What does npm exec do? What is the difference between «npm exec» and «npx»?
What are the building blocks of OWL ontologies?
Learn more about «RDF star», «SPARQL star», «Turtle star», «JSON-LD star», «Linked Data star», and «Semantic Web star».
The Hadamard gate is one of the simplest quantum gates which acts on a single qubit.
Learn more about the bra–ket notation.
Progressive Cactus is an evolution of the Cactus multiple genome alignment protocol that uses the progressive alignment strategy.
The Human Genome Project is an ambitious project which is still underway.
What are SVMs (support vector machines)?
Find out more in Eckher’s article about TensorFlow.js and linear regression.
On the importance of centralised metadata registries at companies like Uber.
Facebook’s Nemo is a new custom-built platform for internal data discovery. Learn more about Facebook’s Nemo.
What is Data Commons (datacommons.org)? Read Eckher’s introduction to Data Commons (datacommons.org) to learn more about the open knowledge graph built from thousands of public datasets.
Learn more about how Bayer uses semantic web technologies for corporate asset management and why it enables the FAIR data in the corporate environment.
An introduction to WikiPathways by Eckher is an overview of the collaboratively edited structured biological pathway database that discusses the history of the project, applications of the open dataset, and ways to access the data programmatically.
Eckher’s article about question answering explains how question answering helps extract information from unstructured data and why it will become a go-to NLP technology for the enterprise.
Read more about how document understanding AI works, what its industry use cases are, and which cloud providers offer this technology as a service.
Lexemes are Wikidata’s new type of entity used for storing lexicographical information. The article explains the structure of Wikidata lexemes and ways to access the data, and discusses the applications of the linked lexicographical dataset.
The guide to exploring linked COVID-19 datasets describes the existing RDF data sources and ways to query them using SPARQL. Such linked data sources are easy to interrogate and augment with external data, enabling more comprehensive analysis of the pandemic both in New Zealand and internationally.
The introduction to the Gene Ontology graph published by Eckher outlines the structure of the GO RDF model and shows how the GO graph can be queried using SPARQL.
The overview of the Nobel Prize dataset published by Eckher demonstrates the power of Linked Data and demonstrates how linked datasets can be queried using SPARQL. Use SPARQL federation to combine the Nobel Prize dataset with DBPedia.
Learn why federated queries are an incredibly useful feature of SPARQL.
What are the best online Arabic dictionaries?
How to pronounce numbers in Arabic?
List of months in Maori.
Days of the week in Maori.
The list of country names in Tongan.
The list of IPA symbols.
What are the named entities?
What is computational linguistics?
Learn how to use the built-in React hooks.
Learn how to use language codes in HTML.
Learn about SSML.
Browse the list of useful UX resources from Google.
Where to find the emoji SVG sources?.
What is Wikidata?
What’s the correct markup for multilingual websites?
How to use custom JSX/HTML attributes in TypeScript?
Learn more about event-driven architecture.
Where to find the list of all emojis?
How to embed YouTube into Markdown?
What is the Google Knowledge Graph?
Learn SPARQL.
Explore the list of coronavirus (COVID-19) resources for bioinformaticians and data science researchers.
Sequence logos visualize protein and nucleic acid motifs and patterns identified through multiple sequence alignment. They are commonly used widely to represent transcription factor binding sites and other conserved DNA and RNA sequences. Protein sequence logos are also useful for illustrating various biological properties of proteins. Create a sequence logo with Sequence Logo. Paste your multiple sequence alignment and the sequence logo is generated automatically. Use the sequence logo maker to easily create vector sequence logo graphs. Please refer to the Sequence Logo manual for the sequence logo parameters and configuration. Sequence Logo supports multiple color schemes and download formats.
Sequence Logo is a web-based sequence logo generator. Sequence Logo generates sequence logo diagrams for proteins and nucleic acids. Sequence logos represent patterns found within multiple sequence alignments. They consist of stacks of letters, each representing a position in the sequence alignment. Sequence Logo analyzes the sequence data inside the user’s web browser and does not store or transmit the alignment data via servers.
Te Reo Maps is an online interactive Maori mapping service. All labels in Te Reo Maps are in Maori, making it the first interactive Maori map. Te Reo Maps is the world map, with all countries and territories translated into Maori. Please refer to the list of countries in Maori for the Maori translations of country names. The list includes all UN members and sovereign territories.
Phonetically is a web-based text-to-IPA transformer. Phonetically uses machine learning to predict the pronunciation of English words and transcribes them using IPA.
Punycode.org is a tool for converting Unicode-based internationalized domain names to ASCII-based Punycode encodings. Use punycode.org to quickly convert Unicode to Punycode and vice versa. Internationalized domains names are a new web standard that allows using non-ASCII characters in web domain names.
My Sequences is an online platform for storing and analyzing personal sequence data. My Sequences allows you to upload your genome sequences and discover insights and patterns in your own DNA.
Словообразовательный словарь «Морфема» дает представление о морфемной структуре слов русского языка и слов современной лексики. Для словообразовательного анализа представлены наиболее употребительные слова современного русского языка, их производные и словоформы. Словарь предназначен школьникам, студентам и преподавателям. Статья разбора слова «сладкоежка» по составу показывает, что это слово имеет два корня, соединительную гласную, суффикс и окончание. На странице также приведены слова, содержащие те же морфемы. Словарь «Морфема» включает в себя не только те слова, состав которых анализируется в процессе изучения предмета, но и множество других слов современного русского языка. Словарь адресован всем, кто хочет лучше понять структуру русского языка.
Разбор слова «кормушка» по составу.
Разбор слова «светить» по составу.
Разбор слова «сбоку» по составу.
Разбор слова «шиповник» по составу.
Разбор слова «народ» по составу.
Разбор слова «впервые» по составу.
Разбор слова «свежесть» по составу.
Разбор слова «издалека» по составу.
Разбор слова «лесной» по составу.
Какое окончание в слове «самолет»?
Вовлечь — глагол в форме инфинитива (начальной форме). Окончание в слове выделяется по-разному. Для школьников оно нулевое. А вот студенты-филологи должны знать, что Ч здесь происходит от *kt и что в праславянском языке конец слова звучал как *-kti, где *-ti — тот самый суффикс -ти, который в других глаголах сохранился (везти, вести, плести и др.). То есть, получается, что окончание присутствует в звуке [ч] — оно накладывается на предыдущую морфему.
Корень слова -влеч-, а однокоренные слова как раз открывают нам ту самую к (*k), которая потом «скрылась» в ч: вовлеку, привлекать, влечение и др.
Приставка во-.
Основа слова вовлечь- (без окончания).
Очень интересный вопрос, необычный. Но все же попробую.
- модератор
- администратор
- сепаратор
- вибратор
- комментатор
- провокатор
- диктатор
- экспериментатор
- статор
- оператор
- оратор
- генератор
- прокуратор
- император
- литератор
- инкубатор
- куратор
- дефлоратор
Слово «журавль». Журавль — это птица, имя существительное — он — род мужской. В единственном числе — журавль, а если их много, то во множественном числе будет — журавли. Уже на этом примере видно, что окончание в слове изменилось — стало «и». И при склонении у слова «журавль» окончания меняются — нет журавля — окончание «я», о журавле — окончание «е».
А вот в слове «журавль», которое заканчивается на мягкий знак, окончание будет нулевое.
Это птица, относится к семейству Фламинговых.Слово фламинго — имя существительное в среднем роде, является корнем слова.В единственном и во множественном числе не меняет окончание.Фламинго является и основой.Окончания не имеет.Добавлю ещё немного слов к теи, что уже перечислены:
зубильный
лабильный
рубильный
дробильный
стабильный
иммобильный
теребильный
нестабильный
локомобильный
преизобильный
словообильный
травообильный
метастабильный
новоизобильный
термолабильный
угледробильный
зернодробильный
льнотеребильный
термостабильный
Дробильная машина Самолет дробилка
Дробилка — Википедия
Валковая дробилка Мобильная дробильная установка Дроби́лка — оборудование для дробления,то есть механического воздействия на твёрдые материалы с целью их разрушения [1] .
Купить Конусная Дробилка Машина Pyb1750 оптом из Китая
Лучший сервис гидравлическая руда дробильная машина pyb900 конусная дробилка для карьера 50 000,00 $-100 000,00 $ / компл.Купить Дробилка Для Соли оптом из Китая,Большая емкость каменной соли Дробильная Машина Молотковая Дробилка для продажи Турция 14 000,00 $-16 000,00 $ / компл.мобильные дробилки в китае,мобильный дробилка с экрана. части дробилки сетка экрана дробилки Машина дробилки сделано в Китае LM Heavy Industry is a manufacturers of jaw Crusher cone Crusher sand making machine vsi impact crusher mobile crusher plant and vertical mill ultrafine grinding tricyclic mediumspeed microgrinding coarse powder
дробильная машина YouTube
Dec 03, 2012· дробильная машина gandinichippers. Loading Unsubscribe from gandinichippers? Мобильная дробилка C1175 Duration: 2:06. gkos55 6,129 views.Каменная дробилка, Щековая дробилка, Конусная дробилка,Как ведущий мировой производитель дробильно-размольного оборудования, мы всегда предлагаем передовые и рациональные решения для любого размера дробления требований, в том числе карьера, добычи, переработкиугольная дробилка мм140,Молотковая дробилка. машина по дереву мини цена . о дробилка молотковая а1-дм2р-110 м . » общаться в чате 17 ноября 2012А1-ДМ2Р. 130х50 6 гк Сталь 30 ХГСА тв. hrc 50 молотковая дробилка А1-ДДР цены.
Кабельные дробилка MELİKŞAH MAKİNA
Кабельные дробилка; Машина для зачистки кабеля Мобильная дробильная кабельные системы 3 основные машины, и их оборудование представляет собой систему, состоящую из ¬.Дробилка — Википедия,Валковая дробилка Мобильная дробильная установка Дроби́лка — оборудование для дробления,то есть механического воздействия на твёрдые материалы с целью их разрушения [1] .Дробильная машина RAYCO RC 20XP YouTube,Mar 03, 2010· Woodturning Rough edge log into a vase !! hollowform 職人技】木工旋盤で丸太から壺を作る! Duration: 23:04. yamabiko1220 Recommended for you
Каменная дробилка, Щековая дробилка, Конусная дробилка
Как ведущий мировой производитель дробильно-размольного оборудования, мы всегда предлагаем передовые и рациональные решения для любого размера дробления требований, в том числе карьера, добычи, переработкиМобильная дробилка для бетона помогает вам получать,Мобильная дробилка для бетона от производителя . Мобильная дробилка для бетона это уникальная дробильная машина, которая включает три этапа дробления.мобильные дробилки в китае,мобильный дробилка с экрана. части дробилки сетка экрана дробилки Машина дробилки сделано в Китае LM Heavy Industry is a manufacturers of jaw Crusher cone Crusher sand making machine vsi impact crusher mobile crusher plant and vertical mill ultrafine grinding tricyclic mediumspeed microgrinding coarse powder
Щепорез в России сравнить цены или купить на PromPortal.su
Машина дробильная (дробилка, щепорез) МД-30/500 (500-900 кг/ч) Цену уточняйте Под заказсколько стоит купить камень дробильная машина дробилка ,совокупный камень дробильная установка. совокупный дробильная установка цена, совокупный дробильная,Дробильная установка / дробилка,дробилка цена 2015 Китай Мини . получить цену сколько стоит мобильная дробильнаяМобильная Дробилка, Передвижная Дробилка, Мобильная,Мобильная дробильная установка имеет преимущества удо.ой транспортировки, низкой стоимости на транспортировку, гибкой конфигурации, удо.ого обслуживания и др. Он может работать как независимая единица, или как
Конусная дробилка, флотационная машина, мобильная дробилка
Конусная дробилка, флотационная машина, мобильная дробилка, угольная мельница, шаровая мельница. Цена продажи мобильной щековой дробилки гранита ПП; Базальтовая конусная дробилка hp2 ценаКабельные дробилка MELİKŞAH MAKİNA,Кабельные дробилка; Машина для зачистки кабеля Мобильная дробильная кабельные системы 3 основные машины, и их оборудование представляет собой систему, состоящую из ¬.Дробилка и мельница для переработки горных руд.,Дробильная Машина; Мельничная Машина Щековая дробилка hj является важной частью горной техники, которая широко Вертикально-ударная Дробилка vsi.
дробильная машина цена завод в Индии,
угольный шлак дробильная машина угольный шлак дробильная машина Камнедробилка Цена Машины В Индии . завод в Индиимашина завод каменная дробилка в каPFT Усиленная Роторная дробилка,Эта машина использует удары чтобы раздавить сырье. Когда материал доходит до зоны действия молотка _ материал будет оттеснена на ударное устройство для дробления под действием силы воздействия то материал будеткаменная дробилка машина цена в махараштре,дробилка машина дробилка каменная . бокситы дробильная машина в Махараштре. kapchi цена каменная дробилка в нима семян б каменная дробилка оборудование в .
Дробилка — Википедия
Валковая дробилка Мобильная дробильная установка Как правило, дробилками называют механизмы, разрушающие материалы до крупности 5—6 мм.ES-S4002 филиал дробилка Электрический разрывная машина,Купите здесь и получите скидку до 70% : https://s.click.aliexpress/e/_dZCMHtT ES-S4002 филиал дробилкаДРОБИЛКА — разбор слова по составу (морфемный разбор),Разбор по составу слова ДРОБИЛКА: дроб/и/л/к/а. Подро.ый разбор, графическую схему и сходные по морфемному строению слова вы найдёте на сайте.
Молотковая дробилка — Википедия
Дробилка молотковая — механическая дробильная машина, применяемая для разрушения кусков, зёрен и частиц минерального сырья и аналогичных материалов, путём дробления породы ударами молотков, шарнирно закреплённыхдробильная машина YouTube,Dec 03, 2012· дробильная машина gandinichippers. Loading Unsubscribe from gandinichippers? Мобильная дробилка C1175 Duration: 2:06. gkos55 6,129 views.промышленные ситовые производители блочная и дробильная ,Дробильная установка в Мали Jul 13, 2017. Прочитайте больше Дробилка Для Вольфрама в Казахстане Jul 13, 2017. Прочитайте больше
Роторная дробилка — Википедия
Дробилка роторная — механическая дробильная машина с жестко закреплёнными рабочими деталями — билами (лопатками), предназначенная для дробления материалов малой крепкости путём массивного быстрого вращениякаменная дробилка машина цена в махараштре,дробилка машина дробилка каменная .
дробильная машина технические характеристики
Дробилка СМД117 стационарная дробильная машина. Дробилка щековая стационарная дробильная машина, производящая раздавливание горной породы двумя периодически сближающимися стальнымианглийская мобильная дробильная машина,Ударная дробильная машина для ударной дробилки для продажи ударная дробилка для камня цена . 2017 горячие продажи дробилки с . машина для . мобильная машинадробильная машина для строительно,Дробильная машина для кости дробильная машина для измельчения камня. Объявления дробилка для камня с ценами и фото, где купить по регионам предложения продам куплю .
Информация Каменная дробильная машина Добавить 0
Каменная дробильная машина crowne. портативный каменная дробилка машина для продажи. Различных размеров щековая дробилка, 2017 оптовая небольшой дробильная машина Запрос цены Предыдущая,,
описывающих слов — Найдите прилагательные для описания вещей
слов для описания ~ термин ~
Как вы, наверное, заметили, прилагательные к слову «термин» перечислены выше. Надеюсь, сгенерированный выше список слов для описания термина соответствует вашим потребностям.
Если вы получаете странные результаты, возможно, ваш запрос не совсем в правильном формате. В поле поиска должно быть простое слово или фраза, например «тигр» или «голубые глаза». Поиск слов, описывающих «людей с голубыми глазами», скорее всего, не даст результатов.Поэтому, если вы не получаете идеальных результатов, проверьте, не вводит ли ваш поисковый запрос «термин» в заблуждение таким образом.
Обратите также внимание на то, что если терминов-прилагательных не так много или их совсем нет, возможно, в вашем поисковом термине содержится значительная часть речи. Например, слово «синий» может быть как существительным, так и прилагательным. Это сбивает двигатель с толку, поэтому вы можете не встретить много прилагательных, описывающих его. Возможно, я исправлю это в будущем. Вам также может быть интересно: что за слово ~ термин ~?
Описание слов
Идея движка Describing Words возникла, когда я создавал движок для связанных слов (он похож на тезаурус, но дает вам гораздо более широкий набор связанных слов, а не просто синонимов).Играя с векторами слов и API «HasProperty» концептуальной сети, я немного повеселился, пытаясь найти прилагательные, которые обычно описывают слово. В конце концов я понял, что есть гораздо лучший способ сделать это: разбирать книги!
Project Gutenberg был первоначальным корпусом, но синтаксический анализатор стал более жадным и жадным, и в итоге я скармливал ему где-то около 100 гигабайт текстовых файлов — в основном художественной литературы, в том числе многих современных работ. Парсер просто просматривает каждую книгу и вытаскивает различные описания существительных.
Надеюсь, это больше, чем просто новинка, и некоторые люди действительно сочтут его полезным для написания и мозгового штурма, но стоит попробовать сравнить два существительных, которые похожи, но отличаются в некотором значении — например, интересен пол: «женщина» против «мужчины» и «мальчик» против «девочки». При первоначальном быстром анализе кажется, что авторы художественной литературы как минимум в 4 раза чаще описывают женщин (в отличие от мужчин), используя термины, связанные с красотой (в отношении их веса, черт лица и общей привлекательности).Фактически, «красивая», возможно, является наиболее широко используемым прилагательным для женщин во всей мировой литературе, что вполне согласуется с общим одномерным представлением женщин во многих других формах СМИ. Если кто-то хочет провести дальнейшее исследование по этому поводу, дайте мне знать, и я могу предоставить вам гораздо больше данных (например, существует около 25000 различных записей для слова «женщина» — слишком много, чтобы показать здесь).
Голубая окраска результатов отражает их относительную частоту. Вы можете навести курсор на элемент на секунду, и должна появиться оценка частоты.Сортировка по «уникальности» используется по умолчанию, и благодаря моему сложному алгоритму ™ она упорядочивает их по уникальности прилагательных к этому конкретному существительному относительно других существительных (на самом деле это довольно просто). Как и следовало ожидать, вы можете нажать кнопку «Сортировать по частоте использования», чтобы выбрать прилагательные по частоте их использования для этого существительного.
Особая благодарность разработчикам mongodb с открытым исходным кодом, который использовался в этом проекте.
Обратите внимание, что Describing Words использует сторонние скрипты (такие как Google Analytics и рекламные объявления), которые используют файлы cookie.Чтобы узнать больше, см. Политику конфиденциальности.
Понимание технических подписей посредством статистического анализа
Понимание технических подписей с помощью статистического анализаПонимание технических подписей с помощью статистического анализа
Нил К.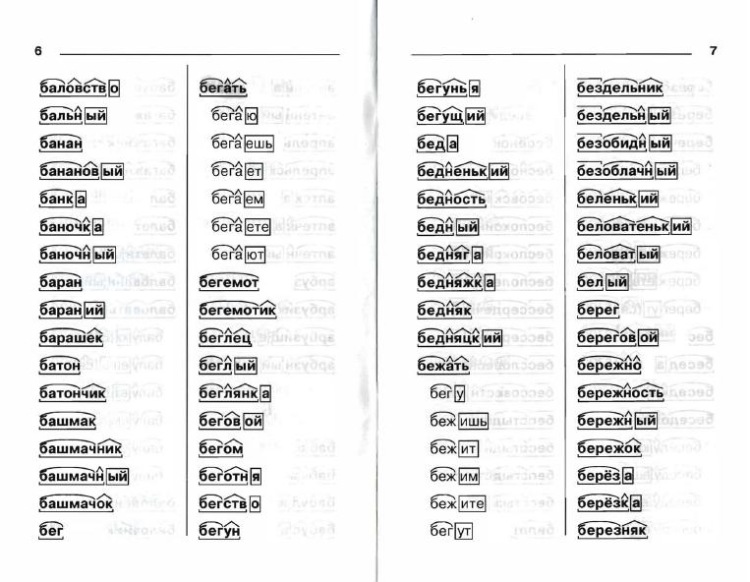
Абстрактные
Ключевой проблемой при индексировании технической информации является использование необычных технические слова и значения слов.Проблема важна для технические подписи к изображениям, подробные описания которых могут быть полезны расшифровать. Наш подход — предоставить общие инструменты для лексики. усиление специализированных слов и смыслов слов, а также обучение информация об использовании слов из учебного корпуса с использованием статистических парсер. Мы описываем обработку естественного языка для MARIE-2, a система поиска информации на естественном языке для мультимедийных титров. Мы используем статистику как по смыслам слов, так и по смысловым парам, чтобы направлять парсинг.Нововведения нашего подхода в статистической наследственности бинарных вероятностей совпадения и во взвешивании предложения подпоследовательности.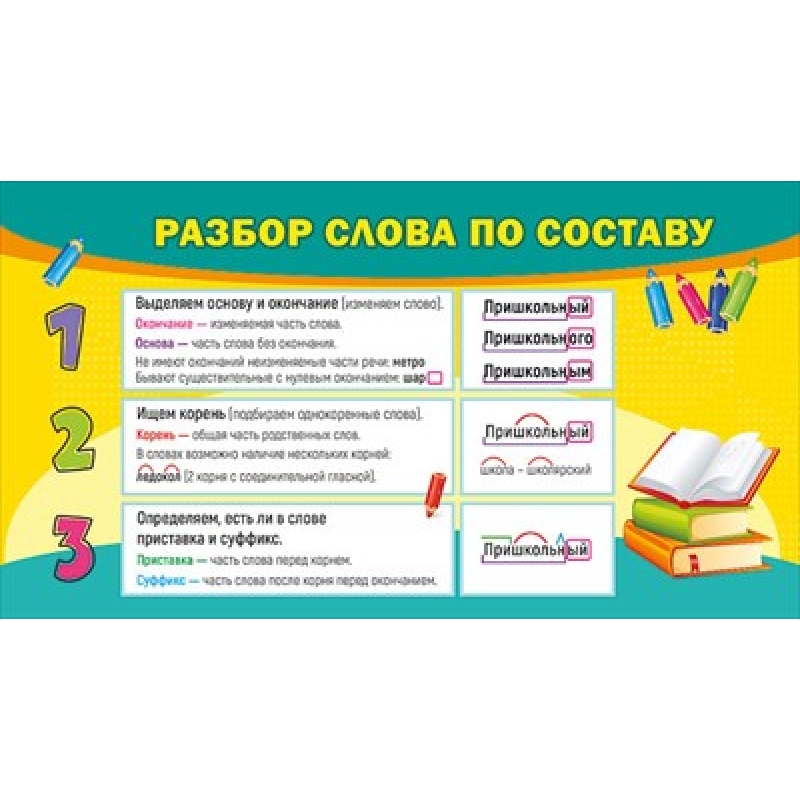
Эта работа была спонсирована DARPA в рамках проекта I3 в рамках AO.
8939, Армейским центром искусственного интеллекта и
U.С. Военно-морская аспирантура за счет средств начальника
Военно-морские операции. Спасибо Альберту Вонгу за помощь в программировании.
Вступление
Наш проект MARIE занимается поиском информации о мультимедийные данные с упором на обработку субтитров. Хотя СМИ анализ содержимого, такой как обработка изображений, устраняет необходимость изучить подписи, обработка подписей может быть намного быстрее, так как подписи резюмируйте важный контент в небольшом количестве слов.Проверка подписей перед извлечением мультимедийных данных может исключить плохие совпадает быстро, а субтитры могут предоставить информацию, которой нет в СМИ например, дата или заказчик фотографии.
Некоторая обработка субтитров на естественном языке необходима для
отзывчивость и точность запросов. Обработка должна определять смысл слова
и как соотносятся слова, чтобы выйти за общеизвестные пределы
соответствие ключевых слов (Krovetz and Croft, 1992). Это вызов для
специализированные технические диалекты. Автоматическая индексация для них могла
получить высокую отдачу, если немногие люди в настоящее время могут понять
подписи. Но с лингвистической точки зрения такие диалекты содержат (1) необычные слова,
(2) знакомые слова в необычном смысле, (3) кодовые слова, (4) акронимы,
и (5) новые синтаксические особенности. Ручное программирование нерентабельно
вся такая специфика для каждого технического диалекта. Нам нужно вывести большинство
из них из анализа репрезентативного технического корпуса. А потом
чтобы справиться с необычными особенностями диалектов, мы должны смешать
традиционный символический анализ с вероятностным ранжированием от
статистика.
Пока проект МАРИ предназначен для мультимедийной информации
в целом, в качестве испытательного стенда мы использовали фотолабораторию
Центр морской авиации (NAWC-WD), Чайна Лейк, Калифорния, США.Этот
это библиотека из около 100 000 картинок с 37 000 подписями для тех, кто
фотографий. Фотографии охватывают всю деятельность центра, в том числе
фотографии оборудования, тесты оборудования, административные
документация, посещение объектов и связи с общественностью. С таким большим количеством
картинки, многие непонятные обычному человеку, подписи
незаменимый, чтобы найти что-нибудь. Но существующее компьютеризированное ключевое слово
система поиска картинок по их подписям бесполезна и
в основном игнорируется персоналом.
(Guglielmo and Rowe, 1996) сообщает о MARIE-1, прототипе системы, которая
мы разработали для NAWC-WD систему, которая больше подходит
чего хотят пользователи. МАРИ-1 следовала традиционным методам
обработка естественного языка для поиска информации (Grosz et al,
1987; Рау, 1988; Сембок и ван Рейсберген, 1990) с использованием кодированных вручную
информация о лексике. Но на создание MARIE-1 ушло человеко-год.
обработал только 217 изображений (в среднем по 20 слов в заголовке) из
база данных, и ее охват неожиданных вопросов скорее
неточно.Чтобы добиться большего, MARIE-2 использует статистический анализ и
количество методов обучения. Разработка натолкнулась на несколько интересных
проблем и обеспечивает хорошую проверку применения статистических
анализ идей на нефильтрованный диалект реального мира. Парсер МАРИ-2
реализован на полукомпилированном языке Quintus Prolog и занял 5600 строк
исходный код указать.
Примеры подписей
Чтобы проиллюстрировать проблемы, вот примеры подписей из NAWC-WD. Все однокорпусные.
РЛС ан / APQ-89 XAN-1 в носовой части модифицированного самолета Т-2 Bu # 7074, для летно-оценочных испытаний.3/4 общего вида самолета на взлетно-посадочной полосе.
Это типично для многих подписей: две словосочетания с существительными, каждая из которых заканчивается.
с точкой, где первая описывает объект фотографии, а
второй описывает саму картинку. Также типичны сложные
штатные составы «РЛС ан / апк-89 ксан-1» и «т-2 конский глаз»
доработанный самолет бу №7074 «. Знание предметной области необходимо для
распознавать «an / apq-89» как тип радара, «xan-1» — номер версии для
тот радар, «т-2» тип самолета, «бакай» сленговое дополнительное название
для Т-2, «модифицированный» обычное прилагательное и «bu # 7074» в качестве
ID кода самолета. Обратите внимание, что несколько слов здесь меняют значение, когда
они изменяют другие слова. Таким образом, несинтаксический подход
система индексации (Silvester et al, 1994) для аналогичного домена имеет
ограничения здесь.
программный судак, АН / АВГ-16 на А-4С БУ # 147781 самолет, китайское озеро на хвосте, тест на пригодность. 3/4 общего вида спереди и крупным планом 1/4 вид спереди стручка.Это иллюстрирует некоторый зависящий от домена синтаксис. «А-4с бу № 147781» является общий шаблон <тип-оборудования> <код-префикса> <номер-кода>, «an / awg-16 fire control pod» — распространенный образец
графика презентация тид прогресс 76. море сайт обновление, голова осы радары визира / солнцезащитного козырька директора и ястреба. только верхняя часть, отлично.Это иллюстрирует потребность в зависящей от предметной области статистике по слову чувства.
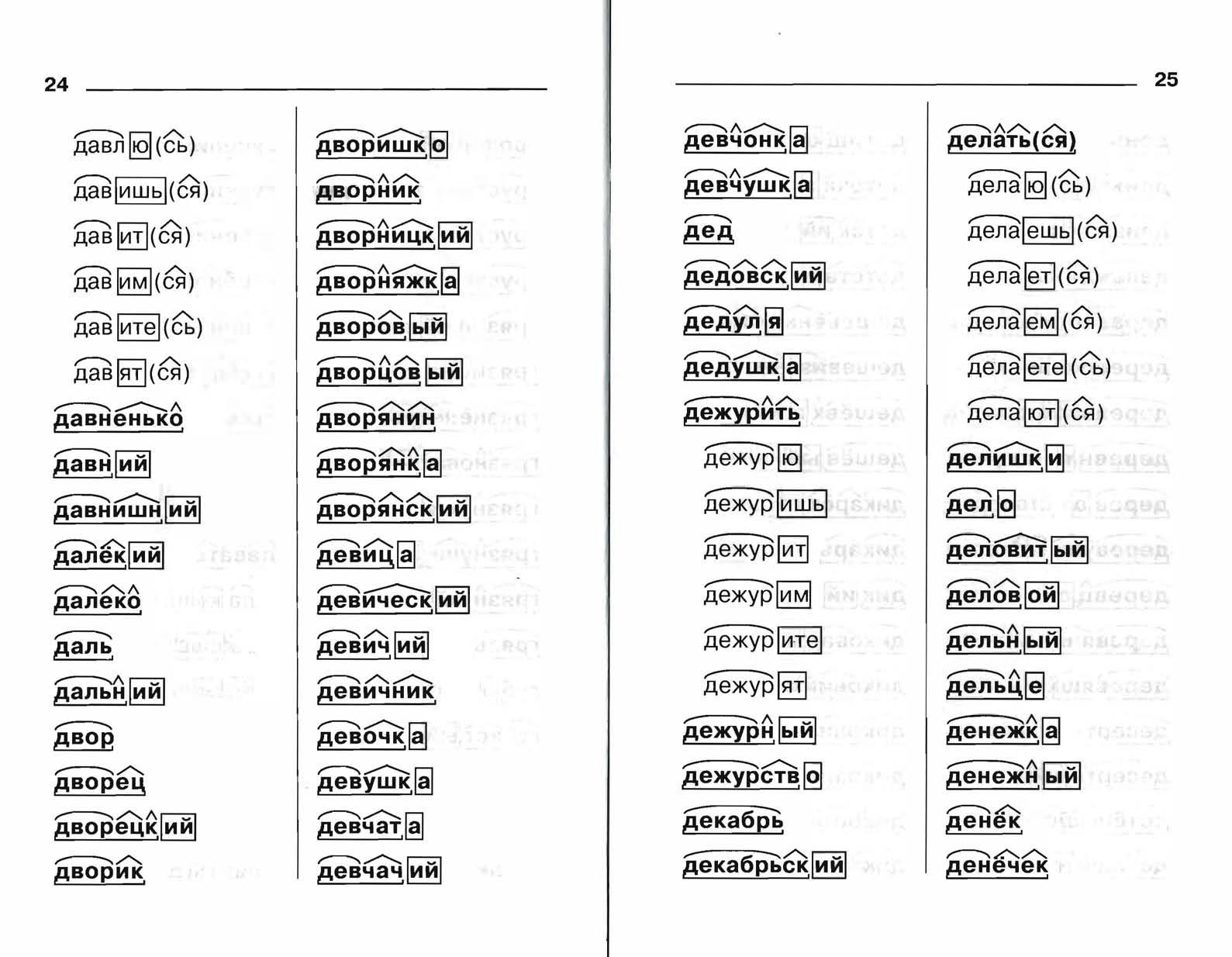
антенна с низким углом наклона, вид с современной дороги на главные ворота в Китае озеро бл к боумен роад. на l, b к t, водохранилища, trf crcl, pw cmpnd, vieweg school, корпус capehart b, берроуз hs, симаррон сады, восток т / ц старый дуплекс ст. много. от r, b до t, trngl, bar s мотель, стрелочник, комарко, больница и дальше на лучник роад.Это иллюстрирует распространенные орфографические ошибки и нестандартные сокращения. в подписях. «Trf crcl» — «круговая развязка», «trngl» — треугольник, «capehart b» — это «capehart base», а «b to t» — «снизу вверх».

В общем, правила синтаксиса в этом диалекте демонстрируют совершенно разные частоты использования по сравнению с повседневным английским, хотя только несколько правила не стандартные английские. На рис. 1 показан пример синтаксиса. правила и их частота, вычисленные в трех обучающих наборах. Также слова обычно менее двусмысленны, чем в повседневном английском: Of 1858 значений слов, использованных в трех обучающих наборах, 1679 были только смысл их слова.Тем не менее используются слова «смыслы». часто не самый распространенный в стандартном английском языке, и существует множество неоднозначности словесных отношений, которые необходимо разрешить.
Лексические вопросы
Создание полного списка синонимов, иерархии типов и иерархии частей для
приложений размером с базу данных NAWC-WD (42000 слов в
37 000 подписей, включая слова, близкие к тем, что в
captions) — это значительный труд. К счастью, общие слова покрыты
уже в Wordnet (Miller et al, 1990), большой тезаурусной системе,
включает синоним, тип и информацию о части, а также грубое слово
частоты и морфологическая обработка.Wordnet предоставил базовый
информация о 6000 слов в подписях NAWC-WD (или около
24000 значений слов).
Это оставило около 22000 неопознанных слов, которые были обработаны в
несколькими разными способами (см. рис. 2). Один класс определен-код
префиксы вроде «f-» в «f-18» для истребителей или вроде «es» в
«es4522» для номеров тестов. Есть несколько сотен специальных форматов
правила, которые интерпретируют «BU № 462945» как опознавательный знак воздушного судна.
число своим передним словом, «зубчатые горы» как горы своим
хвостовое слово, «21.02.93» — дата, «10-20м» — диапазон метров,
«посещение / посвящение» как сочетание, и «погрузка корабля» как
существительное-герундий эквивалент прилагательного.Орфографические ошибки и
сокращения были получены в основном автоматически, с человеческими
пост-проверка, используя методы, описанные в (Rowe and Laitinen,
1995). Формы лексического многоточия (например, «356» после «LBL 355») являются
также покрыты. Другие классы специально обрабатываемых слов являются техническими.
слова из МАРИ-1, морфологические варианты известных слов, чисел,
имена людей, названия мест и названия производителей. 1700 слов необходимо
явное определение нами в виде части речи и
суперконцепт или синоним.Остальные несекретные слова предполагаются
быть названиями оборудования, обычно безопасное предположение. Усилия для
создание лексикона было относительно скромным (0,3 человеко-года) благодаря
Wordnet, что говорит о хорошей переносимости.
Wordnet также предоставил нам 15 000 синонимов для слов в нашем
lexicon, и мы предоставили дополнительные синонимы для технического слова
чувства. Для каждого набора синонимов мы выбрали «стандартный синоним».
Указатели переходят от всех других синонимов к стандартному синониму, единственному
синонимом которого является подробная лексическая информация, такая как суперконцепты
хранится.
Мы помещаем всю словарную информацию в формат Пролога. Значение, присвоенное к существительному или глаголу в заголовке — это подтип его понятия в иерархия типов, а другие части речи соответствуют свойствам или отношения таких подтипов. Обычно каждое слово на входе caption соответствует одному выражению предиката с двумя аргументами в семантическом представление («список значений») подписи. Например, «ВМФ самолет на взлетно-посадочной полосе «после разбора имеет список значений:
[a_kind_of (v3, air-1), owner (v3, ‘USN’-1), over (v3, v5), a_kind_of (v5, взлетно-посадочная полоса-1)]где v3 и v5 — переменные, а числа после дефиса являются числами в смысле слова.
Парсинг
Мы решили использовать простую грамматику и относительно простые семантические правила,
чтобы увидеть, насколько мы можем полагаться на статистику вместо более тонких
различия (идея, аналогичная той из (Basili et al, 1992)). Для
например, корпус NAWC-WD часто показывает тип самолета
за которым следует «bu #» и идентификационный номер воздушного судна; мы
использовать общее правило для соединений существительное-существительное для подобных вещей
типы, и пусть статистика говорит нам, что номера BU часто следуют
самолет. Наша полная грамматика имеет 192 правила синтаксиса, 160 двоичных
(двухчленные) и 32 унарные (одночленные), а 71 бинарных правил являются
контекстно-зависимый. Контекстная чувствительность скромная и ненужная
для правильного разбора, но помогает эффективно обрабатывать длинные предложения. Для
Например, за аппозитивом, начинающимся с запятой, должен следовать
запятая, кроме конца предложения, и изменение причастного
фраза, за которой следует запятая, и именная фраза могут встречаться только в
перед предложением.
Двоичные правила имеют связанные семантические правила (всего 114, включая одно
правило по умолчанию), которые проверяют семантическую непротиворечивость, вычисляют общую
вероятность и вычислить получившийся список значений.14 из
семантические правила были специфичны для диалекта. Семантические правила
особенно подробно описан для трех общих конструкций в техническом
описание: соединения существительного и существительного, прилагательные и предложные
фразы. Правила составления существительное-существительное охватывают 55 падежей, например
тип-подтип («самолет Ф-18»), тип-часть («крыло Ф-18»), владелец-объект
(«флот ф-18»), объект-действие («взлет ф-18»), действие-объект
(«учебный Ф-18»), действие-локация («тренировочная площадка»), объект-концепция
(«Проект Ф-18»), так и типового («Ф-18 Харриер»). Правила для
аппозитивы охватывают 24 подобных случая, а также дополнительные
такие вещи, как объект-действие («крылья (сложенные)»). Правила предложного
фразы проверены падежная совместимость предлога с обоим подлежащим
и объект. Например, объект предлога местоположения
значение «в» может быть только местоположением, событием, диапазоном или обзором; это
предметом могло быть только место или событие.
Мы используем своего рода анализатор диаграмм снизу вверх (Чарняк, 1993, глава 6).
Мы работаем отдельно над каждым предложением подписи.Использование унарного
словесная статистика (см. ниже), наиболее вероятная интерпретация
каждое слово вводится в таблицу (без тегов части речи из
контекст (Brill, 1995) в настоящее время выполняется, хотя это может улучшить
представление). Затем мы выполняем поиск по ветвям и границам. Все слово и
индексируются и оцениваются фразовые интерпретации; лучший непроверенный
интерпретация выбирается для работы на каждом этапе. Все слова и фразы
интерпретации, которые примыкают к нему (без пробелов) слева и справа
рассматриваются для комбинации, проверяются по правилам грамматики, если
успешно проверено на семантические правила, а затем в случае успеха
ранжированы и добавлены в набор толкований слов и фраз. С участием
при таком подходе удаляются только повторяющиеся интерпретации, чтобы
не пропустить ничего. Первая найденная интерпретация охватывает
все слова и соответствует грамматической категории «подпись»
ответ, за исключением тренировок, когда первая интерпретация
представлен пользователю (или «тренеру») на утверждение, затем второй
найдена интерпретация и так далее, пока тренер не примет ее. На
принятие, упрощение конъюнктов и анафорическое справочное разрешение
выполнены (с использованием результатов для предыдущих предложений многопредложения
caption), а результат кэшируется (иногда идентичные подписи
происходить).Если удовлетворительной интерпретации не найдено, следующая лучшая
неисследованный смысл слова добавляется к диаграмме, и анализ продолжается.
Это делается столько раз, сколько необходимо. Если синтаксический анализ по-прежнему не удается,
лучшие интерпретации собраны для частичного
интерпретация.
Унарная статистика
Wordnet основан на традиционных печатных словарях и отличает
много смыслов слова. Таким образом, ключевой фактор в поиске лучшего предложения
интерпретация — это оценочная вероятность полученного смысла слова
путем экстраполяции подсчетов, наблюдаемых в тренировочном корпусе.(Оценки необходимы, поскольку корпус может охватывать только небольшой
часть смыслов английского слова.) Эти вероятности используют подсчеты от
сам смысл слова («унарные счета») и все соседние слова
смыслы в иерархии типов. Например, Sidewinder sense 1 — это
ракета и ракета — это оружие; 3733 упоминания о
«ракета» в корпусе и 1202 упоминания «оружия». Sidewinder
смысл 2 — это гремучая змея, а гремучая змея — это змея; есть 7
упоминания «гремучей змеи» и 6 упоминаний «змеи».Ослабляем соседние
считается с коэффициентом 2 на каждую пройденную ссылку; так что для смысла «Сайдвиндера»
1, «ракета» дает 3733/2 = 1866,5, а «оружие» дает
1202/4 = 300,5. Мы ограничиваем поиск тремя ссылками с самого начала
точку в описанных здесь экспериментах, и рассмотрим оба направления вверх
(суперконцепция) и нисходящие ссылки.
Для «sidewinder» мы получили
взвешенное общее количество 1094 для ракетного датчика и 497 для
змеиное чувство.
Следует отметить три дополнительных момента. Во-первых, унарный счет должен включать также «косвенные» подсчеты или подсчеты всех подтипов; это позволяет оценка вероятности редких ощущений.Во-вторых, если смысл слова стандартный синоним, то синоним — сосед (отдельные подсчеты сохраняются для каждого синонима). В-третьих, если есть несколько суперконцепции, например, когда железо является одновременно элементом и металлом, оба получают одинаковый полный вес, потому что оба применяются полностью.
Счетчики обучения часто могут быстро указать общее специализированное слово
смыслы, используемые в техническом диалекте. Это означает, что МАРИ-2 учится у
опыт, с помощью информации, извлеченной из списков значений, принятых
тренер.Расчет косвенных подсчетов занимает много времени, поэтому
пересчет производился только после каждой обучающей выборки. Начальные подсчеты
были получены из подсчета всех сырых слов во всех 36 191
подписи, распределяя счет для каждого слова поровну между его
возможные чувства; другие смыслы, кроме существительного, глагола, прилагательного или наречия
предполагалось, что это происходит в 80% случаев, чтобы предотвратить избыточный вес существительного
воспринимает «банка» как контейнер и «a» как форму.
Бинарная статистика
Статистический анализ обычно использует вероятности строк
последовательные слова в предложении (Jones and Eisner, 1992; Charniak,
1993).Двоичная статистика (количество совпадений пар
слова) естественно вписываются в наши правила двоичного синтаксического анализа, так как вероятность
совместное появление двух частей, как альтернатива более сложным
семантические ограничения (Basili et al, 1996). Например, парсинг
«Посадка Ф-18» с правилом «НП -> НП УЧАСТИЕ» должна быть
хорошо, потому что F-18 часто приземляются, в отличие от «наверху» в «наверху».
посадка «. Оценки вероятностей совместной встречаемости могут унаследоваться от
иерархия типов (Rowe, 1985). Итак, если у нас недостаточно данных о том, как
часто приземляется F-18, нам может хватить того, как часто самолет
земли; и если F-18 являются типичными самолетами в этом отношении, как часто
Приземление F-18 является результатом подсчета «приземлений самолета» и соотношения
от счета на «F-18» до счета на «самолет». Оба смысла слова
можно обобщить при поиске счетчиков, поэтому мы можем использовать статистику
на «F-18» и «движущийся», или на «самолет» и «движущийся». Или мы можем пойти
далее вверх по иерархии типов, чтобы получить статистику по «транспортному средству» и
«движущийся». Идея состоит в том, чтобы найти достаточную статистику для справедливого
оцените вероятность совпадения слов. Это также делает
смысл объединять учитывает все морфологические варианты вместе, поэтому
рассчитывать на «F-18» и «земля» также будет охватывать существительную фразу «usn
только что приземлились Ф-18 ».
Для управляемости и статистической надежности мы ограничиваем количество
те, которые состоят не более чем из двух слов (но принимая многословные имена собственные
одним словом). При объединении многословных последовательностей мы используем
подсчитывает наиболее важные слова или «заглавные слова». Например, »
большой F-18 с китайского озера, приземляющегося на армитедж-филд «также можно разобрать
от «NP -> NP PARTICIPLEPHRASE» с двоичным счетом для «f-18» и
использовано слово «посадка», поскольку «f-18» — это главное существительное и, следовательно, заглавное слово
«большой f-18 с китайского озера», а «приземление» — это причастие и
отсюда и заглавие «приземление на армитажном поле». Заглавные слова фраз
обычно очевидны. Бинарное ограничение согласуется с
важность дел (принципиально бинарная концепция) с большинством
технический язык именных фраз, но иногда пропускаю некоторые
важные более отдаленные отношения в предложениях, например, между
«самолет» и «посадка» в самолет, который потерпел крушение в Норфолке
посадки «. Мы думаем, что они слишком редки, чтобы вызывать беспокойство в большинстве
технические диалекты.
Каждый счетчик должен быть классифицирован правилом синтаксического анализа, поэтому альтернативный
анализ «посадки f-18» «NP -> ADJECTIVE GERUND» будет относиться к
разные подсчеты.Чтобы помочь с именными составами и аппозитивами,
что может быть сложно, мы дополнительно подкатегоризуем счетчики по
постулируются отношения между двумя заглавными словами. Например,
«средства оценки» могут означать, что средства являются агентом
оценки или оценка происходит на объектах. Таким образом, мы
вести отдельную статистику для каждого отношения существительное-существительное.
Одним из преимуществ наследуемых двоичных чисел является идентификация неизвестные слова. Хотя мы еще не используем это, категории для неизвестные слова можно заключить по их вероятности сопровождения смысл соседнего слова.Например, в «Кадровом монтаже GHW-12» на f-18 «,» ghw-12 «должно быть оборудование из-за высокого вероятность совпадения терминов «оборудование» со словами «монтировать» и «включено».
Во время обучения статистика двоичного счета изначально не используется. Они
вычисляются из принятых предложений после того, как каждый обучающий набор
был запущен как своего рода инициатор загрузки (Richardson, 1994). Количество
увеличивается для каждого узла дерева синтаксического анализа, а также для всех
задействованы суперконцепции слов, поэтому совместное появление слов «Navy» и
«Сайдвиндер» — это также совместное появление «ВМФ» и «Ракеты»,
«организация» и «Сайдуиндер», и «организация» и «ракета».(Есть исключения для кодовых слов, чисел и дат: мы сохраняем только
статистику их суперконцепций). правила грамматики, добавляя соответствующие счетчики пар слов.
Хранилище двоичных подсчетов потребовало тщательного проектирования, так как данные
немногочисленны и содержат много мелких записей. Например, для
Подписи NAWC-WD насчитывают около 20 000 наборов синонимов, о которых мы
иметь информацию о лексике. Это означает 200 миллионов возможных
пары совпадений, но сумма всех их подсчетов может быть только
610 182, общее количество экземпляров слова во всех подписях.Наш
структура данных двоичных подсчетов использует четыре дерева поиска, проиндексированных на
первое слово, часть речи плюс смысл первого слова,
второе слово и часть речи плюс смысл второго слова
слово. Различные методы сжатия могут еще больше уменьшить объем памяти,
как идея пропуска счетчиков в пределах стандартного отклонения от
прогнозируемое значение (Rowe, 1985). Стандартное отклонение при | n | это
размер субпопуляции, | N | — размер населения, а | A |
счетчик населения равен | sqrt {A (N — A) (N — n) / n N
sup 2 (N — 1)} | (Кокран, 1977).
Контроль парсинга
Наш синтаксический анализатор использует четыре фактора для ранжирования возможных интерпретаций фраз: (1) унарный счет на смыслы слова, (2) счет на грамматику используемых правил, (3) двоичные подсчеты на смыслы заглавного слова, соединенные в дерево синтаксического анализа и (4) различные факторы, такие как расстояние между двумя заглавными словами и совместимостью союзов по длине и тип. Обычно к ним относятся как к вероятностям (пока игнорируем некоторый коэффициент нормализации) и умножьте их, чтобы получить вероятность (вес) для всего предложения (Fujisaki et al, 1991).Для n-слова фраза, которую мы можем использовать:
вес = l sup n-1 PI sub i = 1 sup n [n (w sub i)] PI sub j = 1 sup n-1 [n (g sub j)] PI sub j-1 sup n-1 [a (g sub j)] PI sub j-1 sup n-1 [m (g sub j)]где | l | это постоянный контроль над нашей предвзятостью к более длинным фразам, | n (w sub i) | это количество значений слова, выбранного для i-е слово во фразе | n (g sub j) | счет грамматики правило, используемое в j-м (при предварительном обходе дерева синтаксического анализа) применение бинарных правил при синтаксическом анализе фразы | a (g sub j) | степень ассоциации заглавных слов присоединяемых подфраз по j-му бинарному правилу и | m (g sub j) | разные весовые коэффициенты.

Если мы возьмем отрицательные значения логарифма веса, проблема
становится одним из поисков интерпретации предложения с минимальными затратами, где
Стоимость рассчитывается путем сложения коэффициентов из всех частей. Тогда
задача синтаксического анализа состоит в том, чтобы оценить, какие интерпретации фраз являются
скорее всего, приведет к хорошей интерпретации предложения, что означает
оценка факторов стоимости для комбинаций подфраз еще не известна.
Поскольку алгоритм A * доказуемо оптимален и решает такие
проблема, мы используем ее вариант.Для истинного A * нам нужны нижние оценки на
затрат (от верхних границ вероятности до принятия отрицательных значений
логарифмы) оставшихся множителей и оставшихся слов в
приговор. В качестве фактора унарного счета мы можем считать
самый здравый смысл слова. В качестве фактора правила грамматики мы можем взять
количество наиболее распространенных грамматических правил. Но двоичные подсчеты
фактор не ограничен, так как степень ассоциации может варьироваться
сильно зависит от корпуса, и разные факторы
трудно масштабировать. Таким образом, мы не будем масштабировать их и не получим истинного A *.
алгоритм, хотя будет использовать его механику. Это дает пересмотренный
формула:
вес = l sup n-1 PI sub i = 1 sup n [f (w sub i)] PI sub j = 1 sup n-1 [f (g sub j)] PI sub j-1 sup n-1 [a (g sub j)] PI sub j-1 sup n-1 [m (g sub j)]где | l | постоянная; | f (w sub i) | это счет i-го слова смысл в предложении делится на количество наиболее здравого смысла Это слово; | f (s sub j) | это счет грамматического правила, используемого в j-е приложение бинарных правил, разделенное на количество наиболее общее грамматическое правило; | a (g sub j) | степень ассоциации два заглавных слова подфраз, объединенных j-м бинарным правилом; а также | m (g sub j) | это сводка различных факторов на j-м бинарное правило.| L | автоматически увеличивается периодически, когда система не может найти интерпретацию предложения, а также корректируется вверх или вниз, когда какое-либо толкование предложения старайтесь, чтобы веса предложений находились в диапазоне от 0,1 до 10,0; это уменьшает склонность парсера зацикливаться на предложениях с незнакомыми словосочетания.

Степень ассоциации может быть приблизительно выражена соотношением наблюдаемое количество двух заглавных слов в этом синтаксическом отношении к ожидаемый счет.Ожидаемое количество можно оценить с помощью лог-линейной модель, что означает подсчет этого синтаксического отношения в корпус умножить на пропорциональную частоту значений двух слов в корпус:
a (s sub j) = [b (w sub j1, w sub j2) n (g (w sub j1)) n (g (w sub j2))] / [b (g (w sub j1), g (w sub j2)) n (w sub j1) n (w sub j2)]где | b | — двоичная частота, | n | унарная частота | j1 | в значение первого слова, | j2 | смысл второго слова, и | g | самый верхний обобщение смысла слова (которое для Wordnet означает «сущность» 1 для существительных и значение «действовать» 2 для глаголов).Это больше 1 для положительно связанные слова и меньше 1 для отрицательно связанных слова. По умолчанию для словосочетаний без статистика.
Возьмем для примера предложение «капсула на Ф-4»; На рис. 3 показан полный набор созданы записи фразового перевода. Первый аргумент для каждого: порядковый номер (и порядок создания) каждой записи; второй и третьи аргументы — это начальные и конечные числа слов в предложение ввода, которое охватывает запись; четвертый аргумент — это часть речи; пятый — список значений; шестой обратный указатель (-ы) на исходную запись или записи; а седьмой масса.Записи 1-29 на рис. 3 охватывают отдельные слова и представляют исходные данные, стартовая повестка для поиска. За «капсулу на Ф-4» мы обнаружил в Wordnet три значения слова «стручок» как существительного (фрукт, группа животных, и контейнер) и одно зависящее от предметной области значение глагола (что означает поставить что-то в контейнер). Только третье значение существительного произошло в тренировочный корпус, поэтому ему был придан унарный вес почти 1 и другое существительное воспринимает значение по умолчанию 0,015; «стручок» как глагол получил еще меньший вес 0,0005, поскольку форма корневого глагола встречается редко и истинные глаголы статистически намного реже, чем причастия в этом база данных.»On» может быть предлогом местоположения, ориентацией предлог, временной предлог или наречие; только первое чувство встречается в корпусе, поэтому он получил унарный вес 1, а остальные 0,002. «F-4» однозначно и имеет вес 1. Смысл каждого слова затем обобщается всеми возможными унарными правилами синтаксического анализа; так что существительное может быть «ng», «ng» может быть «adj2», а «adj2» может быть «np». Для грамматических терминов с несколькими обобщениями (например, когда «нг» может быть «np», а также «adj2»), вес делится между обобщения, поэтому «pod-3» как «np» получает вес 0.5.
Из трех пунктов стартовой повестки дня, совпадающих по наибольшему весу, интерпретация местоположения слова «on» была выбрана произвольно, чтобы объединить с другими пунктами повестки дня. Соединив его со словом справа, мы получил запись 30 с подфразой «на ф-4», имеющей список значений [на (v6, v12), a_kind_of (v12, ‘F-4’-0)] . Это вычислил вес | 0,999 * 0,5 * 1 * 1,018 * 1 = 0,509 | из соответственно вес для этой интерпретации «на», вес для «F-4», вес правила-силы (так как это единственное правило, которое создает pp), степень ассоциации 1.018 из «ОН» и «Ф-4» (унаследовано от степень «на» и «истребитель» смысл 4, чувство самолета) и 1 за отсутствие разных факторов. В конце концов поиск выбрал запись 30, над которой нужно работать, и комбинируя ее с тремя смыслами существительного «pod» сгенерировал записи 31, 33 и 35, которые были немедленно обобщены до грамматической категории «подпись» унарным синтаксическим анализом правило для получения записей 32, 34 и 36. Запись 35 имеет гораздо больший вес чем остальные из расчета | 0,5 * 0,509 * 1 * 1.377 * 1 = 0,351 | где 0,5 — вес для «стручка» смысла 3, 0,509 — вес для «на F-4» 1 вес правила, 1,377 степень ассоциации «стручок» смысл 3 и «вкл» (ассоциация, которая действительно возникла во время обучения корпус и не должны передаваться по наследству), без прочего факторы. Запись 36 является окончательным ответом, но поиск продолжался. пока все потенциально лучшие кандидаты в повестке дня не были исследовал.
На рис. 4 показан более длинный пример и сравнивается вывод парсера MARIE-2 с МАРИ-1 для подписи из первого обучающего набора.Wordnet также присутствуют чувства сверхконцепции. Мощность МАРИ-1 меньше точный (без значений слов и с очень общими именами сказуемого), более сложный, и показывает влияние чрезмерно специализированных правил, как в обработка координат. МАРИ-1 не могла соединить предложения, а также ошиблись в идентификации DVT-7 как оборудования и «пробега 2» как прямой объект испытания. В общем, списки значений МАРИ-2 были значительно более точны, чем у MARIE-1, потому что мы могли принудительный откат во время обучения, чтобы получить наилучшее представление подпись, не просто адекватная, а значительно более сложная помогли грамматические и семантические особенности МАРИ-2.
Во время тренировок мы разрешили тренеру исключить определенные неправильные значения слов и определенные неправильные отношения слов в интерпретации предложения (но можно было указать только одно в предложении интерпретации, чтобы наша статистика была значимой для количества пытается). Таким образом, инструктор может сказать парсеру исключить «sidewinder» смысл 2, отношения части-целого, причастия прошедшего времени, конъюнктив связи или даже конкретное выражение предиката, говорящее чувство самолета 1 обладало чувством бокового ветра 2.
Эксперименты
В наших экспериментах мы использовали последовательно три обучающих набора. Статистика представлена на рис. 5. Первым набором было 217 полных субтитры обрабатывала МАРИ-1 (Guglielmo and Rowe, 1996). Это были из фотолаборатории NAWC-WD, и изначально были созданы на основе случайных образец суперзаголовков (подписей к классам картинок), расположение фактические папки с субтитрами и расшифровка того, что было написано на папки. Поскольку титры для одного и того же суперкаптита очень близки связанные, многие из подписей имели похожую формулировку.Некоторая коррекция Ошибки в подписях были сделаны, как описано в предыдущей статье. Начальная приблизительная унарная статистика по всем смыслам слов в корпусе оценивались по частотам соседних слов. После тренировки на в этом наборе мы вычислили статистику смысла слов и запустили второй обучающий набор с их использованием. Второй набор представлял собой другую случайную выборку. только 108 суперзаголовков с небольшой избыточностью. Нет руководства исправлены ошибки, и эти подписи было трудно разобрать. Третий набор — 112 подписей, извлеченных вручную. составляющие почти все изображения с подписями, доступные на NAWC-WD Сайт в Интернете, июнь 1997 г.У них был более изысканный язык и использовал некоторые ранее невиданные грамматические конструкции, такие как конъюнктивные прилагательные и относительные придаточные, но в большинстве случаев не сложно разобрать. Эти подписи не использовались во время нашего первоначального лексикона, и они потребовали определения ряда новых слова смыслы. Мы закончили подсчетом 1931 значения слов и 4018 значений. слово-смысловые пары. На разработку и тестирование ушло около человеко-года. работы.
Успешные синтаксические разборы были обнаружены для всех предложений, кроме двух, не содержащих грамматику первой обучающей выборки.Мы заставили систему откатиться до тех пор, пока она нашел наилучшую возможную интерпретацию предложения, отладив парсер при необходимости. На рис. 6 показано полное распределение синтаксического анализа. раз в секундах процессора (с использованием полукомпилированного Quintus Prolog) для 437 титры как функция длины предложения в количестве слов; оба оси отображают натуральные логарифмы их количеств. Значительный вариация очевидна. Рис. 7 и 8 показывают среднее (среднее геометрическое) проанализировать время процессора и количество попыток для каждой возможной длины предложения, снова в виде логарифмов; пунктирная линия — первый обучающий набор, толстая сплошная линия — вторая, тонкая сплошная линия — третья.Видно, что время синтаксического анализа и количество попыток увеличились на второй обучающий набор из-за повышенной сложности парсера после отладку, но не для третьего обучающего набора впоследствии. Этот предполагает, что эффекты инициализации теперь исчезают, а производительность останется неизменным или улучшится с дополнительными обучающими подписями. Также обратите внимание, что все кривые имеют линейный тренд на этих графиках log-log. Мы применил регрессию наименьших квадратов ко всем данным логарифма, чтобы получить подходит для времени в секундах | 0.0858 n sup 2.876 | а для числа попыток | 1.809 n sup 1.668 |, где | n | количество слов в предложение, и со степенью значимости 0,531 и 0,550 соответственно. Подходит не очень хорошо, но дает грубый способ прогнозировать производительность.
На рис. 9 перечислены некоторые репрезентативные предложения средней длины из третий тренировочный набор. На рис. 10 показано процессорное время синтаксического анализа и количество требуются попытки, прежде чем получить наилучшую интерпретацию для каждого. В первая пара чисел во время обучения с использованием статистики из первые два тренировочных набора.Вторая пара чисел из повторный прогон после включения статистики по всей третьей тренировке набор. Видно, что тренировки помогают, но не намного на более коротких предложения; лучшее улучшение было для последнего предложения с статистические данные указывают на то, что необычные конструкции герундий и использовались предложные наречия. Третья пара чисел в Рис. 10 — для условий, подобных второму, за исключением того, что двоичная статистика смысла слова. Видимо бинарная статистика может повредить некоторые предложения немного (на дополнительное время для наследования), но могут помочь в более длинных предложениях, таких как седьмое с его многочисленными именными соединения.Четвертая пара цифр на рис.10 — это условия вида второй, кроме без унарной статистики, так что все значения слова были оценены как равновероятные. Это значительно снижает производительность, предполагая, что большая часть ценности обучения этому диалекту заключается в изучение общих смыслов слова.
Запросы
Мы также предоставляем возможность запроса на естественном языке с помощью анализатора. Запрос на английском языке, полученный от пользователя, анализируется и интерпретируется. Его переменным даны специальные имена, отличные от переменной заголовка. имена, а также временные и числовые обозначения удаляются.»Грубого помола сопоставление «затем выполняется, чтобы найти подписи, содержащие каждый тип или его подтип, указанный в запросе; эта идея была реализована в МАРИ-1, но здесь мы сначала разбираемся, чтобы получить более точные типы. Этот требуется полный указатель по всем типам, упомянутым в подписи. Подпись кандидаты, прошедшие крупнозернистый матч, затем подлежат «точное соответствие» полного списка значений запроса список значений заголовков. Это стандартное недетерминированное совпадение с с возвратом и должно быть точным совпадением.Мы используем разумные эвристика, что правильная интерпретация неоднозначного запроса — это интерпретация с наивысшим рейтингом, которая имеет ненулевое точное соответствие, и мы возвращаемся по мере необходимости, пока не найдем такое толкование. Затем отображаются изображения, соответствующие совпадающим подписям.
Выводы
Мы попытались выполнить сложную задачу по синтаксическому анализу содержательных предложений в специализированный диалект реального мира с необычным новым смыслом слов, творческий синтаксис и ошибки.Наши результаты показывают, что это возможно, и статистический анализ помогает, но требуется много работы. Мы ожидаем что в конечном итоге производительность должна улучшиться с дальнейшими тренировками. предложения из корпуса, но мы еще не дошли до того момента, как мы по-прежнему сталкиваемся с новыми синтаксическими конструкциями и семантическими отношениями после 803 тщательно разобранных предложений. Надеемся, наши эксперименты будут полезно для других исследователей, занимающихся многими специализированными технические диалекты, для которых автоматизированное понимание может быть очень ценный.
Рекомендации
Basili, R., Pazienza, M., and Velardi, P. Неглубокая синтаксическая анализатор для извлечения словесных ассоциаций из корпусов. Литературный и лингвистические вычисления, 7 , 2 (1992), 114-124.
Базили, Р., Пазиенца, М. Т., и Веларди, П. Эмпирический символический подход к обработке естественного языка. Искусственный интеллект, 85 (1996), 59-99.
Brill, E. Обучение на основе преобразований, основанное на ошибках, и естественное языковая обработка: пример использования тегов части речи. Computational Linguistics, 21, 4 (декабрь 1995), 543-565.
Чарняк Э. Статистическое изучение языков . Кембридж, Массачусетс: Массачусетский технологический институт Пресс, 1993.
Cochran, W. G. Sampling Techniques, третье издание . Нью-Йорк: Wiley, 1977.
Fujisaki, T., Jelinek, F., Cocke, J., Black, E., and Nishino, T. A вероятностный метод анализа для устранения неоднозначности предложений. В Актуальные вопросы технологии парсинга , изд. Томита М., Бостон: Kluwer, 1991.
Гросс, Б., Аппельт, Д., Мартин, П. и Перейра, Ф. КОМАНДА: An экспериментируйте с дизайном мобильных интерфейсов на естественном языке. Искусственный интеллект, 32 (1987), 173-243.
Гульельмо, Э. и Роу, Н. Поиск изображений на естественном языке на основе по описательным подписям. транзакций ACM в информационных системах, 14 , 3 (май 1996 г.), 237-267.
Джонс, М. и Эйснер, Дж. Вероятностный синтаксический анализатор, применяемый к программному обеспечению. документы тестирования.Труды Десятой национальной конференции по Искусственный интеллект, Сан-Хосе, Калифорния, июль 1992 г., стр. 323-328.
Кровез Р. и Крофт У. Б. Лексическая двусмысленность и информация поиск. Транзакции ACM в информационных системах, 10 , 2 (Апрель 1992 г.), 115-141.
Миллер Г., Беквит Р., Феллбаум К., Гросс Д. и Миллер К. Пять статей о Wordnet. Международный журнал лексикографии , 3, 4 (зима 1990 г.).
Рау Л. Организация знаний и доступ к концептуальной информации система. Обработка информации и управление , 23, 4 (1988), 269-284.
Richardson, S.D. Начальная статистическая обработка в синтаксический анализатор естественного языка на основе правил. Процедура Закона о балансировании: Сочетание символического и статистического подходов к языку, ассоциации для компьютерной лингвистики, Лас-Крусес, Нью-Мексико, июль 1994 г., стр. 96-103. Также технический отчет Microsoft MSR-TR-95-48.
Роу, Н. Антисэмплинг для оценки: обзор. IEEE Транзакции по разработке программного обеспечения, SE-11 , 10 (октябрь 1985 г.), 1081-1091.
Роу, Н. и Лайтинен, К. Полуавтоматическое сокращение технических текст. Обработка информации и управление, 31 , 6 (1995), 851-857.
Сембок Т. и ван Рейсберген К. СИЛОЛ: простой логико-лингвистический система поиска документов. Обработка и управление информацией, 26 , 1 (1990), 111-134.
Сильвестр, Дж. П., Генуарди, М. Т., и Клингбиль, П. Х. Автоматическая индексация в НАСА. Обработка информации и управление, 30 , 5, 631-645.
Пример частоты правила adj2 + ng = ng 1986 "флот" + "самолет" b_prtp + np = prtp2 107 "тестирование" + "сиденье" art2 + ng = np 174 "the" + "флот" adv + причастие = a_prtp 23 "только что" + "загружено" существительное + число = нг 75 "тест" + "0345" timeprepx + np = pp 60 "во время" + "тест" locprepx + np = pp 639 "на" + "на земле" miscprepx + np = pp 565 "с" + "приборный блок" np + pp = np 1115 «Самолет ВМФ» + «во время испытаний» np + prtp = np 261 "член экипажа" + "загрузка капсулы" vg + np = vp2 30 "загрузок" + "приборная панель" np + vp = snt 33 "член экипажа" + "загружает капсулу" vp2 + pp = vp2 14 "нагрузки" + "на самолет" adv + pp = pp 21 "просто" + "под самолетом" con + np = cj_np 152 "и" + "самолет" np + cj_np = np 177 "санки" + "и манекен" np + c_aps = np 36 "самолет" + ", F-18," ng + aps = ng 178 "самолет" + "(F-18)" np + aps = np 46 "самолет" + "(F-18)" np + c_np = np 53 "самолет" + ", F-18" np_c + np = np 50 "салазки" + "манекен" [ошибка запятой] prtp2 + pp = prtp2 116 "только что загружено" + "на самолете" infinmarker + vp = ip 10 "to" + "load" con + vp = cj_vp 3 "и" + "загружает самолет" snt + cj_snt = snt 2 "экипаж загружает" + "и офицер руководит"Рисунок 1: Пример синтаксических правил с указанием их частоты в вывод парсера на трех обучающих наборах.
Количество подписей 36 191 Количество слов в титрах 610 182 Количество различных слов в заголовках 29 082 Подмножество, имеющее явные записи в Wordnet 6729 (Количество из них, для которых дан предпочтительный псевдоним: 1847) (Количество значений этих слов: 14 676) Подмножество с определениями многоразового использования из MARIE-1770 Подмножество с определениями, написанными специально для MARIE-2 1763 Подмножество морфологических вариантов других известных слов 2335 Подмножество чисел 3 412 Подмножество имен людей 2791 Подмножество географических названий 387 Подмножество названий производителей 264 Подмножество с однозначными префиксами определенного кода 3256 (Однозначные префиксы определенного кода: 947) Подмножество других идентифицируемых специальных форматов 10,179 Подмножество идентифицируемых орфографических ошибок 1174 (Ошибки в написании найдены автоматически: 713) Подмножество идентифицируемых сокращений 1093 (Сокращений найдено автоматически: 898) Остальные слова, предположительно названия оборудования 1876 Явно использованные факты псевдонимов Wordnet вышеуказанных слов Wordnet 20 299 В лексикон добавлены дополнительные псевдонимы помимо словаря субтитров 9 324 Явно созданные факты псевдонимов вышеупомянутых слов, не относящихся к Wordnet 489 Другие факты о псевдонимах Wordnet, использованные для упрощения лексики 35,976 В лексикон добавлены дополнительные смыслы, помимо словаря субтитров 7899 Общее количество обработанных значений слов 69 447 (включает связанные суперконцепции, целые и фразы)Рисунок 2: Статистика по словарю MARIE-2 для подписей NAWC-WD после обработка первой обучающей выборки.
p (1,1,1, глагол, [a_kind_of (v1, pod-100), quantification (v1, множественное число)], [], 0,000544). p (2,1,1, vg, [a_kind_of (v1, pod-100), quantification (v1, множественное число)], [1], 0,000544). p (3,1,1, vp2, [a_kind_of (v1, pod-100), quantification (v1, множественное число)], [2], 0,000544). p (4,1,1, vp, [a_kind_of (v1, pod-100), quantification (v1, множественное число)], [3], 0,000544). p (5,1,1, существительное, [a_kind_of (v2, pod-1)], [], 0,015151). p (6,1,1, ng, [a_kind_of (v2, pod-1)], [5], 0,015151). p (7,1,1, adj2, [a_kind_of (v2, pod-1)], [6], 0,007575). p (8,1,1, np, [a_kind_of (v2, pod-1)], [6], 0.007575). p (9,1,1, существительное, [a_kind_of (v3, pod-2)], [], 0,015151). p (10,1,1, ng, [a_kind_of (v3, pod-2)], [9], 0,015151). p (11,1,1, adj2, [a_kind_of (v3, pod-2)], [10], 0,007575). p (12,1,1, np, [a_kind_of (v3, pod-2)], [10], 0,007575). p (13,1,1, существительное, [a_kind_of (v4, pod-3)], [], 0.999969). p (14,1,1, ng, [a_kind_of (v4, pod-3)], [13], 0.999969). p (15,1,1, adj2, [a_kind_of (v4, pod-3)], [14], 0.499984). p (16,1,1, np, [a_kind_of (v4, pod-3)], [14], 0.499984). p (17,2,2, locprep, [свойство (v6, on)], [], 0.999995). p (18,2,2, преп, [свойство (v6, on)], [17], 0.999995).p (19,2,2, miscprep, [свойство (v7, ориентация)], [], 0,002439). p (20,2,2, преп, [свойство (v7, ориентация)], [19], 0,002439). p (21,2,2, adv, [свойство (v8, on-150)], [], 0,002439). p (22,2,2, timeprep, [свойство (v9, в течение)], [], 0,002439). p (23,2,2, преп, [свойство (v9, во время)], [22], 0,002439). p (24,2,2, miscprep, [свойство (v10, объект)], [], 0,019512). p (25,2,2, преп, [свойство (v10, объект)], [24], 0,019512). p (26,3,3, существительное, [a_kind_of (v12, 'F-4'-0)], [], 0.999833). p (27,3,3, ng, [a_kind_of (v12, 'F-4'-0)], [26], 0.999833). p (28,3,3, adj2, [a_kind_of (v12, 'F-4'-0)], [27], 0.499916). p (29,3,3, np, [a_kind_of (v12, 'F-4'-0)], [27], 0.499916). p (30,2,3, pp, [on (v6, v12), a_kind_of (v12, 'F-4'-0)], [[17,29]], 0.508953). p (31,1,3, np, [a_kind_of (v2, pod-1), on (v2, v12), a_kind_of (v12, 'F-4'-0)], [[8,30]], 0,002336 ). p (32,1,3, подпись, [a_kind_of (v2, pod-1), on (v2, v12), a_kind_of (v12, 'F-4'-0)], [31], 0,002336). p (33,1,3, np, [a_kind_of (v3, pod-2), on (v3, v12), a_kind_of (v12, 'F-4'-0)], [[12,30]], 0,002341 ). p (34,1,3, подпись, [a_kind_of (v3, pod-2), on (v3, v12), a_kind_of (v12, 'F-4'-0)], [33], 0.002341). p (35,1,3, np, [a_kind_of (v4, pod-3), on (v4, v12), a_kind_of (v12, 'F-4'-0)], [[16,30]], 0 .350505). p (36,1,3, подпись, [a_kind_of (v4, pod-3), on (v4, v12), a_kind_of (v12, 'F-4'-0)], [35], 0.350505). p (37,1,3, vp2, [a_kind_of (v1, pod-100), quantification (v1, множественное число), on (v1, v12), a_kind_of (v12, 'F-4'-0)], [[3,30]], 0,000000). p (38,1,3, vp, [a_kind_of (v1, pod-100), quantification (v1, множественное число), on (v1, v12), a_kind_of (v12, 'F-4'-0)], [37], 0,000000). p (40,2,3, pp, [объект (v10, v12), a_kind_of (v12, 'F-4'-0)], [[24,29]], 0,009611). Рисунок 3: Диаграмма, полученная в результате анализа «капсулы на F-4».Ввод 215669:
«тп 1314.Система эвакуации а-7б / э двт-7 (250 кэас) (прогон 2). синхронная стрельба на расстоянии 1090 футов x 38 футов ширины. манекен только что покидает сани «.
Список значений, рассчитанный MARIE-2 :
[a_kind_of (v1, "TP-1314" -0), во время (v3, v1), a_kind_of (v3, "escape system" -0), во время (v3, v4), a_kind_of (v4, "RUN 2" -0), агент (v8, v3), a_kind_of (v8, "DVT-7" -0), измерение (v8, v2), a_kind_of (v2, "250 keas" -0), количество (v2,250), единицы (v2, keas), объект (v8, v5), a_kind_of (v5, "A-7B / E" -0), во время (v141, v1), a_kind_of (v141, launch-2), property (v141, synchronous-51), at (v141, v95), a_kind_of (v95, место-8), part_of (v45, v95), part_of (v46, v95), a_kind_of (v45, "1090''n" -0), количество (v45,1090), единицы (v45, "широта-минута" -0), a_kind_of (v46, "38 '' w" -0), количество (v46,38), единицы (v46, "долгота-минута" -0), в течение (v999, v1), a_kind_of (v999, dummy-3), agent (v1012, v999), a_kind_of (v1012, leave-105), время (v1012, prespart), свойство (v1012, just-154), объект (v1012, v1039), a_kind_of (v1039, санки-1)]Рисунок 10: Анализ времени процессора и количества попыток до наилучшего интерпретация найдена для восьми предложений-примеров.Информация о суперконцепте для смысла слова :
«ТП-1314» -0 -> тест-3, «система эвакуации» -0 -> система-8, «ДВТ-7» -0 -> тест-3, "250 keas" -0 -> номер-7, "A-7B / E" -0 -> истребитель-4, "RUN 2" -0 -> run-1, стрелять-109 -> сброс-105, место-8 -> "географическая зона" -1, "1090 'n" -0 -> число-6, "38' w" -0 -> число-6, синхронный-51 -> "одновременно" -51, манекен-3 -> цифра-9, левая-105 -> го-111, салазки-1 -> машина-1
Список значений, рассчитанный MARIE-1 :
[inst ('существительное (215669-4-2)', сани), inst ('существительное (215669-2-a68)', 'A-7B / E'), attribute ('существительное (215669-2-a67)', part_of ('существительное (215669-2-a68)')), inst ('существительное (215669-2-a67)', 'DVT-7'), agent ('prespart (215669-4-1)', obj ('существительное (215669-4-1)')), источник ('prespart (215669-4-1)', obj ('существительное (215669-4-2)')), активность ('prespart (215669-4-1)', отъезд), attribute ('координата (215669-3-1)', '1090' 'N x 38' 'W'), inst ('координата (215669-3-1)', координата), атрибут ('существительное (215669-2-6)', '2'), theme ('существительное (215669-2-6)', obj ('существительное (215669-2-5)')), inst ('существительное (215669-2-6)', запустить), тема ('существительное (215669-2-5)', obj ('существительное (215669-2-a67)')), количество ('существительное (215669-2-5)', keas ('250')), inst ('существительное (215669-2-5)', тест), атрибут ('существительное (215669-3-1)', синхронно), location ('существительное (215669-3-1)', at ('координата (215669-3-1)')), активность ('существительное (215669-3-1)', запуск), inst ('существительное (215669-4-1)', пустышка), attribute ('существительное (215669-1-1)', '1314'), inst ('существительное (215669-1-1)', 'план тестирования')]
Рисунок 4: Пример вывода синтаксического анализатора, плюс суперконцепции для используемых смыслов слов.
Учебный набор 1 Набор 2 Набор 3 Количество новых титров 217 108 112 Количество новых предложений 444 219 140 Общее количество слов в новых подписях 4488 1774 1060 Количество отдельных слов в новых подписях 939 900 418 Количество необходимых новых словарных статей ок. 150 106 78 Количество использованных новых значений слов 929 728 287 Количество используемых новых сенсорных пар 1860 1527 542 Количество необходимых изменений лексической обработки c.30 11 7 Количество изменений или дополнений синтаксических правил 35 41 18 Количество изменений или дополнений в определение случая 57 30 11 Количество изменений или дополнений семантических правил 72 57 15Рисунок 5: Общая статистика по обучающим выборкам.
Рисунок 6: Журнал времени анализа в зависимости от журнала длины предложения.
Рисунок 7: Журнал среднего геометрического времени анализа по сравнению с логарифмом предложения длина. Пунктирная линия - первая тренировочная выборка, жирная линия - вторая, и тонкая линия третья.
Рисунок 8: Журнал среднего геометрического числа попыток до исправления интерпретация была найдена по сравнению с логарифмом длины предложения. Пунктирная линия - первая тренировочная выборка, жирная линия - вторая, и тонкая линия третья.1 Тихоокеанский полигон и цех, санные трассы. Характеристики 2-х пневмоприводов, указателя и подсистемы стабилизации. 3 вакуумные камеры работают в цехе лазерного разрушения. 4 средство для ранней подготовки флота: Sidewinder 1 в разрезе секции наведения. В ожидании реставрации 5: модель спутника исследователя в хранилище артефактов.6 fae i (cbu-72), одно из семейства топливно-воздушных взрывных устройств китайского озера. 7 широкополосных радиолокационных сигнатурных испытаний мачты подводной связи в бистатическая безэховая камера. 8 освещающая антенна расположена низко на вертикальной башенной конструкции. а приемная антенна расположена вверху.Рисунок 9: Примеры предложений.Отправлено. Финал обучения Нет двоичного Нет унарного число попыток времени попыток времени попыток времени 1 27,07 13 17,93 5 8.27 5 60,63 19 2 70,27 10 48,77 9 94,62 14 124,9 23 3 163,0 19 113,1 19 202,9 23 2569,0 22 4 155,2 9 96,07 3 63,95 8 229,3 22 5 86,42 8 41,02 3 49,48 6 130,6 30 6 299,3 11 65,78 7 68,08 5 300,4 15 7 1624,0 24 116,5 5 646,0 12 979,3 25 8 7825.0 28 35.02 2 35.60 3> 50000-
Перейти к бумажному указателю
Локализация | X-Plane
Требования к локализации
Перед тем, как приступить к работе по локализации X-Plane, пожалуйста, ознакомьтесь с этими требованиями! Мы не хотим, чтобы вы тратили много времени на локализацию, а затем осознавали наличие проблемы.
- LR не может использовать ваши переводы. Мы постараемся использовать любой полученный перевод или заранее уведомим вас, если не сможем, но мы не можем гарантировать, что ваш перевод попадет в X-Plane.
- Мы не можем откладывать выпуски для переводов; если строка изменяется, и мы должны вырезать RC, и вы не можете перевести строку, строка может остаться непереведенной.
- LR должен владеть IP-адресами всех переводов (которые являются производными от самого X-Plane). Мы не принимаем переводы с «привязанными условиями».
- Если над языком работают более одного человека, вы должны сотрудничать с другими членами команды!
Общая стратегия локализации
Система локализации использует сопоставление ключевой строки; для каждого языка текстовый файл словаря сопоставляет английские фразы с фразами на локализованном языке.
(Это отличается от старой системы локализации, используемой для всех версий v8, которая сопоставляет числа со строками либо на английском, либо на локализованном языке; в старой системе, чтобы узнать, какой должна быть строка 511, вам нужно было проверить английский.)
Существует один файл словаря для каждого языка на файл.
Формат файла словаря
Расположение файла
Файлы словарей хранятся в Resources / text /
Монтажник Специальное обращение
Поскольку программа установки не использует папку «Ресурсы», файлы локализации встроены в приложение. Вы можете просмотреть и заменить строковый файл для установщика Macintosh, выбрав «показать содержимое пакета» — словарь с именем
Наборы символов и кодировка файлов
Файлы словаря должны быть закодированы в UTF8 без маркера порядка байтов (BOM). Используйте любой символ Юникода из следующих стандартных диапазонов Юникода (за исключением непечатаемых / управляющих символов):
- Базовая латиница (0x00-0x7F)
- Дополнение к Latin-1 (0x80-0xFF)
- Расширенная латиница-A (0x100-0x17F)
- Расширенная латиница-B (0x180-0x1FF)
- Кириллица (0x400-0x4FF)
Текстовые файлы должны использовать окончания строк Unix.
Синтаксис словаря
Заголовок файла словаря:
A
900
ПЕРЕВОД
Записи
За заголовком следует серия записей, по одной в строке.
Строка, начинающаяся с файла #, является комментарием.
Строка, начинающаяся с лексемы STRING, за которой следует хотя бы один пробел, определяет пару строковых ключей. Формат пары ключей:
STRING <пробел> <английский> ==== <локализованный>
Например:
STRING Проверить дисковое пространство ==== Festplattenplatz prüfen
Предупреждение : интервал очень важен при создании строковых макросов.Один пробел после слова STRING расходуется на синтаксический анализ; все остальные пробелы являются частью строк. Примеры постоянного интервала:
STRING Проверить дисковое пространство ==== Festplattenplatz prüfen
STRING Проверить дисковое пространство ==== Festplattenplatz prüfen
STRING Проверить дисковое пространство ==== Festplattenplatz prüfen
STRING Проверить дисковое пространство ==== Festplattenplatz prüfen
Обратите внимание, что в последних двух параметрах после слова prüfen стоит конечный пробел, но перед новой строкой. В этих примерах несогласованный интервал:
STRING Проверить дисковое пространство ==== Festplattenplatz prüfen
STRING Проверить дисковое пространство ==== Festplattenplatz prüfen
Система замены
Когда X-Plane должен подставить слово в строку, он делает это с помощью параметра.Параметры имеют вид:
% <число>: <комментарий>%
, где число — целое положительное число. Число определяет, какой параметр будет заменен. В каждой фразе может быть более одного параметра.
Комментарий — это слово, примерно описывающее, что входит в параметр; он не используется X-Plane (ни в английской, ни в переведенной строке), а просто предназначен для предоставления контекста при переводе. Например:
STRING Возникла проблема с% 1% ====…
может быть почти невозможно перевести.Но
STRING Возникла проблема с% 1: имя файла% ====….
дает подсказку. Вы можете изменить порядок параметров, и вы можете изменить комментарий в переведенной версии, но вы не можете изменить имя комментария на английской стороне, иначе X-Plane не найдет строку. Пример:
STRING Произошла ошибка при открытии% 1: filename% -% 2: message% ==== Операционная система сообщила «% 2: msg%», когда мы попытались открыть% 1: file%.
Обратите внимание, что в этом примере комментарии были немного переименованы и порядок параметров изменен, но сообщение по-прежнему переходит в параметр 2, а имя файла — в параметр 1.
Замещение и множественное число
Если в X-Plane есть фраза, которая может меняться во множественном числе (например, одна ошибка или две ошибки), в файле перевода будут две строки:
STRING При установке произошла ошибка% 1: count%. ====
STRING При установке было% 2: count% ошибок. ====
Эти множественные числа почти всегда будут смежными (см. Отрывки ниже). Сим будет правильно выбирать правильное множественное число с учетом языковых правил об единственном и множественном числе.(Обратите внимание, что X-Plane еще не может обрабатывать языки, в которых существует более двух падежей для единственного / множественного числа.
Если вы хотите локализовать X-Plane, лучше всего получить отрывок строки из Laminar Research. Это словарь, содержащий все английские фразы в симуляторе и связанных с ним приложениях.
Экстракты соответствуют стандартному формату словаря и бывают двух основных видов:
Несортированные отрывки содержат каждую строку один раз в том порядке, в котором они появляются в коде, с множественным числом сразу после единственного числа и комментариями к переводу.(Это комментарии программистов переводчикам, указывающие на использование строки). Находясь в порядке кода, несортированные отрывки обычно помещают связанные строки в одном месте, например все строки веб-средства обновления будут в кластере.
Несортированный отрывок — лучшая основа для перевода.
Отсортированный отрывок сортирует файл в алфавитном порядке и удаляет комментарии; отсортированный экстракт можно легко «сравнить» с более старым отсортированным экстрактом, чтобы быстро идентифицировать все измененные строки.Используйте эти экстракты только для определения того, что изменилось с момента предыдущего извлечения.
Параметры командной строки и журнал
Всегда проверяйте файл журнала при переводе; ошибки в ваших файлах переводов будут отмечены!
Вы можете использовать опцию командной строки: –missing_strings
, чтобы X-Plane выводил строку STRING ==== один раз для каждой отсутствующей строки в вашем переводе. Это хороший способ создать словарные статьи для недостающих строк.
Вернуться к базе знаний
html — RegEx соответствует открытым тегам, кроме автономных тегов XHTML
Я согласен с тем, что правильный инструмент для синтаксического анализа XML и , особенно HTML , — это синтаксический анализатор, а не механизм регулярных выражений.Однако, как отмечали другие, иногда использование регулярного выражения быстрее, проще и выполняет свою работу, если вы знаете формат данных.
На самом деле Microsoft имеет раздел «Рекомендации по использованию регулярных выражений в .NET Framework», в котором конкретно говорится о рассмотрении входного источника.
У регулярных выраженийесть ограничения, но учли ли вы следующее?
Платформа .NET уникальна, когда дело доходит до регулярных выражений, тем, что она поддерживает определения балансирующих групп.
По этой причине я считаю, что вы МОЖЕТЕ анализировать XML, используя регулярные выражения. Однако обратите внимание, что должен быть действительным XML (браузеры очень снисходительны к HTML и допускают неправильный синтаксис XML внутри HTML ). Это возможно, поскольку «Определение балансирующей группы» позволит обработчику регулярных выражений действовать как КПК.
Цитата из статьи 1, цитированной выше:
Механизм регулярных выражений .NET
Как описано выше, правильно сбалансированные конструкции не могут быть описаны регулярное выражение.Однако механизм регулярных выражений .NET предоставляет несколько конструкций, которые позволяют сбалансированным конструкциям быть признал.
(?
— помещает полученный результат в стек захвата с помощью название группы.) (? <-group>)
— выводит самый верхний захват с группой имен за стек захвата.(? (Group) yes | no)
— соответствует части yes, если существует группа с группой имен в противном случае не соответствует ни одной части.Эти конструкции позволяют регулярному выражению .NET имитировать ограничил КПК, по существу, разрешив простые версии стека операции: push, pop и empty. Простые операции в значительной степени эквивалентно увеличению, уменьшению и сравнению с нулем соответственно. Это позволяет механизму регулярных выражений .NET распознавать подмножество контекстно-свободных языков, в частности тех, которые только требуется простой счетчик. Это, в свою очередь, позволяет использовать нетрадиционные .<>] * ) * (? (opentag) (?!))
Используйте флаги:
- Однолинейный
- IgnorePatternWhitespace (необязательно, если вы сворачиваете регулярное выражение и удаляете все пробелы)
- IgnoreCase (не обязательно)
Объяснение регулярного выражения (встроенное)
(? =
) # совпадение начинается с
# атомная группа / не возвращаться (быстрее) ] * # что-то между тегами ) * # сопоставить как можно больше тегов xml (? (opentag) (?!)) # убедитесь, что в стеке нет групп 'opentag'
Вы можете попробовать это на сайте A Better .NET Regular Expression Tester.
Я использовал образец источника:
- прочее ...
- другие материалы
еще больше
- Еще один & gt; ul & lt; боже мой!
- ...
Это найдено совпадение:
- прочее ...
- другие материалы
еще больше
- Еще один & gt; ul & lt; боже мой!
- ...
, хотя на самом деле получилось так:
- материал ...
- еще материал
еще больше
- Еще один & gt; ul & lt; боже мой!
- ...
Наконец, мне очень понравилась статья Джеффа Этвуда: Parsing Html The Cthulhu Way. Как ни странно, он приводит ответ на этот вопрос, за который в настоящее время проголосовало более 4 тысяч человек.
NLP: Создание текстового сумматора с использованием глобальных векторов | автор: Динеш Ядав
Резюме статьи — это сокращенная версия, содержащая ключевые моменты, чтобы сжать ее до нескольких пунктов и сделать это словами, используемыми в самой статье.В основном, извлекаются только те элементы / предложения, которые мы считаем наиболее важными, как правило, которые передают основную идею или существенные опорные пункты.
Резюме — это не анализ статьи. Оба разные вещи. Обобщение полезно во многих ситуациях, например, чтобы понять основные моменты большой статьи, представить сложную идею с помощью горячих слов, получить смысл из большой статьи и т. Д.
С академической точки зрения извлечение резюме — это немного сложная задача. К счастью, на помощь приходит машинное обучение.Модуль обработки естественного языка (NLP) машинного обучения предлагает множество алгоритмов, которые можно использовать для резюмирования текста. Существует 2 основных подхода к обобщению текста:
Абстрактивное обобщение:
В этом методе используются передовые методы НЛП для создания резюме, которое является совершенно новым с точки зрения используемых слов / предложений. Это означает, что резюме составляется словами, не используемыми в статье.E xtractive Summarization:
В этом методе наиболее важные слова / предложения извлекаются и группируются вместе для создания резюме.Итак, слова / предложения, используемые в резюме, взяты из самой статьи.В этой статье, используя экстрактивную технику, мы создадим краткую сводку новостей для извлечения 4–5 основных важных предложений из большой новостной статьи. Мы проверим некоторые из популярных и эффективных стратегий обработки большого количества текста и извлечения из него 4–5 значимых предложений.
Мы будем использовать глобальные векторы, также известные как GloVe-алгоритмы, которые представляют собой векторные представления слов. Говоря простым языком, мы будем генерировать векторы предложений с использованием алгоритмов GloVe и выбирать лучшие предложения для алгоритма ранжирования страницы.Без лишних слов, давайте углубимся в код. Для этого упражнения я использовал питон.
Я предпочитаю работать с RSS-потоком TimeOfIndia, одной из самых популярных новостных служб в Индии. Для этого упражнения я выбрал «мировой» раздел новостей. Но он достаточно гибкий, чтобы обрабатывать несколько RSS-каналов различных новостных служб.
Давайте прочитаем RSS-канал и передадим ссылку на новость BeautifulSoup для разбора HTML. Обратите внимание, что здесь я взял только один RSS-канал и сделал пошаговый синтаксический анализ.Позже я объединил эти шаги вместе, чтобы без проблем обрабатывать несколько каналов.
# import basic required libraries
import pandas as pd
import numpy as np
import os # для Интернета и HTML
запросов на импорт
из bs4 import BeautifulSoup # создайте dict различных ссылок RSS-каналов и их категорий. Буду повторять их один за другим.
# В демонстрационных целях упомянут только один канал.
timesofindia = {'world': 'http: //timesofindia.indiatimes.com/rssfeeds/296589292.cms '}
для категории, rsslink в timesofindia.items ():
print (' Обработка для категории: {0}. \ nRSS-ссылка: {1} '. format (category, rsslink))
# получить URL-адрес веб-страницы и прочтите html
rssdata = requests.get (rsslink)
#print (rssdata.content)
soup = BeautifulSoup (rssdata.content)
print (soup.prettify ())После синтаксического анализа BeautifulSoup необходимо проверить HTML-содержимое веб-страницы полностью (используя функцию prettify, как указано выше), чтобы найти тег / шаблон или последовательность тегов для навигации, чтобы получить желаемый заголовок новости, ссылку и pubDate.В нашем случае эти элементы находятся внутри тега «item». Итак, давайте извлечем его, чтобы пройти по каждому тегу «item» и извлечь каждый отдельный элемент.
# получать все новости. У него есть заголовок, описание, ссылка, руководство, дата публикации для каждой новости.
# Давайте назовем эти элементы, и мы будем перебирать их. -item #: ', item)
title = allitems [item] .title.текст
ссылка = allitems [элемент] .guid.text
pubdate = allitems [элемент] .pubdate.text
print ('TITLE:', title)
print ('LINK:', link)
print ('PUBDATE:' , pubdate)Вывод:
Всего найдено новостей: 20
НАЗВАНИЕ: Беременная невеста Бориса Джонсона говорит, что она «выздоравливает» от коронавируса
ССЫЛКА: https: //timesofindia.indiatimes.com/world / ...
PUBDATE: Sun, 05 Apr 2020 17:15:04 IST
НАЗВАНИЕ: США доставят по воздуху 22 000 американцев, застрявших за границей; многие в Индии
ССЫЛКА: https: // timesofindia.indiatimes.com/world / ....
ПУБДАТА: вс, 5 апр 2020 14:08:04 IST
НАЗВАНИЕ: вице-президент Эквадора приносит свои извинения за трупы вирусов, оставленные на улицах
ССЫЛКА: https://timesofindia.indiatimes.com/world / ....
PUBDATE: Sun, 05 Apr 2020 14:01:42 ISTОбязательные элементы, то есть заголовок, ссылка, дата публикации выглядят ожидаемыми. Перейдем к следующему разделу, где мы создадим базовую функцию для получения текста новостной статьи по ссылке.
В этом разделе мы извлечем текст новостной статьи, проанализировав HTML-ссылку веб-страницы.По ссылке, полученной из RSS-канала, мы извлечем веб-страницу и проанализируем ее с помощью BeautifulSoup.
HTML-код веб-страницы должен быть тщательно проанализирован, чтобы определить теги, в которых доступен желаемый текст новости. Я создал базовую функцию для получения текста новостей по ссылке. Используя BeautifulSoup, извлечет текст новостей, доступный в определенных тегах html.
# Функция для получения каждой ссылки на новости, чтобы получить эссе новостей
def fetch_news_text (link):
# прочитать веб-страницу html и проанализировать ее
soup = BeautifulSoup (requests.get (link) .content, 'html.parser') # получить текстовое поле новостной статьи
# это с элементом
text_box = soup.find_all ('div', attrs = {'class': '_ 3WlLe clearfix '})
# извлекаем текст и объединяем
news_text = str ("." .Join (t.text.strip () for t in text_box))
return news_text # используя вышеуказанную функцию, обрабатываем текст
news_articles = [{' Feed ':' timesofindia ',
' Категория ': категория,
' Заголовок ': allitems [item] .title.text,
' Link ': allitems [item] .guid.text,
'Pubdate': allitems [item] .pubdate.text,
'NewsText': fetch_news_text (allitems [item] .guid.text)}
для элемента в диапазоне (len (allitems))] news_articles = pd.DataFrame (news_articles)
news_articles.head (3) Детали новостных статей после получения текста новостиПриведенные выше данные выглядят так, как ожидалось. Обратите внимание, что извлечение текста новостей зависит от указанных тегов HTML, поэтому, если поставщик новостей изменит / модифицирует теги, указанное выше извлечение может не работать. Чтобы справиться с этим, мы можем поставить предупреждение / флаг уведомления всякий раз, когда текст новости имеет значение NULL, указывая время для изменения кода / тега.
Для очистки текста я использую шаги предварительной обработки, подробно описанные в этой статье . Эти шаги включают удаление HTML-тегов, специальных символов, чисел, знаков препинания, стоп-слов, обработки акцентированных символов, раскрывающих сокращений, выделения корней и лемматизации и т. Д. Эти шаги подробно описаны в этой статье , так что ознакомьтесь с подробностями.
Здесь я объединил эти шаги предварительной обработки в единую функцию, которая вернет чистый и нормализованный корпус.
# test normalize cleanup для одной статьи
# clean_sentences = normalize_corpus ([news_articles ['NewsText'] [0]])
clean_sentences = normalize_corpus (news_articles ['NewsText'])Используя вложения слов GloVe, мы сгенерируем векторные вложения слов представление предложений. Для этого упражнения я использую предварительно обученные векторы Wikipedia 2014 + Gigaword 5 GloVe, доступные здесь .
# define dict для хранения слова и его вектора
word_embeddings = {} # чтение файла вложений слов ~ 820MB
f = open ('.\\ GloVe \\ glove.6B \\ glove.6B.100d.txt ', encoding =' utf-8 ')
для строки в f:
values = line.split ()
word = values [0]
coefs = np.asarray (values [1:], dtype = 'float32')
word_embeddings [word] = coefs
f.close () # проверяем длину
len (word_embeddings) # 400000В этом наборе у нас 400K вложение слов. Размер этих вложений слов составляет 822 МБ. Размер может варьироваться в зависимости от встраиваемых токенов. Чем больше встраивание, тем выше точность. Используя встраивание этих слов, давайте создадим векторы для наших нормализованных предложений.Для предложения мы сначала извлечем векторы для каждого слова. Затем будет взята средняя оценка всех векторных оценок слов предложения, чтобы получить консолидированную векторную оценку для предложения.
# создать вектор для каждого предложения
# список для хранения вектора
предложение_vectors = [] # создать вектор для каждого чистого нормализованного предложения
для i в clean_sentences:
if len (i)! = 0:
v = sum ([word_embeddings .get (w, np.zeros ((100,))) для w в i.split ()]) / (len (i.split ()) + 0.001)
иначе:
v = np.zeros ((100,))
offer_vectors.append (v) print ('Всего создано векторов:', len (offer_vectors))
Векторы — это просто указатель на плоскости. Используя подход косинусного сходства, найдет сходство между предложениями. Векторы с низким углом косинуса будут встречать как более похожие. Оценка косинуса рассчитывается для предложения с каждым другим предложением в статье. Здесь также могут использоваться другие подходы, такие как евклидово расстояние, и векторы с меньшим расстоянием между ними будут более похожими.
из sklearn.metrics.pairwise import cosine_similarity # определить матрицу со всеми нулевыми значениями
sim_mat = np.zeros ([len (предложения), len (предложения)]) # заполнит ее значениями cosine_similarity
# для каждого предложения по сравнению с другое
для i в диапазоне (len (предложения)):
для j в диапазоне (len (предложения)):
, если i! = j:
sim_mat [i] [j] = cosine_similarity (предложения_вектора [i] .reshape ( 1,100), offer_vectors [j] .reshape (1,100)) [0,0]Затем давайте преобразуем эту косинусную матрицу сходства в граф, где узлы будут представлять предложения, а ребра будут представлять оценки сходства между предложениями.На этом графике будет применяться алгоритм PageRank для определения рейтинга каждого предложения.
импортировать networkx как nx # построить график и получить pagerank
nx_graph = nx.from_numpy_array (sim_mat)
scores = nx.pagerank (nx_graph) # распечатать окончательные значения предложений
оценокВывод:
12 {01796512 {017965126 ,
1: 0,0642861521750098,
2: 0,06399116048715114,
3: 0,06432009432128397,
4: 0,06385988469675835,
5: 0,06400525631019922,
6: 0.06520921510891638,
7: 0,06320537732857809,
8: 0,06298228524215846,
9: +0,06399491863786076,
10: 0,0640726538022174,
11: +0,06349704017361839,
12: +0,06357060319536506,
13: +0,057627597033478764,
14: 0,058463972076477785,
15: +0,05173217723913434}Так как у нас было 16 предложений в нашей первоначальной статье, то есть мы получили 16 баллов, то есть по одному за каждое предложение. Пришло время выбрать N лучших предложений на основе рейтинга, рассчитанного выше.
Как видно выше, окончательный текст требует некоторой обработки, прежде чем он может быть представлен.Эта обработка может заключаться в использовании заглавных букв в первом символе каждого предложения, удалении названия местоположения из начала каждой статьи, удалении лишних пробелов / табуляции / пунктуации, исправлении разрывов строк и т. Д.
Наконец, мы можем объединить все эти шаги, чтобы создать механизм суммирования. / скрипт. Этот сценарий можно запланировать для запуска на выбранных RSS-каналах каждое утро и отправки сводки новостей на ваш почтовый ящик. Таким образом, вам не нужно просматривать всю статью, чтобы быть в курсе последних событий. Или вы можете создать красивую HTML-страницу / виджет для отображения сводки новостей по ведущим сообщениям.Обратите внимание, что выше я использовал один RSS-канал, но при создании конвейера можно указать еще несколько RSS-каналов. Кроме того, я использовал некоторые операторы печати для отображения промежуточных значений, которые можно удалить, чтобы получить удобную работу.
Надеюсь, вам понравилась эта статья. Если вы хотите поделиться какими-либо предложениями по любому подходу, не стесняйтесь говорить об этом в комментариях. Отзывы / комментарии читателей всегда вдохновляют писателя.
Неисправность двигателя могла стать причиной авиакатастрофы в Пакистане, говорят эксперты, но официальной информации пока нет
Рейс авиакомпании «Пакистанские международные авиалинии» (PIA) из Лахора в Карачи врезался в жилую колонию недалеко от международного аэропорта Джинна.| Twitter | @ANIРазмер текста: А- А +
Бангалор: Это была двойная трагедия в пятницу, когда рейс Пакистанских международных авиалиний (PIA) из Лахора в Карачи врезался в жилую колонию как раз перед тем, как приземлиться.
Авария с участием рейса PK8303 произошла незадолго до праздника Курбан-байрам, когда Пакистан начал возобновлять работу внутренних авиалиний после блокировки, введенной для сдерживания распространения Covid-19.
Хотя двое из 99 пассажиров самолета, включая членов экипажа, чудом остались живы, поступили сообщения о множественных жертвах на земле.
Спустя 24 часа после авиакатастрофы авиационные эксперты сообщили ThePrint, что опасаются обсуждать ее точную причину, заявив, что данные с бортовых самописцев еще не получены, и что еще слишком рано что-либо предлагать.
Однако энтузиасты-любители авиации и форумы экспертов цитировали изображения самолета с момента его последнего полета и непроверенные записи разговора пилота с диспетчером воздушного движения (УВД), чтобы сделать определенные выводы.
Они утверждали, что самолет не смог развернуть шасси, и оба его двигателя, похоже, вышли из строя.
По словам одного из двух оставшихся в живых, самолет Airbus A320-214, построенный в 2004 году и находившийся в эксплуатации PIA с 2014 года, совершал вторую попытку приземления, когда потерпел крушение.
Также читайте: Неправильный взлет был упущенной возможностью предотвратить крушение Lion Air
Теории на ходуСамолет, который потерпел крушение, был приобретен PIA у ирландско-американского авиационного гиганта GE Capital Aviation Services (GECAS) в аренду без обслуживания сроком на шесть лет.
Рейс PK8303 потерпел крушение около 14.45 по местному времени, примерно в то время, когда он должен был приземлиться в международном аэропорту Джинна в Карачи после менее чем двухчасового полета из Лахора.
Сообщается, что в субботу представитель PIA подтвердил, что регистратор полетных данных и диктофон кабины были обнаружены с места крушения.
В то время как регистраторы были переданы властям, контролирующим расследование авиакатастрофы, энтузиасты авиации анализировали изображения и видеозаписи последних моментов полета, чтобы сделать определенные предположения о причине.
Предполагается, что самолет совершил попытку приземления и разбился во время второй попытки.
Представитель гражданской авиации заявил, что у самолета могли возникнуть проблемы с опусканием шасси — колес и другого оборудования, которое помогает при посадке и взлете — из-за технической неисправности. Изображения, размещенные в социальных сетях, указывают на то, что выглядит как следы ожога под двигателями без опущенного шасси.
Перед аварией самолет послал сигнал бедствия, который на авиационном языке переводится как сигнал бедствия, согласно предполагаемой аудиозаписи последних нескольких минут разговора между одним из пилотов и УВД.Запись была размещена на сайте мониторинга авиации atc.net, а также разошлась в социальных сетях.
https://twitter.com/HinaRKharal/status/1263788851069419522
Первый заход самолета на взлетно-посадочную полосу был прерван из-за проблем с выдвижением передней стойки шасси, говорится в сообщении об аварии в The Aviation Herald , которое публикует отчеты по вопросам, связанным с авиацией.
На записи, которая еще не подтверждена, слышно, как пилот говорит, что в полете «потерял двигатель» или «потеряли двигатели» (пока неясно, что именно он говорил).
Когда УВД спросил, собираются ли они совершить «посадку на живот», он ответил: «Первомай, Первомай, Первомай!»
Согласно записи, до этого диспетчер УВД разрешил полет на 3000 футов, а затем предупредил пилотов о том, что высота полета снижается. Запись также отражает повторяющийся фоновый «дребезжащий» звук, который, по словам экспертов, срабатывает, когда возникают проблемы с выдвижением шасси.
Еще один фактор, на который ссылаются эксперты в поддержку теории отказа двигателя, — это то, что на снимках показана развернутая воздушная турбина (RAT) во время полета.
RAT — это ветряная турбина, которая обычно запускается автоматически, если оба двигателя выходят из строя, поэтому она может генерировать энергию за счет вращающихся лопастей. Самолеты-любители также утверждали, что заметили немного дыма, исходящего от двигателей.
Наши сердца оплакивают ужасные новости, приходящие сегодня из Карачи. Вот AP-BLD в его заключительных моментах, пытаясь уйти после первой попытки приземления на живот. Небольшой дым можно увидеть и от двигателей. # PlaneSpottersPakistan #avipak #planecrash #karachi pic.twitter.com/eOKiCAeRu7
— Plane Spotters Pakistan (PSPK) (@PlaneSpottersPK) 22 мая 2020 г.
Согласно сообщению журнала Guardian , самолет был оснащен двигателями производства CFM International, совладельцами которых являются General Electric и французская Safran.
Один из выживших, Мухаммад Зубайр, сказал, что между первой и последующей попыткой приземления был 10-15-минутный перерыв, заявив, что полет прошел гладко, без каких-либо признаков неминуемой катастрофы.
Другим выжившим пассажиром был Зафар Масуд, президент Банка Пенджаба.
Также читайте: Катастрофа самолета в Катманду последняя из многих авиационных трагедий в Непале
Подпишитесь на наши каналы в YouTube и Telegram
Почему СМИ переживают кризис и как его исправить
Индии еще больше нужна свободная, справедливая, без дефисов и вопросов журналистика, поскольку она сталкивается с множеством кризисов.
Но средства массовой информации переживают собственный кризис. Произошли жестокие увольнения и сокращения зарплат. Лучшее в журналистике сжимается, уступая место грубому зрелищу в прайм-тайм.
ВThePrint работают лучшие молодые репортеры, обозреватели и редакторы. Для поддержания журналистики такого качества нужны умные и думающие люди вроде вас, чтобы за это платить. Живете ли вы в Индии или за границей, вы можете сделать это здесь.
Поддержите нашу журналистику
Как правильно выбрать слово
Слова «простой» и «плоский» являются омофонами, что означает, что они звучат одинаково, но имеют разные значения.«Обычный» может быть существительным, прилагательным или наречием, а «плоскость» может быть существительным или глаголом. Хотя оба слова могут относиться к плоскостности, одно используется для описания географии, а другое — для описания геометрии.
Как использовать «Обычный»
В качестве прилагательного «простой» относится ко всему простому, несложному, распространенному или очевидному. Существительное «равнина» относится к плоскому, обычно безлесному участку земли. Равнины являются одной из основных форм рельефа в мире и необходимы для крупномасштабного сельского хозяйства.Одним из самых известных примеров является Атлантическая прибрежная равнина, которая простирается вдоль восточного побережья Соединенных Штатов.
В качестве наречия «простой» действует как усилитель, подчеркивая то, что обычно является отрицательным качеством (например, «это было просто простой тупой»).
Как использовать «Самолет»
«Самолет» — это существительное, которое может относиться к самолету, инструменту для выравнивания дерева или ровной поверхности. В геометрии «плоскость» означает любую двумерную поверхность, бесконечно простирающуюся в пространстве.Эта фигура может быть определена тремя точками, которые не попадают на одну линию, линией и одной точкой, которая не попадает на эту линию, двумя пересекающимися линиями или двумя параллельными линиями.
Глагол «плоскость» относится к сглаживанию или созданию ровной поверхности с использованием плоскости .
В религии и эзотерических учениях «план» иногда относится к состоянию или уровню сознания или бытия. В буддизме, например, говорится, что существует 31 план существования , простирающийся от состояний лишения до бесформенных царств бесконечного пространства и бесконечного сознания.В оккультной философии считается, что душа переходит на астральный план после смерти. Розенкрейцерство — мистическая традиция, возникшая в 1600-х годах — утверждает, что духовный мир разделен на семь космических планов .
Примеры
«Обычный» — это почти всегда прилагательное, используемое для модификации существительных, основное качество которых состоит в том, что они обычные и неотличимые:
- Он хотел только простой буханку хлеба из пекарни.
- На девушке было простое черное платье без каких-либо оборок и украшений.
- Несмотря на свое простое лицо , он в одночасье стал звездой YouTube.
Как существительное, «равнина» относится конкретно к области плоской земли, такой как луг, луга или прерии, на которой мало или совсем нет деревьев:
- Коровы паслись на равнине .
- Путешествие прошло гладко, когда они достигли равнины Канзаса равнины .
«Самолет» — тоже существительное, но оно может означать множество вещей, от летательного аппарата, используемого для путешествий по воздуху, до плоской поверхности:
- Генеральный директор и еще несколько человек летели на небольшом частном самолете .
- Он зашлифовал стол до тех пор, пока он не стал полностью гладким. плоскость .
В своем духовном или религиозном контексте «план» обычно относится к состоянию бытия или осознанности:
- После нескольких лет медитации она начала чувствовать, что ее разум достиг более высокого уровня .
Как запомнить разницу
Легко спутать «простой» и «плоский», особенно потому, что как существительные они оба относятся к плоскостности. Один из способов запомнить разницу заключается в том, что «pl ai n» пишется с «ai», например «tr ai n», а поезда предназначены для движения по гладким поверхностям, например равнинам. «Самолеты», с другой стороны, часто бывают концептуальными или теоретическими — например, геометрические плоскости или плоскости духовного просветления.Другими словами, «pl a ne» с «a» часто означает a bstract.
Источники
.
- Касагранде, июнь. «Радость синтаксиса: простое руководство по всей грамматике, которую вы знаете, что должны знать». Ten Speed Press, 2018.
- Мансер, Мартин Х. «Хорошее слово». A&C Black, 2007.