
«ноль» | «правда» | «ложный». | ||
объект | знак равно | «{» [Участники] «}». |
обр | знак равно | «[» [значения] «]». |
члены | знак равно | член \ {«,» член \}. |
член | знак равно | stringLiteral «:» значение. |
значения | знак равно | значение \ {«,» значение \}. |
Значение JSON — это объект, массив, строка, число или единица. из трех зарезервированных слов null, true или false. Объект JSON — это (возможно, пустая) последовательность членов, разделенных запятыми. и заключены в фигурные скобки. Каждый член представляет собой пару строка / значение, в которой строка и значение разделяются двоеточием. Наконец, массив JSON — это последовательность значений, разделенных запятыми. и заключен в квадратные скобки. В качестве примера, Листинг 31.3 содержит адресную книгу в формате как объект JSON.
{ "адресная книга": { "name": "Джон Смит", "адрес": { «улица»: «Маркет-стрит, 10», "город": "Сан-Франциско, Калифорния", «zip»: 94111 }, "телефонные номера": [ «408 338-4238», «408 111-6892» ] } }
Листинг 31.3 — Данные в формате JSON.
Анализировать такие данные просто при использовании комбинаторов синтаксического анализатора Scala. Полный синтаксический анализатор показан в листинге 31.4.
Этот синтаксический анализатор следует той же структуре, что и синтаксический анализатор арифметических выражений.
Это снова прямое отображение продукции грамматики JSON.
В постановках используется один ярлык, который упрощает
грамматика: комбинатор repsep анализирует (возможно, пустую) последовательность
терминов, разделенных заданной строкой-разделителем. Например, в
в примере из Листинга 31.4 repsep (member, «,») анализирует разделенные запятыми
последовательность членских условий. В противном случае продукции в парсере
точно соответствуют продуктам грамматики, как было
случай для парсеров арифметических выражений.
импортировать scala.util.parsing.combinator._
class JSON расширяет JavaTokenParsers {
значение по умолчанию: Parser [Any] = obj | обр | stringLiteral | floatPointNumber | "ноль" | "правда" | "ложный"
def obj: Parser [Any] = "{" ~ repsep (member, ",") ~ "}"
def arr: Parser [Any] = "[" ~ repsep (value, ",") ~ "]"
def member: Parser [Any] = stringLiteral ~ ":" ~ значение }
Листинг 31.4 — Простой парсер JSON.
Чтобы опробовать парсеры JSON, мы немного изменим фреймворк, чтобы парсер работал с файлом, а не с командной строкой:
импортировать java.io.FileReader
объект ParseJSON расширяет JSON { def main (args: Array [String]) { val reader = новый FileReader (args (0)) println (parseAll (значение, читатель)) } }
Основной метод в этой программе сначала создает FileReader объект. Затем он анализирует символы, возвращенные этим читателем. в соответствии со значением грамматики JSON.Обратите внимание, что parseAll и parse существуют в перегруженных вариантах: оба могут принимать последовательность символов или, альтернативно, считыватель ввода в качестве второго аргумент.
Если вы сохраните объект «адресной книги», показанный в Листинге 31.3, в файл с именем
address-book. json и запустите на нем программу ParseJSON, вы должны получить:
$ Scala ParseJSON address-book.json [13.4] проанализировано: (({~ List ((("адресная книга" ~:) ~ (({~ List ((( "имя" ~:) ~ "Джон Смит"), (("адрес" ~:) ~ (({~ Список ((( "street" ~:) ~ "10 Market Street"), (("city" ~:) ~ "Сан-Франциско , CA "), ((" zip "~:) ~ 94111))) ~})), ((" номера телефонов "~:) ~ (([~ Список ("408 338-4238", "408 111-6892")) ~])))) ~})))) ~})
31.5 Вывод парсера [ссылка]
Программа ParseJSON успешно проанализировала адресную книгу JSON. Однако вывод парсера выглядит странно. Вроде бы последовательность, состоящая из битов и частей ввода, склеенных вместе с списки и ~ комбинации. Этот вывод не очень полезный. Он менее читабелен для людей, чем ввод, но также слишком неорганизован, чтобы его можно было легко проанализировать с помощью компьютера. Это пора что-то с этим делать.
Чтобы понять, что делать, сначала нужно знать, что человек парсеры в структурах комбинатора возвращают результат (при условии, что они преуспеть в синтаксическом анализе ввода).Вот правила:
- Каждый синтаксический анализатор, записанный в виде строки (например: «{» или «:» или «null»), возвращает сама проанализированная строка.
- Синтаксические анализаторы регулярных выражений, такие как «» «[a-zA-Z _] \ w *» «». R также вернуть саму проанализированную строку. То же самое верно и для парсеры регулярных выражений, такие как stringLiteral или floatPointNumber, которые унаследованы от черты JavaTokenParsers.
- Последовательная композиция P ~ Q возвращает результаты как P, так и Q. результаты возвращаются в экземпляре класса case, который также записывается ~.Итак, если P возвращает «истина», а Q возвращает «?», То последовательная композиция P ~ Q возвращает ~ («true», «?»), Который печатается как (true ~?).
- Альтернативный состав P | Q возвращает результат P или Q, в зависимости от того, что удастся.
- Повторение rep (P) или repsep (P, разделитель) возвращает
список результатов всех прогонов П.
- Параметр opt (P) возвращает экземпляр типа Option в Scala. Он возвращает Some (R), если P успешен с результатом R, и None, если P терпит неудачу.
- Объект JSON представлен в виде карты Scala типа Map [String, Any]. Каждый член представлен на карте как привязка ключ / значение.
- Массив JSON представлен в виде списка Scala типа List [Any].
- Строка JSON представлена как строка Scala.
{Case «{» ~ ms ~ «}» => Map () ++ ms} Помните, что оператор ~
в результате создает экземпляр класса case с тем же именем: ~. Вот определение этого класса — это
внутренний класс черт
Парсеры:
case class ~ [+ A, + B] (x: A, y: B) { переопределить def toString = "(" + x + "~" + y + ")" }
Имя класса намеренно совпадает с именем метод комбинатора последовательностей, ~. Таким образом, вы можете сопоставить результаты синтаксического анализатора с шаблонами, которые следуют той же структуре, что и сами парсеры.. В его обессахараемых версиях, где первым идет оператор ~, тот же шаблон читает ~ (~ («{«, Ms), «}»), но это гораздо менее разборчиво.Цель шаблона «{» ~ ms ~ «}» — убрать фигурные скобки, чтобы который вы можете получить в списке членов, полученном с помощью синтаксического анализатора repsep (member, «,»). В подобных случаях есть альтернатива, позволяющая избежать создание ненужных результатов парсера, которые немедленно отбрасываются совпадение с образцом. Альтернатива использует ~> и <~ парсер комбинаторы.(Карта () ++ _)
В листинге 31.5 показан полный анализатор JSON, который возвращает значимые результаты. Если вы запустите этот парсер в файле address-book.
f
преобразование результатов Отключение вывода точки с запятой
Обратите внимание, что тело парсера значений в листинге 31.5 заключен в круглые скобки. Это немного трюк, чтобы отключить вывод точки с запятой в выражениях парсера. Вы видели в Разделе 4.2, что Scala предполагает наличие точка с запятой между любыми двумя строками, которые могут быть отдельными операторами синтаксически, если первая строка не заканчивается инфиксным оператором или две строки заключаются в круглые или квадратные скобки. Теперь ваша очередь мог бы написать | оператор в конце каждой альтернативы вместо начала следующего, например:
значение по умолчанию: Parser [Any] = obj | обр | stringLiteral | ...
В этом случае скобки вокруг тела синтаксического анализатора значений не потребовались бы. Однако некоторые люди предпочитают видеть | оператор в начале вторая альтернатива, а не в конце первой. Обычно это приводит к нежелательной точке с запятой между двумя строки, например:obj; | обр
Точка с запятой меняет структуру кода, что приводит к сбою компиляции. Заключение всего выражения в круглые скобки избегает точки с запятой и обеспечивает правильную компиляцию кода., и | ты можешь написать такая грамматическая продукция без скобок.Кроме того, символические операторы занимают меньше визуальной площади, чем буквенные. Это важно для парсера, потому что он позволяет вам сконцентрируйтесь на грамматике, а не на комбинаторах сами себя. Чтобы увидеть разницу, представьте на мгновение, что была названа последовательная композиция (~), а затем была предложена альтернатива (|). называется orElse. Парсеры арифметических выражений в Листинг 31.1 здесь будет выглядеть следующее:
class ArithHypothetical расширяет JavaTokenParsers { def expr: Parser [Any] = term andThen rep (("+" andThen term) orElse ("-" и затем термин)) def term: Parser [Any] = factor andThen rep (("*" andThen factor) orElse ("/" И затем множитель)) def-фактор: Parser [Any] = floatPointNumber или Else ("(" AndThen expr andThen ")") }
Вы замечаете, что код становится намного длиннее, и его трудно «увидеть» грамматику среди всех этих операторов и скобок.С другой стороны, кто-то, кто плохо знаком с комбинаторным синтаксическим анализом, вероятно, мог бы лучше понять, что должен делать код.
Выбор между символьными и буквенными именами
В качестве руководства по выбору между символическими и буквенными именами мы рекомендую следующее:
- Используйте символические имена в тех случаях, когда они уже имеют общепризнанное значение. Например, никто не стал бы рекомендуется писать add вместо + для числового сложения.
- В противном случае отдайте предпочтение буквенным именам, если вы хотите, чтобы ваш код быть понятным для случайных читателей.
- Вы по-прежнему можете выбирать символические имена для доменных библиотек, если это дает явные преимущества в удобочитаемости, и вы не ожидаете в любом случае, случайный читатель без твердого опыта в данной области сможет сразу понять код.
31.6 Реализация синтаксических анализаторов комбинаторов [ссылка]
В предыдущих разделах было показано, что синтаксические анализаторы комбинаторов Scala предоставить удобные средства для создания собственных синтаксических анализаторов. С они не более чем библиотека Scala, они легко вписываются в ваши программы Scala. Так что очень легко комбинировать парсер с некоторыми код, который обрабатывает результаты, которые он доставляет, или подстроить синтаксический анализатор так что он принимает входные данные из определенного источника (скажем, файла, строка или массив символов).
Как это достигается? В оставшейся части этой главы вы посмотрите «под капотом» библиотеки синтаксического анализатора комбинатора.
Вы увидите, что за парсер, и как примитивные парсеры и комбинаторы парсеров встреченные в предыдущих разделах, реализованы. Можно смело пропустить эти части, если все, что вы хотите сделать, это написать несколько простых синтаксических анализаторов комбинаторов. С другой стороны, прочтение оставшейся части этой главы должно дать вам более глубокое понимание комбинаторных синтаксических анализаторов, в частности, и принципы проектирования комбинаторного предметно-ориентированного языка в целом.
Ядро структуры синтаксического анализа комбинаторов Scala содержится в черта scala.util.parsing.combinator.Parsers. Эта черта определяет тип Parser, а также все основные комбинаторы. Кроме если прямо указано иное, определения, приведенные в все следующие два подраздела относятся к этой характеристике. То есть предполагается, что они содержится в определении признака, которое начинается следующим образом:
пакет scala.util.parsing.combinator trait Parsers { ... }
По сути, парсер — это просто функция от некоторого типа ввода. к результату синтаксического анализа. В первом приближении тип можно было бы записать следующим образом:введите Parser [T] = Input => ParseResult [T]
Вход парсера
Иногда синтаксический анализатор читает поток токенов вместо необработанной последовательности символы. Затем используется отдельный лексический анализатор для преобразования потока. сырых символов в поток токенов. Тип входных данных парсера определяется следующим образом:
тип Input = Reader [Elem]
Класс Reader идет из пакета Scala.util.parsing.input. Он похож на Stream, но также отслеживает позиции всех считываемых элементов. Тип Elem представляет собой индивидуальный ввод элементы. Это абстрактный тип член трейта Parsers:тип Elem
Это означает, что подклассы и субтитры Парсерам необходимо создать экземпляр класса Elem для типа ввода элементы, которые анализируются. Например, RegexParsers и JavaTokenParsers исправляет Elem, чтобы он был равен Char. Но это было бы также можно установить для Elem какой-либо другой тип, например тип токенов, возвращенных из отдельного лексера.Результаты парсера
Синтаксический анализатор может либо успешно, либо выйти из строя на некотором заданном входе. Следовательно, класс ParseResult имеет два подкласса для представления успеха и отказ:
запечатанный абстрактный класс ParseResult [+ T] case class Success [T] (результат: T, in: Input) расширяет ParseResult [T] case class Failure (msg: String, in: Input) расширяет ParseResult [ничего]
Случай Success переносит результат, возвращенный анализатором, в его параметр результата. Тип результатов парсера произвольный; это почему ParseResult, Success и Parser параметризованы с параметром типа T.Параметр типа представляет типы результатов возвращается данным парсером. Успех также принимает второй параметр, in, который относится к вводу, который следует сразу за частью, парсер поглотил. Это поле необходимо для объединения парсеров, поэтому что один парсер может работать за другим. Обратите внимание, что это чисто функциональный подход к парсингу. Ввод не читается как сторона эффект, но он сохраняется в потоке. Парсер анализирует некоторую часть входной поток, а затем возвращает оставшуюся часть в своем результате.Другой подкласс ParseResult — Отказ. Этот класс занимает в качестве параметра сообщение, описывающее причину сбоя парсера. Нравиться Успех, Неудача также принимает оставшийся входной поток как второй параметр. Это нужно не для цепочки (парсер не продолжить после сбоя), но разместить сообщение об ошибке в правильное место во входном потоке.
Обратите внимание, что результаты синтаксического анализа определены как ковариантные в типе параметр T. То есть синтаксический анализатор, возвращающий строки как результат, скажем, совместим с парсером, возвращающим AnyRefs.
Класс парсера
Предыдущая характеристика парсеров как функций из вводы для анализа результатов были немного упрощены. Предыдущие примеры показали, что парсеры также реализуют такие методы, как ~ for последовательная композиция двух парсеров и | за их альтернативу состав. Итак, Parser на самом деле является классом, унаследованным от тип функции Input => ParseResult [T] и дополнительно определяет следующие методы:
абстрактный класс Parser [+ T] расширяется (Input => ParseResult [T]) {P => def apply (in: Input): ParseResult [T]
Поскольку синтаксические анализаторы являются функциями (, т.е. , наследуются от), им необходимо определить применить метод. Вы видите абстрактный метод применения в классе Parser, но это только для документации, так как тот же метод в любом случае унаследованный от родительского типа Input => ParseResult [T] (напомним, что этот тип является аббревиатурой от scala.Function1 [Вход, ParseResult [T]]). Метод apply еще не реализован в отдельных синтаксических анализаторах, которые наследуются от абстрактного класса Parser.Эти парсеры будет обсуждаться после следующего раздела об этом псевдониме.
деф ~... def | ... ... }Наложение этого
Тело класса Parser начинается с любопытного выражения:
абстрактный класс Parser [+ T] расширяет ... {p =>
Предложение, такое как «id =>», сразу после открывающей фигурной скобки шаблон класса определяет идентификатор идентификатора как псевдоним для этого в класс. Как будто вы написали:val id = это
в теле класса, за исключением того, что компилятор Scala знает, что идентификатор псевдоним для этого.Например, вы можете получить доступ к объектно-частный член класса m, использующий id.m или this.m; эти два полностью эквивалентны. Первое выражение не будет компилироваться, если id были просто определены как val с этим как его правая рука стороне, потому что в этом случае компилятор Scala будет рассматривать id как нормальный идентификатор.Вы видели подобный синтаксис в Разделе 27.4, где он использовался, чтобы присвоить свой тип признаку. Псевдоним также может быть хорошим сокращением, когда вам нужно получить доступ к это внешнего класса.Вот пример:
class Outer {outer => class Inner { println (Внешний. этот эквалайзер) } }
В примере определяются два вложенных класса: Внешний и Внутренний. Внутри Внутреннее значение this внешнего класса упоминается дважды, используя разные выражения. Первое выражение показывает способ Java выполнения действий: перед зарезервированным словом this можно поставить префикс имя внешнего класса и точка; такое выражение тогда относится к this внешнего класса. Второе выражение показывает альтернатива, которую предоставляет вам Scala.Введя псевдоним с именем external для этого в классе Outer, вы можете ссылаться на этот псевдоним непосредственно также во внутренних классах. Способ Scala более краток и может также улучшится ясность, если вы правильно выберете имя псевдонима. Вы увидите примеры этого здесь и здесь.Парсеры одного токена
Class Parsers определяет общий элемент синтаксического анализатора, который может использоваться для проанализировать любой токен:
def elem (вид: String, p: Elem => Boolean) = новый парсер [Elem] { def применить (in: Input) = если (p (дюйм.первый)) Успех (in.first, in.rest) else Failure (вид + "ожидаемый", in) }
Этот парсер принимает два параметра: строку вида, описывающую, какой тип токена должен быть проанализирован и предикат p для Elems, который указывает, элемент соответствует классу анализируемых токенов.При применении синтаксического анализатора elem (kind, p) к некоторому входу в Первый элемент входного потока проверяется с помощью предиката p. Если p возвращает истину, синтаксический анализатор завершает работу. Его результатом является элемент сам, а его оставшийся вход — это входной поток, начинающийся только после проанализированного элемента.С другой стороны, если p возвращает false, синтаксический анализатор выдает сообщение об ошибке, указывающее, что своего рода токен ожидался.
Последовательная композиция
Парсер elem использует только один элемент. Разбирать более интересных фраз, вы можете связать парсеры вместе с оператор последовательной композиции ~. Как вы видели раньше, P ~ Q — это синтаксический анализатор, который сначала применяет синтаксический анализатор P к заданной входной строке. Затем, если P удастся, синтаксический анализатор Q применяется ко входу это остается после того, как P выполнил свою работу.
Комбинатор ~ реализован как метод в классе Парсер. Его определение показано в Листинге 31.6. Метод является членом парсера. класс. Внутри этого класса p определяется частью «p =>» как псевдоним этого, поэтому p обозначает левый операнд (или: приемник) ~. Его правый операнд представлен параметром q. Теперь, если p ~ q запускается на некотором вводе, сначала запускается p включен, и результат анализируется в соответствии с шаблоном. Если p успешно, q запускается на оставшемся входе in1. Если q также успешно, синтаксический анализатор в целом успешно.Его результатом является ~ объект содержащий как результат p (, т.е. , x), так и результат q (, то есть , y). С другой стороны, если либо p, либо q терпят неудачу, результат p ~ q — это объект Failure, возвращаемый функцией p или q.
абстрактный класс Parser [+ T] ... {p => ... def ~ [U] (q: => Parser [U]) = new Parser [T ~ U] { def apply (in: Input) = p (in) match { case Success (x, in1) => q (in1) match { case Success (y, in2) => Success (new ~ (x, y), in2) случай сбой => сбой } случай сбой => сбой } }
Листинг 31.6 — Метод комбинатора ~.
Тип результата ~ — это синтаксический анализатор, который возвращает экземпляр класс case ~ с элементами типов T и U. выражение типа T ~ U — это просто более удобное сокращение для параметризованный тип ~ [T, U]. Как правило, Scala всегда интерпретирует операция двоичного типа, такая как A op B, как параметризованный тип op [A, B]. Это аналогично ситуации для паттернов, где двоичный шаблон P op Q также интерпретируется как приложение, , то есть , op (P, Q).{Case x ~ y => y}
Альтернативный состав
Альтернативный состав P | Q применяет либо P, либо Q к данный ввод. Сначала он пробует P. Если P успешно, весь синтаксический анализатор преуспевает с результатом P. В противном случае, если P не удается, то Q проверяется на том же входе, что и P. Результат Q тогда результат всего парсера.
Вот определение | как метод класса Parser:
def | (q: => Parser [T]) = новый Parser [T] { def apply (in: Input) = p (in) match { case s1 @ Success (_, _) => s1 случай отказа => q (дюйм) } }
Обратите внимание, что если P и Q не работают, то сообщение об ошибке определяется Q.Этот тонкий выбор обсуждается позже, в Раздел 31.9.Работа с рекурсией
Обратите внимание, что параметр q в методах ~ и | поименно — его типу предшествует =>. Этот означает, что фактический аргумент парсера будет оцениваться только тогда, когда q требуется, что должно быть только после выполнения p. Это позволяет писать рекурсивные парсеры, подобные следующим тот, который анализирует число, заключенное в произвольное количество круглых скобок:
def parens = FloatingPointNumber | "(" ~ parens ~ ")"
Если | и ~ взял параметров по значению , это определение будет немедленно вызвать переполнение стека, ничего не читая, потому что значение parens находится в середине его правой части.[U] (f: T => U): Parser [U] = new Parser [U] { def apply (in: Input) = p (in) match { case Success (x, in1) => Success (f (x), in1) случай сбой => сбой } } }Синтаксические анализаторы, которые не читают ввод
Также есть два парсера, которые не потребляют никаких входных данных: успешный и неудачный. В parser success (result) всегда успешно с заданным результатом. Ошибка синтаксического анализатора (msg) всегда завершается с ошибкой msg. Оба реализованы как методы в парсерах трейтов, внешний трейт, который также содержит класс Parser:
def success [T] (v: T) = new Parser [T] { def apply (in: Input) = Успех (v, in) } def failure (msg: String) = new Parser [Nothing] { def apply (in: Input) = Failure (msg, in) }
Вариант и повтор
В синтаксических анализаторах свойств также определены комбинаторы опций и повторений. opt, rep и repsep.{Case r ~ rs => r :: rs} | успех (Список ()) )
}31.7 Строковые литералы и регулярные выражения [ссылка]
Парсеры, которые вы видели до сих пор использовал строковые литералы и регулярные выражения для разбора отдельных слов. Поддержка этих происходит от RegexParsers, подтипа Парсеры:
trait RegexParsers extends Parsers {
Этот трейт более специализирован, чем парсеры трейтов, в том смысле, что он работает только для входов, представляющих собой последовательности символов:тип Elem = Char
Он определяет два метода, литерал и регулярное выражение, со следующими сигнатурами:неявный литерал def (s: String): Parser [String] =... неявное def regex (r: Regex): Parser [String] = ...
Обратите внимание, что у обоих методов есть неявный модификатор, поэтому они применяются автоматически. всякий раз, когда задана строка или регулярное выражение, но синтаксический анализатор ожидал. Это почему вы можете писать строковые литералы и регулярные выражения прямо в грамматике, без необходимости оборачивать их одним из этих методов. Например, парсер «(» ~ expr ~ «)» будет автоматически расширен до литерал («(«) ~ выражение ~ литерал («)»).Трейт RegexParsers также обрабатывает пробелы. между символами.Для этого он вызывает метод с именем handleWhiteSpace перед запуском синтаксического анализатора литерала или регулярного выражения. Метод handleWhiteSpace пропускает самую длинную входную последовательность, соответствующую whiteSpace. регулярное выражение, которое по умолчанию определено следующим образом:
защищенный val whiteSpace = "" "\ s +" "". r }
Если вы предпочитаете другую обработку белого пространства, вы можете переопределить whiteSpace val. Например, если вы хотите, чтобы пробелы вообще не пропускались, вы можете переопределить whiteSpace пустым регулярным выражением:объект MyParsers расширяет RegexParsers { переопределить val whiteSpace = "".р ... }
31.8 Лексирование и синтаксический анализ [ссылка]
Задача синтаксического анализа часто разделяется на две фазы. В фаза лексера распознает отдельные слова во входных данных и классифицирует их в некоторые классы токенов. Эта фаза также называется лексический анализ. Далее следует синтаксический анализ. фаза, анализирующая последовательности токенов. Синтаксический анализ также иногда просто называется синтаксическим анализом, хотя это немного неточно, лексический анализ также можно рассматривать как проблему синтаксического анализа.
Свойство Parsers, описанное в предыдущем разделе, можно использовать для любой фазы, потому что его входные элементы имеют абстрактный тип Elem. Для лексический анализ, Elem будет создан для Char, означающий отдельные символы, которые составить слово разбираются. Синтаксический анализатор, в свою очередь, создаст экземпляр Elem к типу токена, возвращаемому лексером.
Комбинаторы синтаксического анализаScala предоставляют несколько служебных классов для лексических и синтаксический анализ. Они содержатся в двух подпакетах, по одному для каждого вида анализа:
Scala.util.parsing.combinator.lexical scala.util.parsing.combinator.syntactical
Если вы хотите разделить парсер на отдельный лексер и синтаксический analyzer, вам следует обратиться к документации Scaladoc для этих пакетов. Но для простых синтаксических анализаторов обычно достаточно подхода на основе регулярных выражений, показанного ранее в этой главе.31.9 Сообщение об ошибке [ссылка]
Есть еще одна последняя тема, которая еще не была затронута: как парсер выдать сообщение об ошибке? Отчеты об ошибках для парсеров — это своего рода черное искусство.Одна из проблем заключается в том, что, когда парсер отклоняет какой-либо ввод, он вообще сталкивался с множеством разных неудач. Каждый альтернативный синтаксический анализ должен был потерпеть неудачу, причем рекурсивно в каждой точке выбора. Который из обычно многочисленных сбоев следует отправлять как сообщение об ошибке на Пользователь?
Библиотека синтаксического анализаScala реализует простую эвристику: среди всех отказов, тот, который произошел в последней позиции на входе, является выбрал. Другими словами, парсер выбирает самый длинный префикс, который все еще действителен и выдает сообщение об ошибке, в котором объясняется, почему анализ префикса не мог быть продолжен.Если есть несколько точек отказа в этой последней позиции, та, которая была выбран последним посещенным.
Например, рассмотрите возможность запуска парсера JSON на ошибочном адресе. книга, которая начинается строкой:
{"Name": Джон,
Самый длинный законный префикс этой фразы — «{» name «:». Таким образом, парсер JSON отметит слово John как ошибку. Парсер JSON ожидает значение на этом этапе, но Джон идентификатор, который не считается значением (предположительно, автор документа забыли заключить название в кавычки).Часть, которую ожидалось «ложь», происходит из того факта, что «ложь» это последняя альтернатива производства значения в JSON грамматика. Так что это была последняя неудача на данном этапе. Пользователи, которые знают подробно о грамматике JSON может восстановить сообщение об ошибке, но для неспециалистов это сообщение об ошибке, вероятно, удивит и может также вводить в заблуждение.
Более точное сообщение об ошибке можно создать, добавив «всеобъемлющая» точка отказа как последняя альтернатива значения производство:
значение по умолчанию: Parser [Any] = obj | обр | stringLit | floatPointNumber | "ноль" | "правда" | «ложь» | сбой («недопустимое начало значения»)
Это добавление не меняет набор входных данных, которые принимаются как действительные. документы.Реализация «последней возможной» схемы ошибки. в отчетах используется поле с именем lastFailure: in trait Parsers, чтобы отметить сбой, произошедший на последняя позиция во вводе:
var lastFailure: Option [Failure] = None
Поле инициализируется значением None. Он обновлен в конструкторе класса Failure:case class Failure (msg: String, in: Input) extends ParseResult [Nothing] {
Поле читается по фразе метод, который выдает последнее сообщение об ошибке в случае сбоя парсера. Вот реализация фразы в трейт-парсерах:
if (lastFailure.isDefined && lastFailure.get.in.pos <= in.pos) lastFailure = Некоторые (это) }def фраза [T] (p: Parser [T]) = new Parser [T] { lastFailure = Нет def apply (in: Input) = p (in) match { case s @ Success (out, in1) => если (in1.atEnd) s else Failure ("ожидаемый конец ввода", in1) случай f: Отказ => lastFailure } }
Метод фразы запускает свой анализатор аргументов p.Если p успешно с полностью израсходованный ввод, возвращается успешный результат p. Если p успешно, но ввод не читается полностью, ошибка с сообщением возвращается "ожидаемый конец ввода". Если p не работает, сбой или ошибка хранится в lastFailure. Обратите внимание, что лечение lastFailure не работает; он обновляется как побочный эффект конструктор Failure и сам метод фразы. А возможна функциональная версия той же схемы, но требует передачи значения lastFailure, хотя каждый синтаксический анализатор результат, независимо от того, является ли этот результат успехом или Отказ.31.10 Возврат по сравнению с LL (1) [ссылка]
Комбинаторы синтаксического анализатора используют обратное отслеживание для выбора между разные парсеры в альтернативе. В выражении P | Q, если P не работает, тогда Q запускается на том же входе, что и P. Это происходит даже если P проанализировал несколько токенов до сбоя. В этом случае то же самое токены будут снова проанализированы до
кв.Отслеживание с возвратом накладывает лишь несколько ограничений на то, как сформулировать грамматика, чтобы ее можно было разобрать. По сути, вам просто нужно избегать леворекурсивные производства.Такая продукция как:
expr знак равно выражение "+" термин | срок. всегда будет терпеть неудачу, потому что expr немедленно вызывает себя и, следовательно, никогда прогрессирует дальше. [1] С другой стороны, поиск с возвратом потенциально дорогостоящий, потому что ввод можно анализировать несколько раз. Рассмотрим, например, производство:
expr знак равно термин "+" выражение | срок. Что произойдет, если синтаксический анализатор expr применяется к входу, например (1 + 2) * 3 что составляет юридический термин? Будет испробована первая альтернатива, и потерпит неудачу при сопоставлении со знаком +. Тогда будет испробована вторая альтернатива на тот же срок, и это будет успешно. В конце концов срок закончился анализируется дважды.
Часто можно изменить грамматику, чтобы можно было избегали. Например, в случае арифметических выражений либо будет работать одно из следующих производств:
expr знак равно термин ["+" выражение]. expr знак равно термин \ {"+" термин \}. Многие языки допускают так называемые "LL (1)" грамматики. [2] Когда комбинаторный синтаксический анализатор сформирован из такой грамматики, он никогда не будет backtrack, т.е. , позиция ввода никогда не будет сброшена на более раннюю ценить. Например, грамматики для арифметических выражений и терминов JSON ранее в этой главе оба являются LL (1), поэтому возможности обратного отслеживания структура комбинатора синтаксического анализатора никогда не используется для входных данных от эти языки.
Фреймворк синтаксического анализа комбинатора позволяет выразить ожидания что грамматика является LL (1) явно, с использованием нового оператора ~ !. Этот оператор похож на последовательную композицию ~, но никогда не будет возврат к «непрочитанным» элементам ввода, которые уже были проанализированы. Используя этот оператор, результаты синтаксического анализа арифметических выражений могут в качестве альтернативы можно записать следующим образом:
def expr: Parser [Any] = срок ~! rep ("+" ~! срок | "-" ~! срок) def term: Parser [Any] = фактор ~! rep ("*" ~! фактор | "/" ~! фактор) def-фактор: Parser [Any] = "(" ~! Expr ~! ")" | floatPointNumber
Одним из преимуществ парсера LL (1) является то, что он может использовать более простой ввод техника.Входные данные можно читать последовательно, а входные элементы могут быть отбрасываются после их прочтения. Это еще одна причина, по которой парсеры LL (1) обычно более эффективны, чем парсеры с возвратом.31.11 Заключение [ссылка]
Вы ознакомились со всеми основными элементами синтаксического анализа комбинатора Scala. рамки. На удивление мало кода для чего-то действительно полезный. С помощью фреймворка вы можете создавать парсеры для большого класса контекстно-свободных грамматик. Фреймворк позволяет начать работу быстро, но он также может быть настроен для новых видов грамматики и ввода методы.Будучи библиотекой Scala, она легко интегрируется с остальными. языка. Так что легко интегрировать синтаксический анализатор комбинатора в большая программа Scala.
Обратной стороной комбинаторных синтаксических анализаторов является то, что они не очень эффективен, по крайней мере, по сравнению с синтаксическими анализаторами, созданными для специального назначения такие инструменты, как Yacc или Bison. На это есть две причины. Первый, метод обратного отслеживания, используемый синтаксическим анализом комбинатора, сам по себе не очень эффективный. В зависимости от грамматики и ввода синтаксического анализа он может приводят к экспоненциальному замедлению из-за многократного отката.Это может быть исправлено путем создания грамматики LL (1) и использования зафиксированных последовательных оператор композиции ~ !.
Вторая проблема, влияющая на производительность синтаксических анализаторов комбинаторов: что они смешивают конструкцию парсера и анализ ввода в одном наборе операций. Фактически, синтаксический анализатор генерируется заново для каждого ввода. это проанализировано.
Эту проблему можно преодолеть, но для этого требуется другой реализация фреймворка синтаксического комбинатора. В оптимизирующем framework, синтаксический анализатор больше не будет представлен как функция из входы для анализа результатов.Вместо этого он будет представлен в виде дерева, где каждый шаг конструкции был представлен как класс case. Для Например, последовательная композиция может быть представлена классом case Seq, альтернатива по Alt и так далее. Самый "внешний" парсер метод, фраза, могли бы тогда принять это символическое представление синтаксический анализатор и преобразовать его в высокоэффективные таблицы синтаксического анализа, используя стандартные алгоритмы генератора парсеров.
Что хорошо во всем этом, так это то, что с точки зрения пользователя ничего изменения по сравнению с обычными синтаксическими анализаторами комбинаторов.Пользователи все еще пишут синтаксические анализаторы с точки зрения идентификатора, числа с плавающей точкой, ~, | и т. д. Им не нужно знать, что эти методы генерируют символическое представление парсера вместо функции парсера. Поскольку комбинатор фраз преобразует эти представления в реальные синтаксические анализаторы, все работает как раньше.
Преимущество этой схемы по производительности двоякое. Во-первых, теперь вы можете исключить конструкцию парсера из анализа ввода. Если бы вы написали:
val jsonParser = фраза (значение)
а затем примените jsonParser к нескольким различным входам, jsonParser будет создаваться только один раз, а не каждый раз при чтении ввода.Во-вторых, генерация парсера может использовать эффективные алгоритмы синтаксического анализа. например LALR (1). [3] Эти алгоритмы обычно приводят к большому более быстрые парсеры, чем парсеры, которые работают с возврат.
В настоящее время такого оптимизирующего генератора парсеров еще не было. написан для Scala. Но это было бы вполне возможно. Если кто-то вносит такой генератор, интегрировать будет несложно в стандартную библиотеку Scala. Даже если предположить, что такой генератор будет существовать в какой-то момент будущее, однако есть причины для сохранения текущего парсера рамки комбинатора вокруг.Это намного легче понять и для адаптации, чем генератор парсера, а разница в скорость часто не имеет значения на практике, если вы не хотите разбирать очень большие входы.
Сноски к главе 31:
[1] Есть способы избежать переполнения стека. даже при наличии левой рекурсии, но для этого требуется более совершенная структура комбинатора синтаксического анализа, которая на сегодняшний день имеет не реализовано.
[2] Aho, et. al. , г. Компиляторы: принципы, методы и инструменты .ахо-сетхи-ульман
[3] Aho, et. al. , Компиляторы: принципы, методы и инструменты . ахо-сетхи-ульман
Улучшение композиционности вложения слов с использованием лексикографических определений
% PDF-1.5 % 1 0 obj > / OCG [5 0 R] >> / OpenAction 6 0 R / Контуры 7 0 R / PageLabels> / PageMode / UseOutlines / Страницы 9 0 R / Тип / Каталог >> эндобдж 10 0 obj > эндобдж 2 0 obj > / Шрифт> >> / Поля [] >> эндобдж 3 0 obj > транслировать application / pdf
- Thijs Scheepers, Evangelos Kanoulas и Efstratios Gavves
- Улучшение композиционности вложения слов с использованием лексикографических определений 2018-02-19T08: 22: 59ZLaTeX с acmart 2018/02/07 v1.50 наборных статей для Association for Computing Machinery and hyperref 2016/06/24 v6.83q Гипертекстовые ссылки для LaTeX2019-07-05T16: 29: 05 + 02: 002019-07-05T16: 29: 05 + 02: 00pdfTeX-1.40. 17FalseЭто pdfTeX, версия 3.14159265-2.6-1.40.17 (TeX Live 2016 / Debian) kpathsea версия 6.2.2uuid: 87ca0d57-bbc6-4649-a016-17dfc19bc428uuid: d1671e0b-8ef6-4c24-aa836e3624a конечный поток эндобдж 4 0 obj > эндобдж 5 0 obj > >> >> эндобдж 6 0 obj > эндобдж 7 0 объект > эндобдж 8 0 объект > эндобдж 9 0 объект > эндобдж 11 0 объект > эндобдж 12 0 объект > эндобдж 13 0 объект > эндобдж 14 0 объект > эндобдж 15 0 объект > / Шрифт> / ProcSet [/ PDF / Text] / XObject> >> / Повернуть 0 / Тип / Страница >> эндобдж 16 0 объект >> эндобдж 17 0 объект >> эндобдж 18 0 объект > эндобдж 19 0 объект > эндобдж 20 0 объект > эндобдж 21 0 объект > эндобдж 22 0 объект > эндобдж 23 0 объект > / Граница [0 0 0] / C [1 0 0] /ПРИВЕТ / Rect [84.961 260,561 94,366 269,937] / Подтип / Ссылка / Тип / Аннотация >> эндобдж 24 0 объект > / Граница [0 0 0] / C [0 1 1] /ПРИВЕТ / Rect [116,547 260,561 247,846 269,937] / Подтип / Ссылка / Тип / Аннотация >> эндобдж 25 0 объект > / Граница [0 0 0] / C [0 1 0] /ПРИВЕТ / Rect [181,311 225,585 191,642 233,213] / Подтип / Ссылка / Тип / Аннотация >> эндобдж 26 0 объект > / Граница [0 0 0] / C [0 1 0] /ПРИВЕТ / Rect [254,81 181,749 265,141 189,377] / Подтип / Ссылка / Тип / Аннотация >> эндобдж 27 0 объект > / Граница [0 0 0] / C [0 1 1] /ПРИВЕТ / Rect [52.802 81,581 167,938 90,78] / Подтип / Ссылка / Тип / Аннотация >> эндобдж 28 0 объект > / Граница [0 0 0] / C [0 1 0] /ПРИВЕТ / Rect [416,49 593,916 426,821 601,544] / Подтип / Ссылка / Тип / Аннотация >> эндобдж 29 0 объект > / Граница [0 0 0] / C [0 1 0] /ПРИВЕТ / Rect [354,116 495,286 364,447 502,914] / Подтип / Ссылка / Тип / Аннотация >> эндобдж 30 0 объект > / Граница [0 0 0] / C [0 1 0] /ПРИВЕТ / Rect [430,993 451,45 441,324 459,078] / Подтип / Ссылка / Тип / Аннотация >> эндобдж 31 0 объект > / Граница [0 0 0] / C [0 1 0] /ПРИВЕТ / Rect [457.583 232,272 467,915 239,9] / Подтип / Ссылка / Тип / Аннотация >> эндобдж 32 0 объект > / Граница [0 0 0] / C [0 1 0] /ПРИВЕТ / Rect [499,6 232,348 509,931 239,9] / Подтип / Ссылка / Тип / Аннотация >> эндобдж 33 0 объект > / Граница [0 0 0] / C [0 1 0] /ПРИВЕТ / Rect [549.793 232.272 555.955 239.9] / Подтип / Ссылка / Тип / Аннотация >> эндобдж 34 0 объект > / Граница [0 0 0] / C [0 1 0] /ПРИВЕТ / Rect [374,883 221,313 385,214 228,941] / Подтип / Ссылка / Тип / Аннотация >> эндобдж 35 0 объект > / Граница [0 0 0] / C [0 1 0] /ПРИВЕТ / Rect [463.ʚVq \% n! iɳc3 S "̙lkoGi! [}} ξW n! f9T $ c \ -,
c G, * kby0lal | Hf {6
Рекурсивные нейронные сети с PyTorch
Глубокие нейронные сети от Siri до Google Translate сделали возможным прорыв в машинном понимании естественного языка. Большинство из этих моделей рассматривают язык как плоскую последовательность слов или символов и используют своего рода модель, называемую рекуррентной нейронной сетью (RNN), для обработки этой последовательности. Но многие лингвисты считают, что язык лучше всего понимать как иерархическое дерево фраз, поэтому значительное количество исследований было посвящено моделям глубокого обучения, известным как рекурсивные нейронные сети , , которые учитывают эту структуру.Хотя эти модели, как известно, сложны в реализации и неэффективны в эксплуатации, новая платформа глубокого обучения под названием PyTorch значительно упрощает эти и другие сложные модели обработки естественного языка.
Хотя рекурсивные нейронные сети являются хорошей демонстрацией гибкости PyTorch, это также полнофункциональная среда для всех видов глубокого обучения с особенно сильной поддержкой компьютерного зрения. Фреймворк, созданный разработчиками из Facebook AI Research и нескольких других лабораторий, сочетает в себе эффективные и гибкие серверные библиотеки с ускорением на GPU из Torch7 с интуитивно понятным интерфейсом Python, который фокусируется на быстром прототипировании, читаемом коде и поддержке максимально широкого разнообразия глубоких интерфейсов. модели обучения.
ВРАЩЕНИЕ
В этом посте рассматривается реализация PyTorch рекурсивной нейронной сети с рекуррентным трекером и узлами TreeLSTM, также известная как SPINN - пример модели глубокого обучения на основе обработки естественного языка, которую сложно построить во многих популярных фреймворках. Описываемая мной реализация также является частично пакетной, поэтому она может использовать ускорение графического процессора для работы значительно быстрее, чем версии, в которых не используется пакетная обработка.
Эта модель, обозначающая нейронную сеть синтаксического интерпретатора с расширенным стеком, была представлена в Bowman et al.(2016) как способ решения задачи логического вывода на естественном языке с использованием набора данных SNLI Стэнфорда.
Задача состоит в том, чтобы разделить пары предложений на три категории: если предположить, что первое предложение является точной подписью для невидимого изображения, тогда предложение два (а) определенно, (б) возможно, или (в) определенно не является точным заголовком. ? (Эти классы называются следствием, нейтральным и противоречивым соответственно). Например, предположим, что первое предложение - «две собаки бегают по полю.Тогда предложение, которое сделало бы пару следствием этого, могло бы быть «на улице животные», одно, которое сделало бы пару нейтральным, могло бы быть «некоторые щенки бегут, чтобы поймать палку», и третье, которое сделало бы это противоречием, могло бы быть «Домашние животные сидят на диване».
В частности, цель исследования, которое привело к SPINN, заключалась в том, чтобы сделать это путем кодирования каждого предложения в векторное представление фиксированной длины перед определением их взаимосвязи (есть и другие способы, такие как модели внимания, которые сравнивают отдельные части каждого предложения с друг друга, используя своего рода мягкий фокус).
Набор данных поставляется с сгенерированными машиной синтаксическими деревьями синтаксического анализа, которые группируют слова в каждом предложении по фразам и предложениям, которые имеют независимое значение и каждое состоит из двух слов или вложенных фраз. Многие лингвисты считают, что люди понимают язык, комбинируя значения в иерархическом порядке, как описано подобными деревьями, поэтому, возможно, стоит попытаться построить нейронную сеть, которая работает таким же образом. Вот пример предложения из набора данных, дерево синтаксического анализа которого представлено вложенными круглыми скобками:
((Церковь) ((имеет (трещины (в (потолке)))).))
Одним из способов кодирования этого предложения с помощью нейронной сети, которая учитывает дерево синтаксического анализа, было бы создание слоя нейронной сети Reduce, который объединяет пары слов (представленных встраиваемыми словами, такими как GloVe) и / или фразы, а затем рекурсивно применяет этот слой , принимая результат последней операции Reduce как кодировку предложения:
X = Уменьшить («потолок») Y = Уменьшить («in», X) ... и т. д.
Но что, если я хочу, чтобы сеть работала еще более человечно, читая слева направо и поддерживая контекст предложения, при этом комбинируя фразы с помощью дерева синтаксического анализа? Или что, если я хочу научить сеть строить собственное дерево синтаксического анализа, когда она читает предложение, на основе слов, которые она видит? Вот то же дерево синтаксического анализа, написанное немного иначе:
В церкви) в потолке трещины)))).))
Или третий способ, опять же эквивалент:
СЛОВА: В церкви трещины в потолке. ПАРСЫ: S S R S S S S S R R R R S R R R
Все, что я сделал, это удалил открытые скобки, затем пометил слова буквой «S» для «сдвига» и заменил закрывающие круглые скобки на «R» для «уменьшить». Но теперь информацию можно читать слева направо как набор инструкций для управления стеком и стеклоподобным буфером, с точно такими же результатами, что и рекурсивный метод, описанный выше:
- Поместите слова в буфер.
- Вытащите букву «The» из передней части буфера и вставьте ее в стопку, затем нажмите «церковь».
- Выдвинуть два верхних значения стека, применить «Уменьшить», затем отправить результат обратно в стек.
- Pop «имеет» из буфера и толкать в стопку, затем «трещины», затем «внутрь», затем «the», затем «потолок».
- Повторите четыре раза: вытащите два верхних значения стека, примените «Уменьшить», затем нажмите результат.
- Pop "." из буфера и поместить в стек.
- Повторите два раза: вытащите два верхних значения стека, примените «Уменьшить», затем нажмите результат.
- Извлечь оставшееся значение стека и вернуть его как кодировку предложения.
Я также хочу сохранить контекст предложения, чтобы учесть информацию о частях предложения, которые система уже прочитала при выполнении операций сокращения над последующими частями предложения. Поэтому я заменю функцию Reduce
с двумя аргументами функцией с тремя аргументами, которая принимает левую дочернюю фразу, правую дочернюю фразу и текущее состояние контекста предложения. Это состояние создается вторым уровнем нейронной сети, повторяющимся блоком, который называется Tracker
. Tracker
создает новое состояние на каждом этапе манипулирования стеком (то есть после чтения каждого слова или закрывающей скобки) с учетом текущего состояния контекста предложения, верхней записи b в буфере и двух верхних записей s1 , s2 в стеке:
context [t + 1] = Tracker (context [t], b, s1, s2)
Вы легко можете себе представить, как писать код для этих вещей на вашем любимом языке программирования. Для каждого обрабатываемого предложения он будет загружать следующее слово из буфера, запускать Tracker, проверять, нужно ли помещать в стек или выполнять сокращение, выполнять эту операцию, а затем повторять, пока предложение не будет завершено.Применительно к одному предложению этот процесс представляет собой большую и сложную глубокую нейронную сеть с двумя обучаемыми слоями, применяемыми снова и снова способами, определяемыми манипуляциями со стеком. Но если вы знакомы с традиционными фреймворками глубокого обучения, такими как TensorFlow или Theano, сложно реализовать такую динамическую процедуру. Стоит отступить и потратить немного времени на изучение того, почему это так и что PyTorch делает иначе.
Теория графов
Рисунок 1: Графическое представление структуры функции.Глубинные нейронные сети - это, по сути, просто сложные функции с большим количеством параметров. Целью глубокого обучения является оптимизация этих параметров путем вычисления их частных производных (градиентов) по метрике потерь. Если функция представлена в виде графической структуры вычислений (рисунок 1), то обратный ход этого графа позволяет вычислять эти градиенты без какой-либо избыточной работы. Каждая современная структура для глубокого обучения основана на этой концепции обратного распространения ошибки , и в результате каждая структура нуждается в способе представления графов вычислений.
Во многих популярных фреймворках, включая TensorFlow, Theano и Keras, а также в библиотеке Torch7 nngraph
граф вычислений представляет собой статический объект, который создается заранее. График определяется с помощью кода, который выглядит как математическое выражение, но переменные которого на самом деле являются заполнителями, которые еще не содержат никаких числовых значений. Этот график переменных-заполнителей компилируется один раз в функцию, которую затем можно многократно запускать на пакетах обучающих данных для получения выходных данных и градиентов.
Этот вид статического графа вычислений хорошо работает для сверточных сетей, структура которых обычно является фиксированной. Но во многих других приложениях было бы полезно, если бы структура графа нейронных сетей могла изменяться в зависимости от данных. При обработке естественного языка исследователи обычно хотят развернуть повторяющиеся нейронные сети на столько временных шагов, сколько слов во входных данных. Манипулирование стеком в модели SPINN, описанной выше, в значительной степени зависит от потока управления, такого как для операторов
и if,
для определения структуры графа вычислений для конкретного предложения.В еще более сложных случаях вы можете захотеть построить модели, структура которых зависит от выходных данных подсетей внутри самой модели.
Некоторые (хотя и не все) из этих идей можно впихнуть в системы со статическими графами, но почти всегда ценой снижения прозрачности и запутанного кода. Платформа должна добавлять специальные узлы в свои графы вычислений, которые представляют примитивы программирования, такие как циклы и условные выражения, в то время как пользователи должны изучать и использовать эти узлы, а не инструкции для
и if
на языке, на котором они пишут свой код. .Это связано с тем, что любые операторы потока управления, которые использует программист, будут выполняться только один раз, когда граф построен, жестко кодируя единственный путь вычисления.
Например, для запуска рекуррентного модуля нейронной сети ( rnn_unit
) над векторами в словах (начиная с начального состояния h0
) требуется tf. while_loop
, специальный узел потока управления, в TensorFlow. Дополнительный специальный узел необходим для получения длины слов во время выполнения, поскольку он является только заполнителем во время выполнения кода.
# TensorFlow # (этот код запускается один раз при инициализации модели) # "Words" - это не настоящий список (это переменная-заполнитель), поэтому # Я не могу использовать "len" cond = lambda i, h: iПринципиально иной подход, впервые использованный в течение десятилетий академической работы, включая Harvard's Kayak и autograd, а также в исследовательских фреймворках Chainer и DyNet, основан на графах динамических вычислений .В такой структуре, также известной как определение за запуском, граф вычислений строится и перестраивается во время выполнения с тем же кодом, который выполняет вычисления для прямого прохода, также создавая структуру данных, необходимую для обратного распространения. Этот подход дает более простой код, поскольку поток управления может быть написан с использованием стандарта
для
идля
. Это также упрощает отладку, потому что точка останова во время выполнения или трассировка стека приведет вас к написанному вами коду, а не к скомпилированной функции в механизме выполнения.Та же самая рекуррентная нейронная сеть переменной длины может быть реализована с помощью простого цикла for Python в динамической структуре.# PyTorch (также работает в Chainer) # (этот код запускается при каждом прямом проходе модели) # «Слова» - это список Python с фактическими значениями в нем h = h0 словом в словах: h = rnn_unit (слово, h)PyTorch - это первая среда глубокого обучения, определяемая по запуску, которая соответствует возможностям и производительности структур статических графов, таких как TensorFlow, что делает ее подходящей для всего, от стандартных сверточных сетей до самых смелых идей обучения с подкреплением.Итак, давайте перейдем к рассмотрению реализации SPINN.
Обзор кода
Прежде чем я начну строить сеть, мне нужно настроить загрузчик данных. В глубоком обучении модели часто работают с пакетами примеров данных, ускоряют обучение за счет параллелизма и имеют более плавный градиент на каждом этапе. Я хотел бы иметь возможность сделать это здесь (позже я объясню, как можно группировать описанный выше процесс манипулирования стеком). Следующий код Python загружает некоторые данные с помощью системы, встроенной в текстовую библиотеку PyTorch, которая автоматически создает пакеты, объединяя вместе примеры одинаковой длины.После запуска этого кода
train_iter
,dev_iter
иtest_iter
содержат итераторы, которые циклически перебирают пакеты в поезде, проверку и тестовые разбиения SNLI.из данных импорта torchtext, наборов данных ТЕКСТ = datasets.snli.ParsedTextField (нижний = True) ПЕРЕХОДЫ = datasets.snli.ShiftReduceField () LABELS = data.Field (последовательный = False) train, dev, test = набор данных.SNLI.splits ( ТЕКСТ, ПЕРЕХОДЫ, МЕТКИ, wv_type = 'glove.42B') TEXT.build_vocab (поезд, разработчик, тест) train_iter, dev_iter, test_iter = данные.BucketIterator.splits ( (поезд, разработка, тест), batch_size = 64)Остальной код для настройки таких вещей, как цикл обучения и метрики точности, можно найти в
train.py
. Перейдем к модели. Как описано выше, кодер SPINN содержит параметризованный уровеньReduce
и необязательный повторяющийся трекерTracker
для отслеживания контекста предложения путем обновления скрытого состояния каждый раз, когда сеть считывает слово или применяетReduce
; в следующем коде говорится, что создание SPINN означает просто создание этих двух подмодулей (мы скоро увидим их код) и помещение их в контейнер для дальнейшего использования.импортная горелка из факела импорт нн # создать подкласс класса Module из пакета нейронной сети PyTorch класс SPINN (nn.Module): def __init __ (self, config): super (SPINN, сам) .__ init __ () self.config = config self.reduce = Уменьшить (config.d_hidden, config.d_tracker) если config.d_tracker не равен None: self.tracker = Трекер (config.d_hidden, config.d_tracker)
SPINN .__ init__
вызывается один раз при создании модели; он выделяет и инициализирует параметры, но не выполняет никаких операций нейронной сети и не строит какой-либо граф вычислений.Код, который запускается для каждого нового пакета данных, определяется в методеSPINN.forward
, стандартном имени PyTorch для реализованного пользователем метода, который определяет прямой проход модели. По сути, это просто реализация описанного выше алгоритма манипулирования стеком в обычном Python, работающего с пакетом буферов и стеков - по одному для каждого примера. Я перебираю набор операций «сдвиг» и «уменьшение», содержащихся в переходах, запускаюTracker
, если он существует, и просматриваю каждый пример в пакете, чтобы применить операцию «сдвиг», если требуется, или добавить ее в список примеры, которым нужна операция «уменьшить».Затем я запускаю слойReduce
для всех примеров в этом списке и помещаю результаты обратно в соответствующие стеки.def вперед (себя, буферы, переходы): # Входные данные представляют собой единый тензор вложений слов; # Мне нужно, чтобы это был список стеков, по одному для каждого примера в # пакет, из которого мы можем извлечь самостоятельно. Слова в # каждый пример уже перевернут, так что они могут # читать слева направо, нажимая на конец каждого # список; они также имеют префикс с нулевым значением.buffers = [список (torch.split (b.squeeze (1), 1, 0)) для b в torch.split (buffers, 1, 1)] # нам также нужны два нулевых значения внизу каждого стека, # чтобы мы могли копировать нули во входных данных; эти нули # необходимы, чтобы трекер мог работать, даже если # буфер или стек пуст stacks = [[buf [0], buf [0]] для буфера в буферах] если hasattr (self, 'трекер'): self.tracker.reset_state () для trans_batch в переходах: если hasattr (self, 'трекер'): # Ранее я описал трекер как принимающий 4 # аргументы (context_t, b, s1, s2), но здесь я # предоставить содержимое стека как один аргумент # при сохранении контекста внутри трекера # сам объект.tracker_states, _ = self.tracker (буферы, стеки) еще: tracker_states = itertools.repeat (Нет) левые, правые, трекинг = [], [], [] batch = zip (trans_batch, buffers, stacks, tracker_states) для перехода, buf, stack, отслеживания в пакетном режиме: если переход == SHIFT: stack.append (buf.pop ()) elif transition == УМЕНЬШИТЬ: права.append (stack.pop ()) левые.добавить (stack.pop ()) trackings.append (отслеживание) если права: уменьшенный = iter (self.reduce (влево, вправо, отслеживание)) для перехода складывать в zip (trans_batch, stacks): если переход == УМЕНЬШИТЬ: stack.append (следующий (уменьшенный)) return [stack.pop () для стека в стеках]Вызов
self.tracker
илиself.reduce
запускает метод пересылки подмодуляTracker
илиReduce
соответственно, который принимает список примеров для применения операции.Имеет смысл работать независимо с различными примерами здесь, в основном методе пересылки, сохраняя отдельные буферы и стеки для каждого из примеров в пакете, поскольку выполняются все математически тяжелые операции с ускорением на GPU, которые выигрывают от пакетного выполнения. вTracker
иУменьшите
. Чтобы писать эти функции более чисто, я воспользуюсь некоторыми помощниками (которые я определю позже), которые превращают эти списки примеров в пакетные тензоры и наоборот.Мне бы хотелось, чтобы модуль
Рисунок 2: Функция композиции TreeLSTM, дополненная третьим входом (x, в данном случае состояние трекера). В реализации PyTorch, показанной ниже, пять групп из трех линейных преобразований (представленных тройками синих, черных и красных стрелок) были объединены в три модуля nn.Linear, а функция tree_lstm выполняет все вычисления, расположенные внутри блока. Рисунок Чена и др.(2016).Reduce
автоматически группировал свои аргументы для ускорения вычислений, а затем разупаковывал их, чтобы их можно было независимо вставлять и извлекать позже.Фактическая функция композиции, используемая для объединения представлений каждой пары левых и правых подфраз в представление родительской фразы, представляет собой TreeLSTM, разновидность обычного рекуррентного блока нейронной сети, называемого LSTM. Эта функция композиции требует, чтобы состояние каждого из дочерних элементов фактически состояло из двух тензоров, скрытого состоянияh
и состояния ячейки памятиc
, в то время как функция определяется с использованием двух линейных слоев (nn.Linear
), работающих на скрытых состояниях детей и нелинейной комбинированной функцииtree_lstm
, которая объединяет результат линейных слоев с состояниями ячеек памяти детей.В SPINN это расширяется за счет добавления третьего линейного слоя, который работает со скрытым состоянием трекера.def tree_lstm (c1, c2, lstm_in): # Принимает состояния ячеек памяти (c1, c2) двух потомков, как # а также сумма линейных преобразований детского # скрытых состояний (lstm_in) # Эта сумма преобразованных скрытых состояний разбивается на # кандидат выводит a и четыре логических элемента (i, f1, f2 и o). a, i, f1, f2, o = lstm_in.chunk (5, 1) c = a.tanh () * i.sigmoid () + f1.sigmoid () * c1 + f2.sigmoid () * c2 h = o.sigmoid () * c.tanh () вернуть h, c класс Reduce (nn.Модуль): def __init __ (self, size, tracker_size = None): super (Уменьшить, сам) .__ init __ () self.left = nn.Linear (размер, 5 * размер) self.right = nn.Linear (размер, 5 * размер, смещение = False) если tracker_size не None: self.track = nn.Linear (tracker_size, 5 * size, bias = False) def forward (self, left_in, right_in, tracking = None): слева, справа = партия (left_in), batch (right_in) отслеживание = партия (отслеживание) lstm_in = self.left (слева [0]) lstm_in + = себя.вправо (вправо [0]) если hasattr (self, 'track'): lstm_in + = self.track (отслеживание [0]) return unbatch (tree_lstm (left [1], right [1], lstm_in))Поскольку и уровень
Reduce
, и аналогично реализованныйTracker
работают с использованием LSTM, вспомогательные функцииbatch
иunbatch
работают с парами скрытых состояний и состояний памяти(h, c)
.def batch (указывает): если состояния нет: return None состояния = кортеж (состояния) если состояния [0] равно Нет: return None # состояния - это список тензоров B размерности (1, 2H) # это возвращает два тензора размерности (B, H) вернуть факел.кошка (состояния, 0) .chunk (2, 1) def unbatch (состояние): если состояние None: вернуть itertools.repeat (Нет) # состояние - это пара тензоров размерности (B, H) # это возвращает список тензоров B размерности (1, 2H) вернуть torch.split (torch.cat (состояние, 1), 1, 0)Вот и все. (Остальной необходимый код, включая
Tracker
, находится вspinn.py
, в то время как уровни классификатора, которые вычисляют категорию SNLI из двух кодировок предложений и сравнивают этот результат с целью, дающей окончательную переменнуюпотери
, являются в модели.py
). Прямой код для SPINN и его подмодулей создает чрезвычайно сложный граф вычислений (рисунок 3), кульминацией которого является потеря, детали которого полностью различаются для каждого пакета в наборе данных, но который может каждый раз автоматически передаваться в обратном направлении с очень небольшими накладными расходами просто с помощью вызов
loss.backward ()
, функцию, встроенную в PyTorch, которая выполняет обратное распространение из любой точки графика.Модели и гиперпараметры в полном коде могут соответствовать производительности, указанной в исходной статье SPINN, но их обучение на графическом процессоре в несколько раз быстрее, поскольку реализация полностью использует преимущества пакетной обработки и эффективности PyTorch.В то время как исходная реализация занимает 21 минуту для компиляции графа вычислений (это означает, что цикл отладки во время реализации по крайней мере такой длинный), затем около пяти дней для обучения, версия, описанная здесь, не имеет этапа компиляции и занимает около 13 часов для обучения. GPU Tesla K40 или около 9 часов на Quadro GP100.
Рисунок 3: Небольшая часть графа вычислений для SPINN с размером пакета два, на котором выполняется версия кода Chainer, представленная в этом посте.Вызов подкрепления
Версия описанной выше модели без
Tracker
на самом деле довольно хорошо подходит для нового tf TensorFlow.fold предметно-ориентированный язык для особых случаев динамических графиков, но версию сTracker
будет намного сложнее реализовать. Это связано с тем, что добавлениеTracker
означает переход от рекурсивного подхода к методам на основе стека. Это (как в приведенном выше коде) наиболее просто реализовать с помощью условных переходов, которые зависят от значений ввода. Но в Fold отсутствует встроенная операция условного ветвления, поэтому структура графа в модели, построенной с ее помощью, может зависеть только от структуры ввода, а не от его значений.Кроме того, было бы практически невозможно создать версию SPINN, для которойTracker
решает, как анализировать входное предложение при его чтении, поскольку структуры графа в Fold - хотя они зависят от структуры входного примера - должны быть полностью исправлено после загрузки примера ввода.Одна такая модель была исследована исследователями из DeepMind и Google Brain, которые применили обучение с подкреплением для обучения трекера SPINN синтаксическому анализу входных предложений без использования каких-либо внешних данных синтаксического анализа.По сути, такая модель начинается со случайного предположения и обучается, вознаграждая себя, когда ее синтаксический анализ дает хорошую точность для общей задачи классификации. Исследователи написали, что они «используют размер пакета 1, поскольку граф вычислений необходимо реконструировать для каждого примера на каждой итерации в зависимости от образцов из сети политик [Tracker]», но PyTorch позволит им использовать пакетное обучение даже в сети. как этот со сложной, стохастически меняющейся структурой.
PyTorch также является первым фреймворком, в библиотеку которого встроено обучение с подкреплением (RL) в виде графов стохастических вычислений , что делает использование RL градиента политики столь же простым, как и обратное распространение. Чтобы добавить его в модель, описанную выше, вам просто нужно переписать первые несколько строк основного цикла SPINN for следующим образом, что позволяет
Tracker
определять вероятность выполнения каждого типа перехода синтаксического анализатора.! # Nn.functional содержит операции нейронной сети без параметров от факела.nn import функционал как F переходы = [] for i in range (len (buffers [0]) * 2-3): # мы знаем, сколько шагов # получить необработанные оценки для каждого типа перехода парсера tracker_states, transition_scores = self.tracker (буферы, стеки) # использовать функцию softmax для нормализации оценок в вероятности, # затем выберите из распределения, которое определяют эти вероятности transition_batch = F.softmax (transition_scores) .multinomial () transitions.append (transition_batchЗатем, когда пакет прошел полностью и модель знает, насколько точно он предсказал свои категории, я могу отправить сигналы вознаграждения обратно через эти узлы графа стохастических вычислений в дополнение к обратному распространению через остальную часть графа традиционным способом:
# loss должен содержать потерю для примера, а mean и std # представляют средние значения по многим партиям вознаграждение = (-потери - среднее) / стандартное для перехода в переходах: переход.усилить (награды) # подключаем стохастические узлы к конечной переменной потерь # чтобы их можно было найти, умножив на ноль # потому что этот трюк не должен изменить значение убытка loss = loss.mean () + 0 * сумма (переходов) .sum () # выполнить обратное распространение через детерминированные узлы и # градиент политики RL для стохастических узлов loss.backward ()Исследователи Google сообщили о результатах SPINN плюс RL, которые были немного лучше, чем исходные SPINN, полученные на SNLI, несмотря на то, что версия RL не использовала предварительно вычисленную информацию дерева синтаксического анализа.Область глубокого обучения с подкреплением для обработки естественного языка является совершенно новой, и исследовательские проблемы в этой области широко открыты; за счет встраивания RL в структуру PyTorch значительно снижает входной барьер.
Подробнее на GTC
Приходите на конференцию по технологиям графических процессоров, которая состоится 8–11 мая в Сан-Хосе, штат Калифорния, чтобы узнать больше о глубоком обучении и PyTorch. GTC - крупнейшее и самое важное событие года для разработчиков AI и GPU. Используйте код CMDLIPF, чтобы получить скидку 20% на регистрацию!
Присоединяйтесь ко мне в GTC и узнайте больше о моей работе в моем выступлении со Стивеном Мерити «Квази-рекуррентные нейронные сети - высокооптимизированная архитектура RNN для графического процессора» (S7265).Вам также понравится доклад Сумита Чинтала «PyTorch, платформа для исследований искусственного интеллекта нового поколения».
Начните работу с PyTorch сегодня
Следуйте инструкциям на pytorch.org для установки на выбранную платформу (скоро появится поддержка Windows). PyTorch поддерживает Python 2 и 3 и вычисления на процессорах или графических процессорах NVIDIA с использованием CUDA 7.5 или 8.0 и CUDNN 5.1 или 6.0. Бинарные файлы Linux для conda и pip включают даже сам CUDA, поэтому вам не нужно настраивать его самостоятельно.
Официальные учебные пособия включают 60-минутное введение и пошаговое руководство по Deep Q-Learning, современной модели обучения с подкреплением.Существует также чудесно исчерпывающий учебник от Джастина Джонсона из Стэнфорда, а официальные примеры включают, среди прочего, глубокую сверточную генеративную состязательную сеть (DCGAN) и модели для ImageNet и нейронного машинного перевода. Ричи Нг из Национального университета Сингапура ведет актуальный список других реализаций PyTorch, примеров и руководств. Разработчики PyTorch и сообщество пользователей в любое время отвечают на вопросы на дискуссионном форуме, хотя вам, вероятно, сначала следует проверить документацию по API.
Несмотря на то, что PyTorch был доступен только в течение короткого времени, в трех исследовательских работах он уже использовался, несколько академических и промышленных лабораторий приняли его. Раньше, когда графы динамических вычислений были более неясными, мои коллеги и я из Salesforce Research считали Шайнер своим секретным соусом; Теперь мы рады, что PyTorch делает этот уровень мощности и гибкости массовым при поддержке крупных компаний. Удачного взлома!
Построение таблицы синтаксического анализа LL (1)
Предварительное условие - классификация синтаксических анализаторов сверху вниз, ПЕРВЫЙ набор, набор СЛЕДУЮЩИЙ
Нисходящий синтаксический анализатор строит дерево синтаксического анализа сверху вниз, начиная с нетерминального начала.Существует два типа парсеров сверху вниз:
- Парсер сверху вниз с отслеживанием
- синтаксические анализаторы сверху вниз без возврата
Парсеры сверху вниз без возврата можно разделить на две части:
В этой статье мы собираемся чтобы обсудить нерекурсивный спуск, который также известен как LL (1) Parser.
LL (1) Разбор:
Здесь 1-й L представляет, что сканирование входа будет выполняться слева направо, а второй L показывает, что в этом методе анализа мы будем использовать крайнее левое положение. Дерево деривации.и, наконец, 1 представляет количество упреждений, означает, сколько символов вы увидите, когда захотите принять решение.Построение таблицы синтаксического анализа LL (1):
Для построения таблицы синтаксического анализа у нас есть две функции:1: First (): Если есть переменная, и из этой переменной, если мы попытаемся проложите все струны, то начало Terminal Symbol называется первым.
2: Follow (): Что такое терминальный символ , который следует за переменной в процессе деривации.
Теперь, после вычисления первого и последующего набора для каждого нетерминального символа , мы должны построить таблицу синтаксического анализа. В таблице строки будут содержать нетерминалы, а столбец будет содержать символы терминала.
Все Null Productions Grammars будут находиться под элементами Follow, а остальные продукты будут находиться под элементами First set.Теперь давайте разберемся на примере.
Пример-1:
Рассмотрим грамматику:E -> TE ' E '-> + TE' | е Т -> ФТ ' T '-> * FT' | е F -> id | (E) ** e обозначает эпсилонНайдите их первый и последующие наборы:
Первый Следуйте E -> TE ' {id, (} ,)} E '-> + TE' / e {+, e} {$,)} T -> FT ' {id, (} {+, $,)} T '-> * FT' / e {*, e} {+, $,)} F -> id / (E) {id, (} {*, +, $,)} Теперь таблица синтаксического анализа LL (1):
id + * ( ) $ E E -> TE ' E -> TE' E ' E' -> + TE ' E' -> e E '-> e T T -> FT' T - > FT ' T' T '-> e T' -> * FT T '-> e T' -> e F F -> id F -> (E) Как вы можете видеть, все пустые продукты помещаются под следующий набор этого символа, а все остальные продукты лежат под первый из этого символа.
Примечание: Не все грамматики подходят для таблицы синтаксического анализа LL (1). Возможно, что одна ячейка может содержать более одной продукции.
Давайте посмотрим на примере.
Пример-2:
Рассмотрим грамматикуS -> A | а A -> aНайдите их первый и последующие наборы:
Первый Следуйте S -> A / a {a} {$} {$} 9045 A -> a {a} {$} Таблица синтаксического анализа:
2 9045 9045 > A, S -> a a $ A A -> a Здесь мы видим, что в одной ячейке находятся два производства.Следовательно, эта грамматика невозможна для LL (1) Parser.
Вниманию читателя! Не прекращайте учиться сейчас. Получите все важные концепции теории CS для собеседований SDE с помощью курса CS Theory Course по приемлемой для студентов цене и будьте готовы к отрасли.
chempy.util.parsing - документация chempy 0.4.1
# - * - кодировка: utf-8 - * - "" "Функции для химических формул и реакций" "" из __future__ import (absolute_import, Division, print_function) из коллекций импортировать defaultdict импорт ре предупреждения об импорте из .pyutil import ChemPyDeprecationWarning, memoize parsing_library = 'pyparsing' # информация, используемая для выборочного тестирования. @memoize () def _get_formula_parser (): "" "Создать синтаксический анализатор прямого синтаксического анализа для химических формул БНФ для простой химической формулы (без вложенности) целое число :: '0' .. '9' + element :: 'A' .. 'Z' 'a' .. 'z' * термин :: элемент [целое число] формула :: срок + BNF для вложенной химической формулы целое число :: '0' .. '9' + element :: 'A' .. 'Z' 'a' .. 'z' * термин :: (элемент | '(' формула ')') [целое число] формула :: срок + Ноты ----- Код в этой функции взят из ответа на StackOverflow: http: // stackoverflow.com / a / 18555142/7написано: Пол Макгуайр, http://stackoverflow.com/users/165216/paul-mcguire в ответ на вопрос, сформулированный: Thales MG, http://stackoverflow.com/users/2708711/thales-mg код находится под лицензией CC-WIKI. (см .: http://blog.stackoverflow.com/2009/06/attribution-required/) "" " _p = __import __ (анализ_библиотеки) Вперед, Группа, OneOrMore = _p.Forward, _p.Group, _p.OneOrMore Необязательно, ParseResults, Regex = _p.Необязательно, _p.ParseResults, _p.Regex Подавить, Word, nums = _p.Suppress, _p.Word, _p.nums LPAR, RPAR = map (Подавить, "()") целое число = Слово (числа) # добавить действие синтаксического анализа для преобразования целых чисел в целые числа для поддержки выполнения сложения # и умножение во время синтаксического анализа integer.setParseAction (лямбда t: int (t [0])) # element = Word (alphas.upper (), alphas.lower ()) # или, если вы хотите быть более конкретным, используйте это Regex element = Regex ( r "A [cglmrstu] | B [aehikr]? | C [adeflmorsu]? | D [bsy] | E [rsu] | F [emr]? |" "G [ade] | H [efgos]? | I [nr]? | Kr? | L [airu] | M [dgnot] | N [abdeiop]? |" "Os? | P [abdmortu]? | R [abefghnu] | S [bcegimnr]? | T [abcehilm] |" "Uu [bhopqst] | U | V | W | Xe | Yb? | Z [nr]") # вперед объявить 'формулу', чтобы ее можно было использовать в определении 'термина' формула = Вперед () термин = Группа ((элемент | Группа (LPAR + формула + RPAR) («подгруппа»)) + Необязательно (целое число, по умолчанию = 1) ("mult")) # определяем содержимое формулы как одно или несколько терминов формула << OneOrMore (термин) # добавить действия синтаксического анализа для обработки во время синтаксического анализа # действие синтаксического анализа для умножения подгрупп def multiplyContents (токены): t = токены [0] # если эти токены содержат подгруппу, то используйте множитель для # увеличить количество всех элементов в подгруппе если т.подгруппа: mult = t.mult за срок в t.подгруппе: термин [1] * = mult вернуть t.subgroup term.setParseAction (multiplyContents) # добавить действие синтаксического анализа, чтобы суммировать несколько ссылок на один и тот же элемент def sumByElement (токены): elementsList = [t [0] для t в токенах] # конструкция установлена, чтобы увидеть, есть ли дубликаты дубликаты = len (elementsList)> len (set (elementsList)) # если есть повторяющиеся имена элементов, просуммируйте по элементам и # возвращаем новый вложенный ParseResults если дубликаты: ctr = defaultdict (число) для t в токенах: ctr [t [0]] + = t [1] вернуть ParseResults ([ParseResults ([k, v]) для k, v в ctr.Предметы()]) formula.setParseAction (sumByElement) формула возврата символы = ( 'H', 'He', 'Li', 'Be', 'B', 'C', 'N', 'O', 'F', 'Ne', 'Na', 'Mg', 'Al ', Si, P, S, Cl, Ar, K, Ca, Sc, Ti, V, Cr, Mn, Fe ', Co, Ni, Cu, Zn, Ga, Ge, As, Se, Br, Kr, Rb, Sr, Y, Zr, Nb, Mo, Tc, Ru, Rh, Pd, Ag, Cd, In, Sn, Sb, Te, I, Xe, Cs, Ba, La, Ce, Pr, Nd, Pm, Sm, Eu, Gd, Tb, Dy, Ho, Er, Tm, Yb, Lu, Hf, Ta, W, Re, Os, Ir, Pt, Au, Hg, Tl, Pb, Bi, Po, At, Rn, Fr, Ra, Ac, Th, Pa, U, Np, Pu, Am, Cm, Bk, Cf, 'Es', 'Fm', 'Md', 'Нет', 'Lr', 'Rf', 'Db', 'Sg', 'Bh', 'Hs', 'Mt', 'Ds', Rg, Cn, Uut, Fl, Uup, Lv, Uus, Uuo ) имена = ( «Водород», «Гелий», «Литий», «Бериллий», «Бор», «Углерод», «Азот», «Кислород», «Фтор», «Неон», «Натрий», «Магний», «Алюминий», «Кремний», «Фосфор», «Сера», «Хлор», «Аргон», «Калий», «Кальций», «Скандий», «Титан», Ванадий, Хром, Марганец, Железо, Кобальт, Никель, «Медь», «Цинк», «Галлий», «Германий», «Мышьяк», «Селен», «Бром», «Криптон», «Рубидий», «Стронций», «Иттрий», «Цирконий», «Ниобий», «Молибден», «Технеций», «Рутений», «Родий», «Палладий», «Серебро», «Кадмий», «Индий», «Олово», «Сурьма», «Теллур», «Йод», «Ксенон», «Цезий», «Барий», «Лантан», «Церий», «Празеодим», «Неодим», «Прометий», «Самарий», Европий, Гадолиний, Тербий, Диспрозий, Гольмий, «Эрбий», «Тулий», «Иттербий», «Лютеций», «Гафний», «Тантал», «Вольфрам», «Рений», «Осмий», «Иридий», «Платина», «Золото», «Меркурий», «Таллий», «Свинец», «Висмут», «Полоний», «Астатин», Радон, Франций, Радий, Актиний, Торий, Протактиний, «Уран», «нептуний», «плутоний», «америций», «кюрий», «Берклий», «Калифорниум», «Эйнштейний», «Фермий», «Менделевий», «Нобелиум», «Лоуренсий», «Резерфордиум», «Дубний», «Сиборгий», 'Бориум', 'Калий', 'Мейтнерий', 'Дармштадтий', 'Рентгениум', 'Copernicium', '(Унунтриум)', 'Флеровий', '(Унунпентиум)', 'Livermorium', '(Ununseptium)', '(Ununoctium)' ) lower_names = кортеж (сущ.lower (). lstrip ('('). rstrip (')') для n в именах) [документы] def atomic_number (имя): пытаться: вернуть символы. index (имя) + 1 кроме ValueError: вернуть lower_names.index (name.lower ()) + 1 # Данные в '_relative_atomic_masses' находятся под лицензией CC-SA # https://en.wikipedia.org/w/index.php?title=List_of_elements&oldid=700476748 _relative_atomic_masses = ( "1,008 4,002602 (2) 6,94 9,0121831 (5) 10,81 12,011 14,007 15,999" "18.998403163 (6) 20.1797 (6) 22.98976928 (2) 24.305 26,9815385 (7) 28,085 " "30.973761998 (5) 32.06 35.45 39.948 (1) 39.0983 (1) 40.078 (4)" «44,955908 (5) 47,867 (1) 50,9415 (1) 51,9961 (6) 54,938044 (3) 55,845 (2)» «58,933194 (4) 58,6934 (4) 63,546 (3) 65,38 (2) 69,723 (1) 72,630 (8)» "74,921595 (6) 78,971 (8) 79,904 83,798 (2) 85,4678 (3) 87,62 (1)" "88,(2) 91,224 (2) 92, (2) 95,95 (1) [98] 101,07 (2) 102,(2)» «106,42 (1) 107,8682 (2) 112,414 (4) 114,818 (1) 118,710 (7) 121,760 (1)» «127,60 (3) 126, (3) 131,293 (6) 132,196 (6) 137.327 (7) " "138. (7) 140.116 (1) 140.
(2) 144.242 (3) [145] 150,36 (2)» «151,964 (1) 157,25 (3) 158,92535 (2) 162,500 (1) 164,93033 (2) 167,259 (3)» «168,93422 (2) 173,045 (10) 174,9668 (1) 178,49 (2) 180,94788 (2) 183,84 (1)» «186,207 (1) 190,23 (3) 192,217 (3) 195,084 (9) 196,966569 (5) 200,592 (3)» «204,38 207,2 (1) 208,98040 (1) [209] [210] [222] [223] [226] [227]» "232.0377 (4) 231.03588 (2) 238.02891 (3) [237] [244] [243] [247] [247]" «[251] [252] [257] [258] [259] [266] [267] [268] [269] [270] [269]» «[278] [281] [282] [285] [286] [289] [289] [293] [294] [294]» ) def _get_relative_atomic_masses (): для массы в _relative_atomic_masses.расколоть(): если mass.startswith ('[') и mass.endswith (']'): доходность с плавающей запятой (масса [1: -1]) elif '(' в массе: yield float (mass.split ('(') [0]) еще: доходность (плавающая (масса)) relative_atomic_masses = кортеж (_get_relative_atomic_masses ()) [документы] def mass_from_composition (композиция): "" "Вычисляет молекулярную массу на основе атомных масс. Параметры ---------- состав: дикт Сопоставление словаря int (атомный номер) с int (коэффициент) Возврат ------- плавать молекулярная масса в единицах атомной массы Ноты ----- Атомный номер 0 обозначает заряд или «чистую электронную недостаточность». Примеры -------- >>> '%.2f '% mass_from_composition ({0: -1, 1: 1, 8: 1}) '17 .01 ' "" " масса = 0,0 для k, v в композиции.items (): если k == 0: # электрон масса - = v * 5.489e-4 еще: масса + = v * относительная_атомная_масса [k-1] возвратная масса def _get_charge (chgstr): если chgstr == '+': возврат 1 elif chgstr == '-': возврат -1 для токена, анти, войдите в zip ('+ -', '- +', (1, -1)): если токен в chgstr: если анти в chgstr: Raise ValueError ("Недопустимое описание платежа (+ & - присутствует)") до, после = chgstr.сплит (токен) если len (до)> 0 и len (после)> 0: Raise ValueError ("Значения до и после токена начисления") если len (до)> 0: # will_be_missing_in = '0.5.0' warnings.warn ("'Fe / 3 +' устарел, используйте, например, 'Fe + 3'", ChemPyDeprecationWarning, stacklevel = 3) знак возврата * int (1 if before == '' else before) если len (после)> 0: знак возврата * int (1 если после == '' иначе после) Raise ValueError ("Недопустимое описание начисления (+ или - отсутствует)") def _formula_to_parts (формула, префиксы, суффиксы): # Удалять префиксы и суффиксы drop_pref, drop_suff = [], [] для ign в префиксах: если формула.начинается с (ign): drop_pref.append (ign) формула = формула [len (ign):] для ign в суффиксах: если формула.endswith (ign): drop_suff.append (ign) формула = формула [: - len (ign)] # Выписка заряда если '/' в формуле: # will_be_missing_in = '0.5.0' warnings.warn ("/ depr. (до 0.5.0): используйте 'Fe + 3' вместо 'Fe / 3 +'", ChemPyDeprecationWarning, stacklevel = 3) части = формула.split ('/') если '+' в частях [0] или '-' в частях [0]: Raise ValueError ("Плата должна быть разделена знаком /") если parts [1] не равен None: wo_pm = части [1].replace ('+', '') .replace ('-', '') если wo_pm! = '', а не str.isdigit (wo_pm): Raise ValueError ("Спецификатор начисления, не являющийся цифрой") если len (parts)> 2: Raise ValueError ("В формуле допускается не более одного '/'") еще: для токена в '+ -': если токен в формуле: если formula.count (token)> 1: Raise ValueError ("Несколько токенов:% s"% токен) parts = formula.split (токен) части [1] = токен + части [1] перерыв еще: parts = [формула, Нет] вернуть части + [кортеж (drop_pref), кортеж (drop_suff [:: - 1])] def _parse_stoich (стоич): if stoich == 'e': # особый случай, электрон не является элементом возвращаться {} return {символы.index (k) +1: n для k, n в _get_formula_parser (). parseString (stoich)} _greek_letters = ( 'альфа', 'бета', 'гамма', 'дельта', 'эпсилон', 'дзета', 'эта', 'тета', 'йота', 'каппа', 'лямбда', 'му', 'ню', 'xi', 'omicron', 'пи', 'ро', «сигма», «тау», «ипсилон», «фи», «чи», «пси», «омега» ) _greek_u = u'αβγδεζηθικλμνξοπρστυφχψω ' _latex_mapping = {k + '-': '\\' + k + '-' для k в _greek_letters} _latex_mapping ['epsilon-'] = '\\ varepsilon-' _latex_mapping ['omicron-'] = 'o-' _latex_mapping ['.\ d + ', s) если len (m) == 0: m = 1 elif len (m) == 1: s = s [len (m [0]):] m = int (m [0]) еще: Raise ValueError ("Не удалось проанализировать:% s"% s) вернуть м, с [docs] def formula_to_composition (формула, префиксы = Нет, суффиксы = ('(s)', '(l)', '(g)', '(aq)')): "" "Разобрать состав формулы, представляющей химическую формулу Композиция представлена как dict-отображение int -> int (атомарное число -> кратность). «Атомный номер» 0 представляет собой чистый заряд.Параметры ---------- формула: str Химическая формула, например 'h3O', 'Fe + 3', 'Cl-' префиксы: повторяющиеся строки Префиксы, которые следует игнорировать, например ('.', 'альфа-') суффиксы: кортеж строк Суффиксы, которые следует игнорировать, например ('(g)', '(s)') Примеры -------- >>> formula_to_composition ('Nh5 +') == {0: 1, 1: 4, 7: 1} Правда >>> formula_to_composition ('. NHO- (aq)') == {0: -1, 1: 1, 7: 1, 8: 1} Правда >>> formula_to_composition ('Na2CO3.7h3O ') == {11: 2, 6: 1, 8: 10, 1: 14} Правда "" " если префиксы отсутствуют: prefixes = _latex_mapping.keys () stoich_tok, chg_tok = _formula_to_parts (формула, префиксы, суффиксы) [: 2] tot_comp = {} parts = stoich_tok.split ('.') для idx, stoich в перечислении (части): если idx == 0: m = 1 еще: м, stoich = _get_leading_integer (stoich) comp = _parse_stoich (стоич) для k, v в comp.items (): если k не в tot_comp: tot_comp [k] = m * v еще: tot_comp [k] + = m * v если chg_tok не равно None: tot_comp [0] = _get_charge (chg_tok) вернуть tot_comp def _subs (строка, шаблоны): для патта, отвечайте в шаблонах.Предметы(): строка = строка.replace (патт, ответ) строка возврата def _parse_multiplicity (строки, entity_keys = None): "" " Примеры -------- >>> _parse_multiplicity (['2 h3O2', 'O2']) == {'h3O2': 2, 'O2': 1} Правда >>> _parse_multiplicity (['2 * h3O2', 'O2']) == {'h3O2': 2, 'O2': 1} Правда >>> _parse_multiplicity (['']) == {} Правда "" " результат = {} для элементов в [re.split ('\ * |', s) для s в строках]: если len (items) == 1: если items [0] == '': Продолжать результат [items [0]] = 1 elif len (элементы) == 2: результат [items [1]] = int (items [0]) еще: поднять ValueError ("Ко многим частям в подстроке") если entity_keys не равно None: для k в результате: если k не находится в файле entity_keys: Raise ValueError ("Неизвестный ключ_содержания:% s"% k) вернуть результат [документы] def to_reaction (строка, ключи_содержания, токен, Cls, globals_ = None, ** kwargs): "" "Разбирает строку на объект реакции и вещества Reac1 + 2 Reac2 + (2 Reac1) -> Prod1 + Prod2; 10 ** 3.7; ref = 'doi: 12 / ab' Reac1 = Prod1; 2.1; Параметры ---------- строка: str строковое представление для анализа entity_keys: итерация строк Допустимые имена, например ('h3O', 'H +', 'OH-') токен: str маркер-разделитель между реагентом и стороной продукта Cls: класс например подкласс Реакции globals_: dict (необязательно) Глобальные переменные передаются в: func: `eval`, когда` `None``: chempy.units.default_units используется с chempy и дополнительные записи default_units.Ноты ----- Эта функция вызывает: func: `eval`, поэтому есть серьезные проблемы с безопасностью. с запуском этого на ненадежных данных. "" " # TODO: добавить обработку юнитов. если globals_ равно None: импортный чемпи из ставок на импорт chempy.kinetics из chempy.units import default_units globals_ = {k: getattr (ставки, k) для k в каталоге (ставки)} globals_.update ({'chempy': chempy, 'default_units': default_units}) если default_units не равен None: globals_.обновление (default_units.as_dict ()) пытаться: stoich, param, kw = map (str.strip, line.rstrip ('\ n'). split (';')) кроме ValueError: если ';' в соответствии: stoich, param = map (str.strip, line.rstrip ('\ n'). split (';')) еще: stoich, param = line.strip (), kwargs.pop ('параметр', 'Нет') еще: kwargs.update ({} если globals_ равно False else eval ('dict (' + kw + ')', globals_)) если isinstance (param, str): param = None, если globals_ имеет значение False else eval (param, globals_) если жетон не в стейч: Raise ValueError ("Отсутствующий токен:% s"% токен) reac_prod = [[y.strip () для y в x.split ('+')] для x в stoich.split (токен)] act, inact = [], [] для стороны в reac_prod: если сторона [-1] .startswith ('('): если не сторона [-1] .endswith (')'): Raise ValueError ("Неверный формат (отсутствует закрывающая скобка)") inact.append (_parse_multiplicity (сторона [-1] [1: -1] .split ('+'), субстанция_ключи)) act.append (_parse_multiplicity (сторона [: - 1], entity_keys)) еще: в действии.добавить ({}) act.append (_parse_multiplicity (сторона, ключи_содержания)) # stoich coeff -> dict return Cls (act [0], act [1], param, inact_reac = inact [0], inact_prod = inact [1], ** kwargs) def _formula_to_format (sub, sup, formula, prefixes = None, инфиксы = Нет, суффиксы = ('(s)', '(l)', '(g)', '(aq)')): parts = _formula_to_parts (формула, prefixes.keys (), суффиксы) stoichs = части [0] .split ('.') строка = '' для idx, stoich in enumerate (stoichs): если idx == 0: m = 1 еще: м, stoich = _get_leading_integer (stoich) строка + = _subs ('.', инфиксы) если m! = 1: строка + = str (m) строка + = re.sub (r '([0-9] +)', лямбда m: sub (m.group (1)), stoich) если parts [1] не равен None: chg = _get_charge (части [1]) если chg <0: token = '-' if chg == -1 else '% d-'% -chg если chg> 0: token = '+' if chg == 1 else '% d +'% chg строка + = sup (токен) если len (частей)> 4: Raise ValueError ("Неверная формула") pre_str = '' .join (map (lambda x: _subs (x, prefixes), parts [2])) вернуть pre_str + string + ''.присоединиться (части [3]) [документы] def formula_to_latex (формула, префиксы = None, infixes = None, ** kwargs): r "" "Преобразовать строку формулы в латексное представление Параметры ---------- формула: str Химическая формула, например 'h3O', 'Fe + 3', 'Cl-' префиксы: dict Префиксные преобразования, по умолчанию: греческие буквы и. инфиксы: dict Инфиксные преобразования, по умолчанию:. {2 +} (водн.)' >>> formula_to_latex ('.{% s} '% str (int (суффикс)) + единица измерения еще: вернуть s + unit _unicode_sub = {} для k, v в перечислении (u "₀₁₂₃₄₅₆₇₈₉"): _unicode_sub [str (k)] = v _unicode_sup = { '+': u'⁺ ', '-': u'⁻ ', } для k, v в перечислении (u "⁰¹²³⁴⁵⁶⁷⁸⁹"): _unicode_sup [str (k)] = v [документы] def formula_to_unicode (формула, префиксы = None, infixes = None, ** kwargs): u "" "Преобразование строки формулы в строковое представление Unicode Параметры ---------- формула: str Химическая формула, например 'h3O', 'Fe + 3', 'Cl-' префиксы: dict Префиксные преобразования, по умолчанию: греческие буквы и.инфиксы: dict Инфиксные преобразования, по умолчанию:. суффиксы: кортеж строк Суффиксы, которые нужно сохранить, например ('(g)', '(s)') Примеры -------- >>> formula_to_unicode ('Nh5 +') == u'NH₄⁺ ' Правда >>> formula_to_unicode ('Fe (CN) 6 + 2') == u'Fe (CN) ₆²⁺ ' Правда >>> formula_to_unicode ('Fe (CN) 6 + 2 (aq)') == u'Fe (CN) ₆²⁺ (aq) ' Правда >>> formula_to_unicode ('. NHO- (aq)') == u'⋅NHO⁻ (aq) ' Правда >>> formula_to_unicode ('альфа-FeOOH (s)') == u'α-FeOOH (s) ' Правда "" " если префиксы отсутствуют: prefixes = _unicode_mapping если инфиксы None: infixes = _unicode_infix_mapping вернуть _formula_to_format ( лямбда x: ''.присоединиться (_unicode_sub [str (_)] для _ в x), лямбда x: '' .join (_unicode_sup [str (_)] для _ в x), формулы, префиксы, инфиксы, ** kwargs) [документы] def number_to_scientific_unicode (number, fmt = '%. 3g'): u "" " Примеры -------- >>> number_to_scientific_unicode (3.14) == u'3.14 ' Правда >>> number_to_scientific_unicode (3.14159265e-7) == u'3.14 · 10⁻⁷ ' Правда >>> Импортировать количества как pq >>> number_to_scientific_html (2 ** 0,5 * pq.m / pq.s) '1,41 м / с' "" " пытаться: unit = '' + number.dimensionality.unicode число = число. величина кроме AttributeError: unit = '' s = fmt% номер если 'e' в s: префикс, суффикс = s.split ('e') префикс возврата + u '· 10' + u ''. join (map (_unicode_sup.get, str (int (суффикс)))) + unit еще: вернуть s + unit [документы] def formula_to_html (формула, префиксы = None, infixes = None, ** kwargs): u "" "Преобразовать строку формулы в строковое представление html Параметры ---------- формула: str Химическая формула, e.грамм. 'h3O', 'Fe + 3', 'Cl-' префиксы: dict Префиксные преобразования, по умолчанию: греческие буквы и. инфиксы: dict Инфиксные преобразования, по умолчанию:. суффиксы: кортеж строк Суффиксы, которые нужно сохранить, например ('(g)', '(s)') Примеры -------- >>> formula_to_html ('Nh5 +') 'NH 4 + ' >>> formula_to_html ('Fe (CN) 6 + 2') 'Fe (CN) 6 2+ ' >>> formula_to_html ('Fe (CN) 6 + 2 (водн.)') 'Fe (CN) 6 2+ (водн.)' >>> formula_to_html ('.NHO- (водн.) ') '& sdot; NHO - (водн.)' >>> formula_to_html ('альфа-FeOOH (s)') '& alpha; -FeOOH (s)' "" " если префиксы отсутствуют: префиксы = _html_mapping если инфиксы None: infixes = _html_infix_mapping return _formula_to_format (lambda x: '% s '% x, лямбда x: '% s '% x, формулы, префиксы, инфиксы, ** kwargs) [docs] def number_to_scientific_html (number, fmt = '%.3g '): "" " Примеры -------- >>> number_to_scientific_html (3.14) == '3.14' Правда >>> number_to_scientific_html (3.14159265e-7) '3.14 & sdot; 10 -7 ' >>> Импортировать количества как pq >>> number_to_scientific_html (2 ** 0,5 * pq.m / pq.s) '1,41 м / с' "" " пытаться: unit = '' + str (число.