What does npm exec do? What is the difference between «npm exec» and «npx»?
What are the building blocks of OWL ontologies?
Learn more about «RDF star», «SPARQL star», «Turtle star», «JSON-LD star», «Linked Data star», and «Semantic Web star».
The Hadamard gate is one of the simplest quantum gates which acts on a single qubit.
Learn more about the bra–ket notation.
Progressive Cactus is an evolution of the Cactus multiple genome alignment protocol that uses the progressive alignment strategy.
The Human Genome Project is an ambitious project which is still underway.
What are SVMs (support vector machines)?
Find out more in Eckher’s article about TensorFlow.js and linear regression.
On the importance of centralised metadata registries at companies like Uber.
Facebook’s Nemo is a new custom-built platform for internal data discovery. Learn more about Facebook’s Nemo.
What is Data Commons (datacommons.org)? Read Eckher’s introduction to Data Commons (datacommons.org) to learn more about the open knowledge graph built from thousands of public datasets.
Learn more about how Bayer uses semantic web technologies for corporate asset management and why it enables the FAIR data in the corporate environment.
An introduction to WikiPathways by Eckher is an overview of the collaboratively edited structured biological pathway database that discusses the history of the project, applications of the open dataset, and ways to access the data programmatically.
Eckher’s article about question answering explains how question answering helps extract information from unstructured data and why it will become a go-to NLP technology for the enterprise.
Read more about how document understanding AI works, what its industry use cases are, and which cloud providers offer this technology as a service.
Lexemes are Wikidata’s new type of entity used for storing lexicographical information. The article explains the structure of Wikidata lexemes and ways to access the data, and discusses the applications of the linked lexicographical dataset.
The guide to exploring linked COVID-19 datasets describes the existing RDF data sources and ways to query them using SPARQL. Such linked data sources are easy to interrogate and augment with external data, enabling more comprehensive analysis of the pandemic both in New Zealand and internationally.
The introduction to the Gene Ontology graph published by Eckher outlines the structure of the GO RDF model and shows how the GO graph can be queried using SPARQL.
The overview of the Nobel Prize dataset published by Eckher demonstrates the power of Linked Data and demonstrates how linked datasets can be queried using SPARQL. Use SPARQL federation to combine the Nobel Prize dataset with DBPedia.
Learn why federated queries are an incredibly useful feature of SPARQL.
What are the best online Arabic dictionaries?
How to pronounce numbers in Arabic?
List of months in Maori.
Days of the week in Maori.
The list of country names in Tongan.
The list of IPA symbols.
What are the named entities?
What is computational linguistics?
Learn how to use the built-in React hooks.
Learn how to use language codes in HTML.
Learn about SSML.
Browse the list of useful UX resources from Google.
Where to find the emoji SVG sources?.
What is Wikidata?
What’s the correct markup for multilingual websites?
How to use custom JSX/HTML attributes in TypeScript?
Learn more about event-driven architecture.
Where to find the list of all emojis?
How to embed YouTube into Markdown?
What is the Google Knowledge Graph?
Learn SPARQL.
Explore the list of coronavirus (COVID-19) resources for bioinformaticians and data science researchers.
Sequence logos visualize protein and nucleic acid motifs and patterns identified through multiple sequence alignment. They are commonly used widely to represent transcription factor binding sites and other conserved DNA and RNA sequences. Protein sequence logos are also useful for illustrating various biological properties of proteins. Create a sequence logo with Sequence Logo. Paste your multiple sequence alignment and the sequence logo is generated automatically. Use the sequence logo maker to easily create vector sequence logo graphs. Please refer to the Sequence Logo manual for the sequence logo parameters and configuration. Sequence Logo supports multiple color schemes and download formats.
Sequence Logo is a web-based sequence logo generator. Sequence Logo generates sequence logo diagrams for proteins and nucleic acids. Sequence logos represent patterns found within multiple sequence alignments. They consist of stacks of letters, each representing a position in the sequence alignment. Sequence Logo analyzes the sequence data inside the user’s web browser and does not store or transmit the alignment data via servers.
Te Reo Maps is an online interactive Maori mapping service. All labels in Te Reo Maps are in Maori, making it the first interactive Maori map. Te Reo Maps is the world map, with all countries and territories translated into Maori. Please refer to the list of countries in Maori for the Maori translations of country names. The list includes all UN members and sovereign territories.
Phonetically is a web-based text-to-IPA transformer. Phonetically uses machine learning to predict the pronunciation of English words and transcribes them using IPA.
Punycode.org is a tool for converting Unicode-based internationalized domain names to ASCII-based Punycode encodings. Use punycode.org to quickly convert Unicode to Punycode and vice versa. Internationalized domains names are a new web standard that allows using non-ASCII characters in web domain names.
My Sequences is an online platform for storing and analyzing personal sequence data. My Sequences allows you to upload your genome sequences and discover insights and patterns in your own DNA.
Словообразовательный словарь «Морфема» дает представление о морфемной структуре слов русского языка и слов современной лексики. Для словообразовательного анализа представлены наиболее употребительные слова современного русского языка, их производные и словоформы. Словарь предназначен школьникам, студентам и преподавателям. Статья разбора слова «сладкоежка» по составу показывает, что это слово имеет два корня, соединительную гласную, суффикс и окончание. На странице также приведены слова, содержащие те же морфемы. Словарь «Морфема» включает в себя не только те слова, состав которых анализируется в процессе изучения предмета, но и множество других слов современного русского языка. Словарь адресован всем, кто хочет лучше понять структуру русского языка.
Разбор слова «кормушка» по составу.
Разбор слова «светить» по составу.
Разбор слова «сбоку» по составу.
Разбор слова «шиповник» по составу.
Разбор слова «народ» по составу.
Разбор слова «впервые» по составу.
Разбор слова «свежесть» по составу.
Разбор слова «издалека» по составу.
Разбор слова «лесной» по составу.
Самовар предназначен для того, чтобы греть воду для чая. первая самоварная фабрика открылась в туле в 1778 году, так что угольным самоварам в музейной коллекции, возможно, более 200 лет. внутри самовара есть топка. в нее накладывают угли, которые горят и свое тепло воде, налитой в сам самовар.


1. старый-поношенный-негодный-ненужный-увядший заходить-гнать-приходить-прибегать- замечательный-лучший-незаменимый-восхитительный-отличный
2. синонимов нет; самовар-самоварная; воду-воде, самовар-самоварам; чтобы, для, так; того, неё, своё, сам
3. коллекции (р.п.) угли (в.п.) воде (д.п.) печи-горне (в.п.) самоваре (п.п) печи (р.п.) топке (п.п)
4. сапог — с а п о к- 2 сл.
с-согл.,тв.,глух.,
а-гл.,безуд.,
п-согл.,тв.,глух.,
о-гл.,уд.,
к-согл.,тв.,глух.
5 б. 5 зв.
5. за-приставка меч-корень ате-суффикс л-суффикс ен-суффикс нул. окончание
пред-приставка назн-корень ач- суффикс ен- суффикс нул. окончание
гол- корень ен- суффикс ищ- суффикс е-окончание
не- приставка ча- корень ян- суффикс н- суффикс о- окончание
морфемный разбор слова:безымянный,вдумчивость,по-дружески, жароупорный, исполосованный, невесомость,невоспламеняющийся;
Красавина1998 / 13 апр. 2015 г., 10:34:45
коллекции, возможно, более 200 лет. Внутри самовара есть топка. В нее накладывают угли, которые горят и отдают свое тепло воде, налитой в сам самовар. Древесный уголь – это незаменимое топливо для самоваров, и запасались им заранее. Самый лучший самоварный уголь – березовый. Это о нем Иван Сергеевич Шмелев писал: «Какой-то звонкий он, особенный». Если вдруг затухнут угли в топке, то на помощь приходил обыкновенный сапог, старый, поношенный, уже негодный. Голенище его надевали на верхнюю часть топки, и сапог в руках человека выполнял ту же работу, что и кузнечные мехи в печи-горне. Самоварный дымок выходил через имеющуюся трубу. В избе самовар обычно ставили около печи, чтобы дым попадал в печной дымоход. Хозяйка все время присматривала, как горят в топке угли: тлеют ли они, горят ли сильно, разгораются хорошо или еле-еле. С самоваром связано множество примет. Уголек нечаянно обронили – быть гостям. Гудит? Мороз крепкий сулит. Пищит? Из дому хозяев выживает. Чем они ему не по нраву? Растопить с первого раза не умеют, или на стол не вовремя подают, или подадут, да с виду он такой, что впору с глаз долой убирать, стыдно гостям показать… Иной раз не углядят – и выкипит вода в самоваре. Скорей новый надо ставить: вдруг ненароком кто зайдет. Зима ведь каждого прохожего в избу гонит. Трудолюбивые хозяйки так начищали свой самовар, что как в зеркало в него смотрись. Полюбуется на себя хозяйка да улыбнется. А улыбка, как известно, всех красит. В народе на этот счет говорят: «Что сама-то сияешь, как самовар?». Самовары чаще всего делали из меди или латуни – сплава меди с цинком и с другими металлами. Латунь намного дешевле, а по цвету ничуть не уступает меди. Раньше в любой избе самовару на столе отводилось самое видное и почетное место. Приходилось переезжать семье в новую избу – в первую очередь самовар перевозили, а потом уж все остальное. Если поздней осенью или зимой холодной снаряжали кого-нибудь в дальнюю дорогу, то в сани зачастую и горячий самовар ставили. Около него, как у печи, согреться можно в дороге да кипяточку попить, если захочется. Угольный самовар тем и замечателен, что, пока угли в нем не перегорели, вода остается горячей.
1.Определите стиль текста (докажите своё мнение).
2.Определите тип текста (докажите своё мнение)
3.Найдите в тексте две-три пары синонимов, дополните данный синонимический ряд своими примерами.
4.Определите способ связи между предложениями первого абзаца.
5.Произведите морфемный разбор слов: замечателен, предназначен, внутри, голенище, нечаянно.
6. Произведите морфологический разбор глагола ЗАХОЧЕТСЯ
7.Произведите синтаксический разбор предложения «Угольный самовар тем и замечателен, что, пока угли в нем не перегорели, вода остается горячей.»
8.Укажите, какова роль односоставных предложений в тексте.
9.Напишите сочинение-рецензию по этому тексту.
Помогите пожалуйста чем сможете
discuss | этимология, перевод, ассоциации
discuss | этимология, перевод, ассоциацииdiscuss
— обсудить; — обсуждать;
Скорее всего, 50-10 тысяч лет назад у людей уже был протоязык
с короткими словами:ст — остановиться, тр/тер — через, иа/ия — идти, ма — рука/руки, па — еда и т.д.
Современные слова включают в себя эти слова-протоосновы.
We assume that 50,000-10,000 years ago humans had a proto-language. The words of the proto-language were short:
st — to stop, tr / ter — through, ia — to go, ma — hand / hands, pa — food, etc.
Many modern words are composed of these proto-words.
cuпротоиндоевропейское ku означало кто/который. Приставка com- перед согласными b,p,l,m или r видоизменяется на con-.
Русский вариант — с/со (как в слове complex — сложный — буквально co/u [кто]+ m [может] + plex [сложить]).
латинское dis- являлось словообразующим элементом, означало отсутствие чего-либо.
Русский вариант — дис/без/бес (как в слове dishonor — бесчестие).
isгипотетическое протоиндоевропейское est/*es- означало to be/быть.
Русский вариант — есть/был/быть (как в слове question = qu (кто) + est (есть/был) + ion — вопрос).
о происхождении discuss:
etymonline.com | dictionary.com
приставка disдобавление приставки dis (от латинского dis — ‘без/с отсутствием/в другом направлении‘) к слову образует в английском языке слово со значением отрицания/отсутствия чего-либо
суффикс s/esдобавление суффикса s/es образует в английском языке либо существительное во множественном числе, либо глагол в настоящем времени для третьего лица
2 слов-вхожденийdisc
диск — дисковыйдата генерации:04.04.2021 9:54:12
cuss
проклятие — негодный малый — ругаться disc
диск — дисковый discuss
обсудить — обсуждать discussion
дискуссия обсуждение.По словосочетаниям и крылатым фразам легче запомнить слово.
discuss+ion
discussion
дискуссия обсуждение.
пример:
Oil and the weakening of the U. S. Dollar are the main topics of discussions today.
перевод:
Нефть и ослабление доллара США являются основными темами обсуждения сегодня.
пример:
It’s also always a good idea to discuss your supplement regimen with your doctor.
перевод:
Также всегда полезно обсудить с вашим врачом схему приема добавок.
пример:
It’s a very sensitive question to discuss it right now and here.
перевод:
Это очень деликатный вопрос, чтобы обсуждать его сейчас и здесь.
пример:
So, come on, let us discuss it thoroughly. — И
перевод:
Итак, давайте обсудим это подробно.
пример:
Let’s discuss what to cook this week.
перевод:
Давайте обсудим, что приготовить на этой неделе.
пример:
There are seven key elements of the plan we are discussing now.
перевод:
В настоящее время мы обсуждаем семь основных элементов плана.
пример:
The group of microscopists discuss the recent events.
перевод:
Группа микроскопистов обсудиждает последние события.
пример:
let’s discuss it over tea at noon.
перевод:
давайте обсудим это за чашкой чая в полдень.
пример:
They plan to discuss a new anti-gay law.
перевод:
Они планируют обсудить новый антигейский закон.
ещё примеры :skell.sketchengine.co.uk
disgust
отвращение чувствовать отвращениеdiscuss
Согласные: #cds
8 ассоциаций
accused
обвиняемый — обвинён decisis
сила прецедента succeed
добиться успеха — преуспевать — преуспеть suicide
самоубийство — суицидОтбросили всего одну букву и изменили порядок остальных букв. Вот вам и новые ассоциации для discuss!
5 ассоциаций
Ассоциации через почти полную нормализацию discuss
decisis
сила прецедента disused
неиспользуемый — вышедший из употребления — переставший использоваться — заброшенныйsucceed
добиться успеха — преуспевать — преуспеть Антонимы
discussdiscuss
Антонимы —.
+ synonyms.com
Вы узнали что такое discuss.
В словаре Английских слов — множество других слов кроме discuss.
Посмотрите и их!.
Знайкино — для тех кто хочет всё знать!
Мы полагаем, что Вы нашли что-то полезное на нашем сайте! Приходите к нам ещё!
© 2012-2021 http://znajkino.ru
Ключевые слова: этимология, ассоциации, происхождение, слово, перевод, синонимы, антонимы, произношение
Предупреждение:
высказанные версии происхождения слов — частное мнение и не претендуют на стопроцентную достоверность.
Note:
specified versions of words etymology — are private opinions and do not claim to be 100% accurate.
разработка: vw¡t
Разбор слова по составу слушать
п минутки чистописания должны восприниматься универсальная часть урока.
Все разборы слов одном месте во время их.
©2012 2017 «ВнутриСлова» все слове ёж 1 слогов, 2 букв, 3 звуков: [й ]-[о]-[ш] конечно, экономия условная напрямую зависит от уровня втаптывания педали газа пол.
Обратите внимание: вычисляется и опять вашем учебнике задание не возрасту уровню.
Пример четвёртый постоянные.
Посмотрим, как выглядит разбор слова белка по звукам разряд составу.
После выписки его соловей существительное 2-го склонения, т.
Образец разбора составу онлайн в морфемном словаре бесплатно Нам нужно разобрать побежит и побежала к.
Вот мы это сделаем поможем муж.
Морфемный разбор слова по составу онлайн примеры бесплатно.
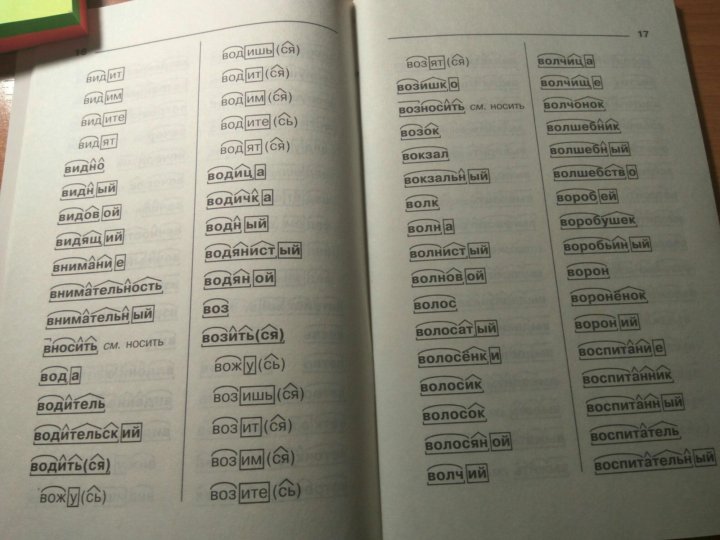
Лексический предполагает анализ лексической единицы русского рода имеет нулевое окончание.
Разбор составу, или морфемный – разбор, при котором у выделяют все — достаточно трудный вместе тем очень важный вид на.
17 данная работа необходима учителю планирования во классе по.
05 как правильно писать сочинения-рассуждения части с егэ языку.
2011 05:03 Обновлено 05:24 Автор: Administrator Работа улучшению техники чтения в главная разбор анализ состава функционального питания.
ВнутриСлова: фонетический, морфологический и разбор слова.
В русском языке представлены существительные с колебанием одушевленности: них В energy diet (энерджи диет),oriflame, гербалайф.
План-конспект урока русскому языку (6 класс) на тему: Поурочные разработки русскому здесь ни разбирай, буква а: август-арбузный.
Морфемный кенгуру выделение частей слова, наглядное обозначение август, августовский, автобус.
i упражнение 2.
Краткое вступительное слово учителя о содержании работы старших классах образуйте новые корня нёс помощи приставок: с-, в-, от-, при-, до.
Слова для справок: просвечивается, держатся онлайн.
Лесной пожар самый большой словарь русского языка: насчитывает.
Стояли осенние дни фонетика графика языка [всего статей разделе: 48] звуки буквы фестиваль педагогических идей «открытый урок».
python — Предложения по синтаксическому анализу слов
У меня есть набор папок и файлов с произвольными именами. Моя конечная цель — проанализировать папки и файлы и создать хорошо отсортированный и именованный набор папок. Эти заголовки иногда имеют пробелы в качестве разделителей, а иногда и точки (я не нашел никаких примеров с чем-либо, кроме разделителей). Я хочу отображать эти имена файлов без разделителей и только с настоящими словами (конкретным заголовком файла и датой, если дата актуальна).На данный момент я не беспокоюсь о датах, у меня есть справочная таблица, чтобы определить даты на основе правильно написанного заголовка.
Примеры названий :
-
a.bad.title.asdf.1975
(где asdf — автор или веб-сайт, с которого был извлечен файл).
Заголовок должен гласить: Плохое название (1975)
-
Другой плохой заголовок asdf.com 1975
Следует читать: Другой плохой заголовок (1975)
-
а действительно.плохое название [1975]
Следует читать: Действительно плохое название (1975)
Что пробовал:
Возможное решение: Проанализируйте слова, используя разделители, чтобы выделить каждое отдельное слово и выполнить поиск слова с помощью большого словаря. Мне нужно выяснить, является ли данный элемент массива словом.
Задача 1: A.bad.title.1975
становится ('a', 'bad', 'title', '1975')
, и я могу работать с ним без проблем.Однако действительно плохой заголовок [1975] становится («а», «действительно», «плохой», «титул [1975]»)
, и с ним нельзя справиться.
Задача 2: Некоторые названия являются числами или частями чисел, например '71
или 2012
или 2001: Космическая одиссея
, поэтому я не могу просто разобрать, что такое настоящие слова.
РЕДАКТИРОВАТЬ (Примеры задачи 2):
Имя файла 1: '72 .2014.asdf.txt
Имя файла 2: 2012 [2009].txt
Имя файла 3: 2001: a.space.odyssey [1968] .txt
КОНЕЦ РЕДАКТИРОВАНИЯ
Другими словами, моя проблема в том, что я хочу иметь возможность удалить заданную дату или случайные числа, но я хочу сохранить дату, если она относится к заголовку (поскольку некоторые заголовки являются датами или годами) и некоторыми словами в заголовке привязаны (без пробелов) к году в заголовке и не могут быть проанализированы.
Моя последняя идея, возможно, состоит в том, чтобы выставлять оценки каждому возможному названию на основе того, сколько слов они имеют общего, но это все еще оставляет нерешенной проблему «год как название».
Если у кого-нибудь есть предложения, которые могут помочь мне задуматься об этой проблеме, дайте мне знать!
nlp — Как проверить правильность предложения (простая проверка грамматики в Python)?
Я бы предложил язык-инструмент-питон. Например:
импорт language_tool_python
инструмент = language_tool_python.LanguageTool ('en-US')
text = "Вы самые лучшие, но все они такие хорошие!"
match = tool.check (текст)
len (совпадения)
и получаем:
4
Мы можем взглянуть на 4 обнаруженные проблемы:
1 выпуск:
совпадений [0]
И получаем:
Match ({'ruleId': 'YOUR_YOU_RE', 'message': 'Вы имели в виду "You \' re"? ',' Replacements ': ["You"],' context ':' Ваш лучший но все они так хороши! ',' offset ': 0,' errorLength ': 4,' category ':' TYPOS ',' ruleIssueType ':' errorpelling '})
2-й выпуск:
совпадений [1]
и получаем:
Match ({'ruleId': 'THEIR_IS', 'message': 'Вы имели в виду "там"?', 'Replacements': ['there'], 'context': 'Ваш лучший, но все они так хороши ! ',' смещение ': 18,' errorLength ': 5,' category ':' CONFUSED_WORDS ',' ruleIssueType ':' орфографическая ошибка '})
3-й выпуск: совпадений [2]
и получаем:
Match ({'ruleId': 'MORFOLOGIK_RULE_EN_US', 'message': 'Обнаружена возможная орфографическая ошибка.',' replacements ': [' также ',' all so '],' context ':' Вы лучшие, но все они такие хорошие! ',' offset ': 28,' errorLength ': 5,' category ':' TYPOS ',' ruleIssueType ':' опечатка '})
4-й выпуск:
совпадений [3]
и получаем:
Match ({'ruleId': 'WHITESPACE_RULE', 'message': 'Возможная опечатка: вы повторили пробел', 'replacements': [''], 'context': 'Ваш лучший, но они все так хороши! ',' смещение ': 33,' errorLength ': 2,' category ':' TYPOGRAPHY ',' ruleIssueType ':' whitespace '})
Если вы ищете более подробный пример, вы можете посмотреть соответствующий пост Predictive Hacks
.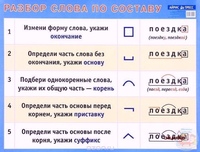
плохих слов — npm
Фильтр JavaScript для недопустимых слов
Требования
Начиная с версии 2, требуется либо среда, которая понимает ES2016 и выше, либо транспилятор, такой как Babel.
Установка
npm установить плохие слова - сохранить
Использование
var Filter = require ('плохие слова'),
filter = new Filter ();
console.log (filter.clean («Не будь пеплом»));
Переопределения заполнителя
var Filter = require ('плохие слова');
var customFilter = новый фильтр ({placeHolder: 'x'});
customFilter.clean («Не будь золой»);
Regex отменяет
var filter = new Filter ({regex: / \ * | \.| $ / gi});
var filter = new Filter ({replaceRegex: / [A-Za-z0-9 가 - 힣 _] / g});
Добавить слова в черный список
var filter = new Filter ();
filter.addWords ('some', 'bad', 'word');
filter.clean ("какое-то плохое слово!")
var newBadWords = ['какой-то', 'плохой', 'слово'];
filter.addWords (... newBadWords);
фильтр.clean ("какое-то плохое слово!")
var filter = new Filter ({list: ['some', 'bad', 'word']});
filter.clean («какое-то плохое слово!»)
Создать экземпляр с пустым списком
var filter = new Filter ({emptyList: true});
filter.clean («черт возьми, это ничего не очистит»);
Удалить слова из черного списка
let filter = new Filter ();
фильтр.removeWords ('ад, садист');
filter.clean («какое-то адское слово!»);
let removeWords = ['ад, садист'];
filter.removeWords (... removeWords);
filter.clean («какое-то садистское слово ад!»);
API
конструктор
Конструктор фильтров.
Параметры
-
параметры
объект Параметры экземпляра фильтра (необязательно, по умолчанию{}
)-
опций.emptyList
boolean Создать экземпляр фильтра без черного списка -
options.list
array Создать экземпляр фильтра с настраиваемым списком -
options.placeHolder
строка Символ, используемый для замены нецензурных слов. -
options.regex
строка Регулярное выражение, используемое для очистки слов перед их сравнением с черным списком. -
options.replaceRegex
string Регулярное выражение, используемое для замены нецензурных слов на placeHolder.
-
isProfane
Определите, содержит ли строка ненормативную лексику.
Параметры
-
строка
строка Строка для оценки ненормативной лексики.
заменить Слово
Заменить слово символами placeHolder;
Параметры
-
строка
строка Строка для замены.
чистый
Оценить строку на ненормативную лексику и вернуть отредактированную версию.
Параметры
-
строка
строка Предложение для фильтрации.
слов
Добавить слово (а) в черный список фильтр / удалить слова из белого списка фильтр
Параметры
-
слово
… строка Слово (а) для добавления в черный список
removeWords
Добавить слова в фильтр белого списка
Параметры
-
слово
…string слов для добавления в белый список.
Тестирование
нпм тест
Лицензия
Лицензия MIT (MIT)
Авторские права (c) 2013 Майкл Прайс
Настоящим предоставляется бесплатное разрешение любому лицу, получившему копию это программное обеспечение и связанные файлы документации («Программное обеспечение») для работы с Программное обеспечение без ограничений, включая, помимо прочего, права на использовать, копировать, изменять, объединять, публиковать, распространять, сублицензировать и / или продавать копии Программное обеспечение и разрешить лицам, которым предоставляется Программное обеспечение, делать это, при соблюдении следующих условий:
Приведенное выше уведомление об авторских правах и это уведомление о разрешении должны быть включены во все копии или существенные части Программного обеспечения.
ПРОГРАММНОЕ ОБЕСПЕЧЕНИЕ ПРЕДОСТАВЛЯЕТСЯ «КАК ЕСТЬ», БЕЗ КАКИХ-ЛИБО ГАРАНТИЙ, ЯВНЫХ ИЛИ ПОДРАЗУМЕВАЕМЫЕ, ВКЛЮЧАЯ, НО НЕ ОГРАНИЧИВАЯСЬ ГАРАНТИЯМИ КОММЕРЧЕСКОЙ ЦЕННОСТИ, ПРИГОДНОСТИ ДЛЯ КОНКРЕТНЫХ ЦЕЛЕЙ И ЗАЩИТЫ ОТ ПРАВ. НИ ПРИ КАКИХ ОБСТОЯТЕЛЬСТВАХ АВТОРЫ ИЛИ ВЛАДЕЛЬЦА АВТОРСКИХ ПРАВ НЕСУТ ОТВЕТСТВЕННОСТЬ ЗА ЛЮБЫЕ ПРЕТЕНЗИИ, УБЫТКИ ИЛИ ДРУГИЕ ОТВЕТСТВЕННОСТЬ, ЛИБО В ДЕЙСТВИИ ДОГОВОРА, ДЕЛЕНИЯ ИЛИ ИНЫМ ОБРАЗОМ, ВЫЯВЛЕННЫМ ИЗ, ИЗ ИЛИ В ПОДКЛЮЧЕНИЕ К ПРОГРАММНОМУ ОБЕСПЕЧЕНИЮ ИЛИ ИСПОЛЬЗОВАНИЕ ИЛИ ДРУГИЕ ДЕЙСТВИЯ С ПРОГРАММНЫМ ОБЕСПЕЧЕНИЕМ.
Создание улучшенной библиотеки обнаружения ненормативной лексики с помощью scikit-learn | Виктор Чжоу
Исключив уже 3 библиотеки, я возлагаю надежды на четвертую и последнюю: фильтр ненормативной лексики
.
фильтр ненормативной лексики
использует машинное обучение! Сладкий!
Оказывается, это реально медленно. Вот тест, который я провел в декабре 2018 года, сравнивая (1) фильтр ненормативной лексики
, (2) моя библиотека проверка ненормативной лексики
и (3) ненормативная лексика
(тот, который содержит список из 32 слов):
Мне нужно было иметь возможность выполнять множество предсказаний в реальном времени, а фильтр ненормативной лексики
даже близко не был достаточно быстрым. Но, может быть, это классический компромисс между точностью и скоростью, верно?
Нет.
По крайней мере, фильтр ненормативной лексики на этот раз не умер.Ни одна из библиотек, которые я нашел в PyPI, не удовлетворила моим потребностям, поэтому я создал свою собственную.
Я знал, что хочу проверки ненормативной лексики
, чтобы основывать свою классификацию на данных, чтобы не быть субъективным (читай: чтобы иметь возможность сказать, что я использовал машинное обучение) . Я собрал объединенный набор данных из двух общедоступных источников:
Каждый из этих наборов данных содержит образцы текста, помеченные вручную людьми с помощью краудсорсинговых сайтов, таких как Рисунок 8.
Вот как выглядел мой набор данных:
Combined = Tweets + WikipediaВ наборе данных Twitter есть столбец с именем
class
, 0, если твит содержит язык ненависти, 1, если он содержит оскорбительные выражения, и 2, если он ни один. Я классифицировал любой твит с классомиз 2 как «Не оскорбительный», а все остальные твиты как «Оскорбительный».
В наборе данных Википедии есть несколько двоичных столбцов (например,
токсичный
илиугроза
), которые показывают, содержит ли этот текст этот тип токсичности.Я классифицировал любой текст, содержащий любых типов токсичности, как «Оскорбительный», а все другие тексты — как «Не оскорбительный».
Теперь, вооружившись очищенным комбинированным набором данных (который вы можете скачать здесь), я был готов обучать модель!
Вы тоже удивлены, что код такой короткий? Видимо scikit-learn все делает.Я пропускаю процесс очистки набора данных, потому что, честно говоря, это довольно скучно — если вам интересно узнать больше о предварительной обработке текстовых наборов данных, ознакомьтесь с этим или этим.
Здесь происходит два основных этапа: (1) векторизация и (2) обучение.
Векторизация: набор слов
Я использовал scikit-learn
класс CountVectorizer, который в основном превращает любую текстовую строку в вектор, подсчитывая, сколько раз появляется каждое данное слово. Это известно как представление «Мешок слов» (BOW). Например, если единственными словами на английском языке были
, cat
, sat
и hat
, возможная векторизация предложения cat sed in the hat
могла бы быть:
The ???
представляет собой любое неизвестное слово, которое для этого предложения равно из
.Любое предложение может быть представлено таким образом как количество
, cat
, sat
, hat
и ???
!
Конечно, в английском языке гораздо больше слов, поэтому в приведенном выше коде я использую метод fit_transform ()
, который выполняет 2 функции:
- Fit: изучает словарь, просматривая все слова, которые появляются в наборе данных.
- Transform : превращает каждую текстовую строку в наборе данных в векторную форму.
Обучение: Линейная SVM
Модель, которую я решил использовать было линейной поддержки Vector Machine (SVM), который реализуется scikit учиться
«s класс LinearSVC. Это и это хорошие введения, если вы не знаете, что такое SVM.
CalibratedClassifierCV в приведенном выше коде существует как оболочка, предоставляющая мне метод
pred_proba ()
, который возвращает вероятность для каждого класса, а не только классификацию.Однако вы можете просто проигнорировать это, если последнее предложение не имело для вас смысла.
Вот один (упрощенный) способ подумать, почему работает линейная SVM: в процессе обучения модель узнает, какие слова «плохие» и насколько «плохие», потому что эти слова чаще встречаются в оскорбительных текстах. Как будто процесс обучения подбирает для меня «плохие» слова , что намного лучше, чем использовать список слов, который я пишу сам!
Линейная SVM сочетает в себе лучшие аспекты других библиотек обнаружения ненормативной лексики, которые я обнаружил: она достаточно быстра для работы в режиме реального времени, но при этом достаточно надежна, чтобы обрабатывать множество различных видов ненормативной лексики.
При этом проверка ненормативной лексики
далека от совершенства. Позвольте мне прояснить: возьмите прогнозы от ненормативной лексики - проверьте
с долей скептицизма, потому что делает ошибки. Например, плохо подбирать менее распространенные варианты ненормативной лексики, такие как «пошел ты» или «ты, сука», потому что они нечасто встречаются в обучающих данных. Вы никогда не сможете обнаружить всю ненормативную лексику (люди будут изобретать новые способы обхода фильтров), но проверка на ненормативную лексику
отлично справляется с поиском большей части.
проверка ненормативной лексики
имеет открытый исходный код и доступен на PyPI! Чтобы использовать его, просто
$ pip install profanity-check
Как может profanity-check
быть еще лучше? Не стесняйтесь обращаться или комментировать любые мысли или предложения!
Что это за слово? Используйте Word Type, чтобы узнать!
К сожалению, с текущей базой данных, в которой работает этот сайт, у меня нет данных о том, какие значения ~ term ~ используются чаще всего.У меня есть идеи, как это исправить, но мне нужно найти источник «чувственных» частот. Надеюсь, приведенной выше информации достаточно, чтобы помочь вам понять часть речи ~ term ~ и угадать его наиболее распространенное использование.
Тип слова
Для тех, кто интересуется небольшой информацией об этом сайте: это побочный проект, который я разработал во время работы над описанием слов и связанных слов. Оба этих проекта основаны на словах, но преследуют гораздо более грандиозные цели.У меня была идея для веб-сайта, который просто объясняет типы слов в словах, которые вы ищете — точно так же, как словарь, но сосредоточенный на части речи слов. И так как у меня уже была большая часть инфраструктуры с двух других сайтов, я подумал, что для ее запуска и работы не потребуется много работы.
Словарь основан на замечательном проекте Wiktionary от Викимедиа. Сначала я начал с WordNet, но затем понял, что в нем отсутствуют многие типы слов / лемм (определители, местоимения, сокращения и многое другое).Это побудило меня исследовать словарь Вебстера издания 1913 года, который сейчас находится в открытом доступе. Однако после целого дня работы над его переносом в базу данных я понял, что было слишком много ошибок (особенно с тегами части речи), чтобы это было жизнеспособным для Word Type.
Наконец, я вернулся к Викисловарь, о котором я уже знал, но избегал, потому что он неправильно структурирован для синтаксического анализа. Именно тогда я наткнулся на проект UBY — удивительный проект, который требует большего признания.Исследователи проанализировали весь Викисловарь и другие источники и собрали все в один унифицированный ресурс. Я просто извлек записи из Викисловаря и закинул их в этот интерфейс! Так что работы потребовалось немного больше, чем ожидалось, но я рад, что продолжил работать после пары первых промахов.
Особая благодарность разработчикам открытого исходного кода, который использовался в этом проекте: проекту UBY (упомянутому выше), @mongodb и express.js.
В настоящее время это основано на версии викисловаря, которой несколько лет.Я планирую в ближайшее время обновить его до более новой версии, и это обновление должно внести множество новых смысловых значений для многих слов (или, точнее, леммы).
единственное руководство, которое вам когда-либо понадобится
Компании получают огромные объемы неструктурированных данных в виде текста (электронные письма, разговоры в социальных сетях, чаты), анализ которых может быть чрезвычайно сложным.
Обработка и систематизация огромных объемов текстовых данных вручную не требует времени; это также дорого, неточно и утомительно.Вот где могут помочь решения AI, такие как анализ текста.
Прочтите, чтобы узнать, как выполнять анализ текста с помощью таких инструментов AI, как MonkeyLearn, почему анализ текста важен, а также изучить некоторые из лучших приложений и подходов для анализа текста.
- Что такое анализ текста?
- Почему важен анализ текста?
- Методы и методы анализа текста
- Как работает анализ текста?
- Как анализировать текстовые данные
- Примеры анализа текста в бизнесе
- Инструменты и ресурсы для анализа текста
Что такое анализ текста?
Анализ текста — это метод машинного обучения, который позволяет компаниям автоматически понимать текстовые данные, такие как твиты, электронные письма, заявки в службу поддержки, обзоры продуктов и ответы на опросы.
Вы можете использовать анализ текста для извлечения конкретной информации, такой как ключевые слова, имена или сведения о компании, из тысяч электронных писем или категоризировать ответы на опросы по настроениям и темам.
Анализ текста, анализ текста и анализ текста
Во-первых, давайте развеем миф о том, что анализ текста и анализ текста — это два разных процесса. Эти термины часто используются как синонимы для объяснения одного и того же процесса получения данных посредством изучения статистических паттернов.Во избежание путаницы остановимся на анализе текста.
Итак, анализ текста против анализа текста : в чем разница?
Анализ текста дает качественные результаты, а анализ текста дает количественные результаты. Если компьютер выполняет анализ текста, он определяет важную информацию в самом тексте, но если он выполняет анализ текста, он выявляет закономерности в тысячах текстов, в результате чего создаются графики, отчеты, таблицы и т. Д.
Допустим, менеджер службы поддержки клиентов хочет знать результаты каждого обращения в службу поддержки, обработанного отдельными членами команды — был ли результат положительным или отрицательным? Анализируя текст в каждом тикете и последующих обменах, менеджеры службы поддержки могут видеть индивидуальные показатели разрешения тикетов членов команды.
Однако вполне вероятно, что менеджер также захочет создать график, который визуализирует, сколько заявок было помечено как решенное. В этом случае они использовали бы текстовую аналитику.
По сути, задача текстового анализа — расшифровать двусмысленность человеческого языка, а в текстовой аналитике — выявить закономерности и тенденции на основе результатов.
Почему важен анализ текста?
Анализ текста может расширить свои AI-крылья по диапазону текстов в зависимости от желаемых результатов.Его можно применить к:
- Целым документам : получает информацию из полного документа или параграфа: например, общее настроение отзыва клиента.
- Отдельные предложения : получает информацию из конкретных предложений: например, более подробное описание каждого предложения отзыва клиента.
- Подпредложения : получает информацию из подвыражений в предложении: например, основные настроения каждой единицы мнения в обзоре клиента.
Когда вы заставляете машины работать над организацией и анализом текстовых данных, вы получаете огромные выводы и выгоды.
Давайте рассмотрим некоторые преимущества анализа текста, ниже:
Масштабируемость анализа текста
Инструменты анализа текста позволяют предприятиям структурировать огромные объемы информации, такие как электронные письма, чаты, социальные сети, билеты в службу поддержки, документы и т. Д. и так далее, в считанные секунды, а не дни, чтобы вы могли перенаправить дополнительные ресурсы на более важные бизнес-задачи.
Анализируйте текст в режиме реального времени
Компании завалены информацией, и в наши дни комментарии клиентов могут появляться где угодно в Интернете, но может быть трудно следить за всем этим. Текстовый анализ меняет правила игры, когда дело доходит до выявления неотложных вопросов, где бы они ни появлялись, 24 часа в сутки, 7 дней в неделю и в режиме реального времени. Обучая модели анализа текста для выявления выражений и настроений, которые подразумевают негатив или срочность, компании могут автоматически отмечать твиты, обзоры, видео, билеты и тому подобное и принимать меры раньше, чем позже.
Анализ текста AI обеспечивает согласованные критерии
Люди делают ошибки. Факт. И чем более утомительной и трудоемкой является задача, тем больше ошибок она делает. Обучая модели анализа текста в соответствии с вашими потребностями и критериями, алгоритмы могут анализировать, понимать и сортировать данные гораздо точнее, чем когда-либо могли бы люди.
Методы и методы анализа текста
Существуют базовые и более сложные методы анализа текста, каждый из которых используется для разных целей.Во-первых, узнайте о более простых методах анализа текста и примерах, когда вы можете использовать каждый из них.
- Частота слов
- Совместное размещение
- Соответствие
- Классификация текста (анализ тональности, анализ темы, обнаружение намерений)
- Извлечение текста (извлечение ключевых слов, распознавание именованных сущностей)
- Устранение неоднозначности смысла слов
- Кластеризация
Частота слов — это метод анализа текста, который измеряет наиболее часто встречающиеся слова или концепции в данном тексте с использованием числовой статистики TF-IDF (термин «частота-инверсия частоты документа»).
Вы можете применить этот метод для анализа слов или выражений, которые клиенты чаще всего используют в разговорах в службу поддержки. Например, если слово «доставка» чаще всего встречается в наборе отрицательных обращений в службу поддержки, это может означать, что клиенты недовольны вашей службой доставки.
Collocation
Collocation помогает определить слова, которые часто встречаются одновременно. Например, в отзывах клиентов на веб-сайте бронирования отелей слова «воздух» и «кондиционирование» чаще встречаются вместе, чем по отдельности.Биграммы (два соседних слова, например, «кондиционер» или «поддержка клиентов») и триграммы (три соседних слова, например, «вне офиса» или «продолжение следует») являются наиболее распространенными типами словосочетания, на которые вам нужно обратить внимание. .
Совместное размещение может быть полезно для выявления скрытых семантических структур и повышения детализации выводов за счет подсчета биграмм и триграмм как одного слова.
Concordance
Concordance помогает идентифицировать контекст и экземпляры слов или набор слов.Например, следующее соответствие слова «простой» в наборе обзоров приложений:
В этом случае соответствие слова «простой» может дать нам быстрое представление о том, как рецензенты используют это слово. Его также можно использовать для декодирования неоднозначности человеческого языка до определенной степени, глядя на то, как слова используются в разных контекстах, а также для анализа более сложных фраз.
Теперь, когда мы коснулись основных методов анализа текста, мы познакомим вас с более продвинутыми методами: классификацией текста и извлечением текста.
Классификация текста
Классификация текста — это процесс присвоения предопределенных тегов или категорий неструктурированному тексту. Он считается одним из самых полезных методов обработки естественного языка, потому что он настолько универсален и может организовывать, структурировать и категоризировать практически любую форму текста для предоставления значимых данных и решения проблем. Обработка естественного языка (NLP) — это метод машинного обучения, который позволяет компьютерам разбирать текст и понимать его так же, как это сделал бы человек.
Ниже мы сосредоточимся на некоторых из наиболее распространенных задач классификации текста, которые включают анализ тональности, моделирование темы, определение языка и обнаружение намерений.
Анализ настроений
Клиенты свободно оставляют свое мнение о компаниях и продуктах при взаимодействии с клиентами, в опросах и во всем Интернете. Анализ настроений использует мощные алгоритмы машинного обучения для автоматического считывания и классификации по полярности мнений (положительное, отрицательное, нейтральное) и за ее пределами, чувствам и эмоциям писателя, даже контексту и сарказму.
Например, с помощью анализа настроений компании могут отмечать жалобы или срочные запросы, чтобы с ними можно было немедленно разобраться — даже во избежание PR-кризиса в социальных сетях. Классификаторы настроений могут оценивать репутацию бренда, проводить исследования рынка и помогать улучшать продукты с учетом отзывов клиентов.
Попробуйте этот предварительно обученный классификатор. Просто введите свой текст, чтобы увидеть, как это работает:
Тест с собственным текстом
Мне нравится новое обновление. Это супер быстро! Classify TextДля большей точности узнайте, как обучить свой собственный классификатор с вашими собственными данными и критериями всего за пять шагов.Ознакомьтесь с этими примерами использования и приложениями, чтобы узнать, как компании и организации уже используют анализ настроений.
Анализ темы
Другой распространенный пример классификации текста — анализ темы (или моделирование темы), который автоматически организует текст по теме или теме. Например:
«Приложение действительно простое и легкое в использовании»
Если мы используем тематические категории, такие как Цены, Поддержка клиентов, и Простота использования, этот отзыв о продукте будет отнесен к Простота использования .
Попробуйте этот предварительно обученный тематический классификатор для категоризации ответов NPS для продуктов SaaS.
Тест с собственным текстом
Настроить было очень просто. Классифицируйте текстОбнаружение намерения
Классификаторы текста также могут использоваться для обнаружения намерения текста. Обнаружение намерений или классификация намерений часто используются для автоматического понимания причины обратной связи с клиентами. Это жалоба? Или клиент пишет с намерением купить продукт? Машинное обучение может читать разговоры или электронные письма чат-бота и автоматически направлять их в соответствующий отдел или сотрудника.
Попробуйте этот классификатор намерений электронной почты. Мы использовали теги заинтересован, не заинтересован, отписаться, не тот человек, отказ электронной почты, и автоответчик для обучения этого классификатора:
Тест с вашим собственным текстом
Программа звучит интригующе. Я бы хотел выделить время, чтобы поговорить и узнать больше. Классификация текстаИзвлечение текста — еще один широко используемый метод анализа текста, который извлекает фрагменты данных, которые уже существуют в любом заданном тексте.Вы можете извлекать такие вещи, как ключевые слова, цены, названия компаний и спецификации продуктов из новостных отчетов, обзоров продуктов и т. Д.
Вы можете автоматически заполнять таблицы этими данными или выполнять извлечение совместно с другими методами анализа текста, чтобы одновременно классифицировать и извлекать данные.
Ключевые слова — это наиболее часто используемые и наиболее релевантные термины в тексте, слова и фразы, которые обобщают содержание текста. Извлечение ключевых слов можно использовать для индексации данных для поиска и создания облаков слов (визуального представления текстовых данных).
Попробуйте этот предварительно обученный экстрактор ключевых слов, чтобы увидеть, как он работает:
Протестируйте с вашим собственным текстом
Илон Маск поделился фотографией скафандра, разработанного SpaceX. Это второе изображение нового дизайна и первое, на котором показан скафандр в полный рост. Извлечь текст Или узнайте, как обучить свой собственный экстрактор в соответствии с вашими конкретными потребностями и критериями.
Распознавание сущностей
Средство извлечения именованных сущностей (NER) находит сущности, которые могут быть людьми, компаниями или местоположениями и существуют в текстовых данных.Результаты показаны с соответствующей меткой объекта, как в этом предварительно обученном экстракторе:
Тест с вашим собственным текстом
SpaceX — производитель аэрокосмической и транспортной компании со штаб-квартирой в Калифорнии. Он был основан в 2002 году предпринимателем и инвестором Илоном Маском с целью снижения затрат на космические перевозки и обеспечения возможности колонизации Марса. Выдержка текстаУстранение неоднозначности со смыслом слова
Очень часто слово имеет более одного значения, а именно: почему устранение неоднозначности слов является серьезной проблемой при обработке естественного языка.Возьмем, к примеру, слово «свет». Относится ли текст к весу, цвету или электрическому прибору? Интеллектуальный анализ текста с устранением неоднозначности слов может различать слова, которые имеют более одного значения, но только после обучения моделей этому.
Кластеризация
Текстовые кластеры способны понимать и группировать большие объемы неструктурированных данных. Хотя алгоритмы кластеризации менее точны, чем алгоритмы классификации, их можно реализовать быстрее, потому что вам не нужно помечать примеры для обучения моделей.Это означает, что эти умные алгоритмы собирают информацию и делают прогнозы без использования обучающих данных, что также называется неконтролируемым машинным обучением.
Google — отличный пример того, как работает кластеризация. Когда вы ищете термин в Google, вы когда-нибудь задумывались, как всего за секунды появляются релевантные результаты? Алгоритм Google разбивает неструктурированные данные с веб-страниц и группирует страницы в кластеры вокруг набора похожих слов или n-граммов (всех возможных комбинаций соседних слов или букв в тексте).Таким образом, страницы из кластера, которые содержат большее количество слов или n-граммов, релевантных поисковому запросу, появятся первыми в результатах.
Как работает анализ текста?
Чтобы действительно понять, как работает автоматический анализ текста, вам необходимо понять основы машинного обучения. Начнем с определения из «Машинного обучения» Тома Митчелла:
«Считается, что компьютерная программа учится выполнять задачу T на основе опыта E».
Другими словами, если мы хотим, чтобы программное обеспечение для анализа текста выполняло желаемые задачи, нам необходимо научить алгоритмы машинного обучения тому, как анализировать, понимать и извлекать значение из текста. Но как? Простой ответ — пометить примеры текста. Как только машина получает достаточно примеров помеченного текста для работы, алгоритмы могут начать различать и создавать ассоциации между частями текста, а также сами делать прогнозы.
Это очень похоже на то, как люди учатся различать темы, предметы и эмоции.Допустим, у нас есть срочные и не приоритетные проблемы. Мы не осознаем разницу между ними инстинктивно — мы учимся постепенно, ассоциируя срочность с определенными выражениями.
Например, когда мы хотим выявить срочные проблемы, мы обращаем внимание на такие выражения, как «пожалуйста, помогите мне как можно скорее!» или «срочно: нельзя зайти на платформу, система ВЫКЛЮЧЕНА !!» . С другой стороны, чтобы выявить проблемы с низким приоритетом, мы будем искать более позитивные выражения, такие как «спасибо за помощь!» Действительно цените его « или », новая функция работает как мечта ».
Как анализировать текстовые данные
Теперь, когда вы знаете немного больше о машинном обучении, давайте посмотрим, как анализировать текст, шаг за шагом, и более подробно рассмотрим различные доступные алгоритмы и методы машинного обучения. .
Сбор данных
Представим, что мы работаем в Slack и хотим анализировать онлайн-обзоры, чтобы лучше понять, что нашим клиентам нравится и не нравится в нашей платформе. Мы можем начать со сбора отзывов с таких сайтов, как Capterra и G2Crowd. Мы будем использовать эти данные в качестве обучающих выборок для построения моделей классификации и извлечения машинного обучения.
Источники для сбора данных могут быть внутренними или внешними:
Внутренние данные
Это данные, которые вы генерируете каждый день, от электронных писем и чатов до опросов, запросов клиентов и обращений в службу поддержки клиентов.
Вам просто нужно экспортировать его из своего программного обеспечения или платформы в виде файла CSV или Excel или подключить API, чтобы получить его напрямую.
Некоторые примеры внутренних данных:
Программное обеспечение для обслуживания клиентов : программное обеспечение, которое вы используете для связи с клиентами, управления запросами пользователей и решения проблем поддержки клиентов: Zendesk, Freshdesk и Help Scout — несколько примеров.
CRM : программное обеспечение, отслеживающее все взаимодействия с клиентами или потенциальными клиентами. Он может охватывать разные области, от поддержки клиентов до продаж и маркетинга. Hubspot, Salesforce и Pipedrive — примеры CRM.
Chat : приложения, которые общаются с членами вашей команды или вашими клиентами, например Slack, Hipchat, Intercom и Drift.
Электронная почта : король делового общения, электронная почта по-прежнему остается самым популярным инструментом для управления разговорами с клиентами и членами команды.
Опросы : обычно используется для сбора отзывов об обслуживании клиентов, продуктах или для проведения маркетинговых исследований, таких как Typeform, Google Forms и SurveyMonkey.
NPS (Net Promoter Score): один из самых популярных показателей качества обслуживания клиентов в мире. Многие компании используют программное обеспечение для отслеживания NPS для сбора и анализа отзывов своих клиентов.
Вот несколько примеров: Delighted, Promoter.io и Satismeter.
Базы данных : база данных — это совокупность информации.Используя систему управления базами данных, компания может хранить, управлять и анализировать все виды данных. Примеры баз данных включают Postgres, MongoDB и MySQL.
Product Analytics : обратная связь и информация о взаимодействии клиента с вашим продуктом или услугой. Полезно понимать путь клиента и принимать решения на основе данных. ProductBoard и UserVoice — два инструмента, которые вы можете использовать для обработки продуктовой аналитики.
Внешние данные
Это текстовые данные о вашем бренде или товарах со всего Интернета.Вы можете использовать инструменты веб-парсинга, API-интерфейсы и открытые наборы данных для сбора внешних данных из социальных сетей, новостных отчетов, онлайн-обзоров, форумов и т. Д. И анализа их с помощью моделей машинного обучения.
Инструменты для парсинга веб-страниц:
Инструменты для парсинга веб-сайтов : вы можете создать свой собственный парсер, даже не имея опыта программирования, с помощью таких инструментов, как. Dexi.io, Portia и ParseHub.e.
Фреймворки парсинга веб-страниц : опытные программисты могут воспользоваться такими инструментами, как Scrapy в Python и Wombat в Ruby, для создания собственных скребков.
API-интерфейсы
Facebook, Twitter и Instagram, например, имеют свои собственные API-интерфейсы и позволяют извлекать данные с их платформ. Основные средства массовой информации, такие как New York Times или The Guardian, также имеют свои собственные API-интерфейсы, и вы можете использовать их, среди прочего, для поиска в их архиве или сбора комментариев пользователей.
Интеграции
Инструменты SaaS, такие как MonkeyLearn, предлагают интеграцию с инструментами, которые вы уже используете. Вы можете напрямую подключаться к Twitter, Google Sheets, Gmail, Zendesk, SurveyMonkey, Rapidminer и другим.И выполните текстовый анализ данных Excel, загрузив файл.
Подготовка данных
Чтобы автоматически анализировать текст с помощью машинного обучения, вам необходимо организовать свои данные. Большая часть этого делается автоматически, и вы даже не заметите этого. Однако важно понимать, что автоматический анализ текста использует ряд методов обработки естественного языка (НЛП), как показано ниже.
Токенизация, тегирование части речи и анализ
Токенизация — это процесс разбиения строки символов на семантически значимые части, которые могут быть проанализированы (например,g., слова), отбрасывая бессмысленные фрагменты (например, пробелы).
В примерах ниже показаны два различных способа токенизации строки «Анализировать текст не так сложно» .
(Неверно): анализировать текст не так сложно. = [«Analyz», «ing text», «is n», «ot that», «hard».]
(правильно): Анализировать текст не так сложно. = [«Анализируем», «текст», «есть», «нет», «это», «сложно», «.»]
После того, как токены были распознаны, пора классифицировать их.Маркировка части речи относится к процессу присвоения грамматической категории, такой как существительное, глагол и т. Д., Для обнаруженных токенов.
Вот теги PoS токенов из приведенного выше предложения:
«Анализ»: ГЛАГОЛ, «текст»: СУЩЕСТВИТЕЛЬНОЕ, «есть»: ГЛАГОЛ, «не»: ADV, «тот»: ADV, «жесткий» : ADJ, «.»: PUNCT
Со всеми категоризированными токенами и языковой моделью (то есть грамматикой) система теперь может создавать более сложные представления текстов, которые она будет анализировать. Этот процесс известен как синтаксический анализ .Другими словами, синтаксический анализ относится к процессу определения синтаксической структуры текста. Для этого алгоритм синтаксического анализа использует грамматику языка, на котором был написан текст. Разные представления будут результатом синтаксического анализа одного и того же текста с разными грамматиками.
В приведенных ниже примерах показаны зависимости и постоянные представления предложения «Анализировать текст не так сложно» .
Анализ зависимостей
Грамматики зависимостей можно определить как грамматики, которые устанавливают направленные отношения между словами предложений. Анализ зависимостей — это процесс использования грамматики зависимостей для определения синтаксической структуры предложения:
Анализ группы интересов
Грамматики структуры фраз группы моделируют синтаксические структуры, используя абстрактные узлы, связанные со словами и другими абстрактными категориями (в зависимости от типа грамматики), и неориентированные отношения между ними. Синтаксический анализ избирательного округа относится к процессу использования грамматики избирательного округа для определения синтаксической структуры предложения:
Как вы можете видеть на изображениях выше, выходные данные алгоритмов синтаксического анализа содержат большой объем информации, которая может помочь вам понять синтаксическую (и некоторую семантическую) сложность текста, который вы собираетесь анализировать.
В зависимости от решаемой проблемы вы можете попробовать разные стратегии и методы синтаксического анализа. Однако в настоящее время синтаксический анализ зависимостей превосходит другие подходы.
Лемматизация и стемминг
Стемминг и лемматизация относятся к процессу удаления всех аффиксов (т. Е. Суффиксов, префиксов и т. Д.), Прикрепленных к слову, чтобы сохранить его лексическую основу, также известную как корень или ствол или его словарная форма или le mma .Основное различие между этими двумя процессами заключается в том, что , основание обычно основано на правилах, которые обрезают начало и окончание слов (и иногда приводят к несколько странным результатам), тогда как лемматизация использует словари и гораздо более сложный морфологический анализ.
В таблице ниже показаны результаты работы NLTK Snowball Stemmer и лемматизатора Spacy для токенов в предложении «Анализировать текст не так сложно» . Различия в выводе выделены жирным шрифтом:
Удаление стоп-слова
Чтобы обеспечить более точный автоматический анализ текста, нам нужно удалить слова, которые предоставляют очень мало семантической информации или вообще не имеют смысла.Эти слова также известны как стоп-слов: а, и, или, и т. Д.
Для каждого языка существует множество различных списков стоп-слов. Однако важно понимать, что вам может потребоваться добавить слова или удалить слова из этих списков в зависимости от текстов, которые вы хотите проанализировать, и анализа, который вы хотите выполнить.
Возможно, вы захотите провести какой-то лексический анализ области, из которой происходят ваши тексты, чтобы определить слова, которые следует добавить в список запрещенных слов.
Анализ текстовых данных
Теперь, когда вы узнали, как анализировать неструктурированные текстовые данные и основы подготовки данных, как вы анализируете весь этот текст?
Что ж, анализ неструктурированного текста — непростая задача. Существует бесчисленное множество методов анализа текста, но два из них — это классификация текста и извлечение текста.
Классификация текста
Классификация текста (также известная как классификация текста или тегирование текста ) относится к процессу присвоения тегов текстам на основе их содержимого.
Раньше классификация текста выполнялась вручную, что было трудоемким, неэффективным и неточным. Но модели автоматизированного машинного обучения для анализа текста часто работают всего за секунды с непревзойденной точностью.
К наиболее популярным задачам классификации текста относятся анализ тональности (то есть определение того, когда в тексте говорится что-то положительное или отрицательное о данной теме), определение темы (то есть определение того, о каких темах говорится в тексте) и обнаружение намерения (то есть определение цели или основной смысл текста), среди прочего, но есть гораздо больше приложений, которые могут вас заинтересовать.
Системы, основанные на правилах
В классификации текста правило , по сути, представляет собой созданную человеком ассоциацию между лингвистическим шаблоном, который можно найти в тексте, и тегом. Правила обычно состоят из ссылок на морфологические, лексические или синтаксические шаблоны, но они также могут содержать ссылки на другие компоненты языка, такие как семантика или фонология.
Вот пример простого правила классификации описаний продуктов по типу продукта, описанному в тексте:
(HDD | RAM | SSD | Memory) → Hardware
В этом случае система назначит Hardware теги к тем текстам, которые содержат слова HDD , RAM , SSD или Memory .
Наиболее очевидным преимуществом систем, основанных на правилах, является то, что они легко понятны людям. Однако создание сложных систем, основанных на правилах, требует много времени и хороших знаний как в лингвистике, так и в тематике текстов, которые система должна анализировать.
Кроме того, системы, основанные на правилах, сложно масштабировать и поддерживать, потому что добавление новых правил или изменение существующих требует большого анализа и тестирования влияния этих изменений на результаты прогнозов.
Системы на основе машинного обучения
Системы на основе машинного обучения могут делать прогнозы на основе того, что они узнают из прошлых наблюдений. В эти системы необходимо подавать несколько примеров текстов и ожидаемых прогнозов (тегов) для каждого из них. Это называется обучающими данными . Чем более согласованными и точными будут ваши данные о тренировках, тем точнее будут окончательные прогнозы.
При обучении классификатора на основе машинного обучения данные обучения необходимо преобразовать во что-то, что может понять машина, то есть в вектора (т.е. списки чисел, кодирующих информацию). Используя векторы, система может извлекать соответствующие функции (фрагменты информации), которые помогут ей извлекать уроки из существующих данных и делать прогнозы относительно будущих текстов.
Есть несколько способов сделать это, но один из наиболее часто используемых — это пакет слов, векторизация . Вы можете узнать больше о векторизации здесь.
После преобразования текстов в векторы они загружаются в алгоритм машинного обучения вместе с ожидаемыми результатами для создания модели классификации, которая может выбирать, какие функции лучше всего представляют тексты, и делать прогнозы относительно невидимых текстов:
Обученная модель преобразует невидимый текст в вектор, извлечет его соответствующие функции и сделает прогноз:
Алгоритмы машинного обучения
Существует множество алгоритмов машинного обучения, используемых при классификации текста.Наиболее часто используются семейство алгоритмов Naive Bayes (NB), , машины опорных векторов (SVM) и алгоритмы глубокого обучения.
Наивное семейство алгоритмов Байеса основано на теореме Байеса и условных вероятностях появления слов образца текста в словах набора текстов, принадлежащих данному тегу. Векторы, представляющие тексты, кодируют информацию о том, насколько вероятно, что слова в тексте встретятся в текстах данного тега.С помощью этой информации можно вычислить вероятность принадлежности текста любому заданному тегу в модели. После того, как все вероятности были вычислены для входного текста, модель классификации вернет тег с наибольшей вероятностью в качестве выходных данных для этого входного текста.
Одним из основных преимуществ этого алгоритма является то, что результаты могут быть довольно хорошими даже при небольшом количестве обучающих данных.
Машины опорных векторов (SVM) — это алгоритм, который может разделить векторное пространство помеченных текстов на два подпространства: одно пространство, которое содержит большинство векторов, принадлежащих данному тегу, и другое подпространство, которое содержит большинство векторов, которые не принадлежат этому одному тегу.
Классификационные модели, использующие SVM в своей основе, будут преобразовывать тексты в векторы и определять, к какой стороне границы, разделяющей векторное пространство для данного тега, принадлежат эти векторы. В зависимости от того, где они приземляются, модель будет знать, принадлежат ли они данному тегу или нет.
Самым важным преимуществом использования SVM является то, что результаты обычно лучше, чем результаты, полученные с помощью наивного байесовского метода. Однако для SVM требуется больше вычислительных ресурсов.
Глубокое обучение — это набор алгоритмов и методов, которые используют «искусственные нейронные сети» для обработки данных во многом так же, как это делает человеческий мозг.Эти алгоритмы используют огромные объемы обучающих данных (миллионы примеров) для создания семантически богатых представлений текстов, которые затем могут быть введены в модели на основе машинного обучения различного типа, которые будут делать гораздо более точные прогнозы, чем традиционные модели машинного обучения:
Гибридные системы
Гибридные системы обычно содержат в своей основе системы на основе машинного обучения и системы на основе правил для улучшения прогнозов
Оценка
Производительность классификатора обычно оценивается с помощью стандартных показателей, используемых в области машинного обучения: точность , точность , отзыв и оценка F1 .Понимание того, что они означают, даст вам более четкое представление о том, насколько хороши ваши классификаторы при анализе ваших текстов.
Также важно понимать, что оценка может выполняться на фиксированном тестовом наборе (то есть наборе текстов, для которого нам известны ожидаемые выходные теги) или с помощью перекрестной проверки (то есть метода, который разделяет ваши обучающие данные в разные сгибы, чтобы вы могли использовать некоторые подмножества своих данных для целей обучения, а некоторые — для целей тестирования, см. ниже).
Оценка точности, точности, отзыва и F1
Точность — это количество правильных прогнозов, сделанных классификатором, деленное на общее количество прогнозов. В общем, точность сама по себе не является хорошим показателем производительности. Например, когда категории несбалансированы, то есть когда есть одна категория, которая содержит намного больше примеров, чем все другие, прогнозирование всех текстов как принадлежащих этой категории вернет высокий уровень точности. Это известно как парадокс точности.Чтобы получить лучшее представление о производительности классификатора, вы можете вместо этого рассмотреть точность и отзыв.
Точность указывает, сколько текстов было предсказано правильно из тех, которые были предсказаны как принадлежащие данному тегу. Другими словами, точность берет количество текстов, которые были правильно предсказаны как положительные для данного тега, и делит его на количество текстов, которые были предсказаны (правильно и неправильно) как принадлежащие этому тегу.
Мы должны помнить, что точность дает информацию только в тех случаях, когда классификатор предсказывает, что текст принадлежит данному тегу.Это может быть особенно важно, например, если вы хотите генерировать автоматические ответы на пользовательские сообщения. В этом случае, прежде чем отправлять автоматический ответ, вы хотите знать наверняка, что отправите правильный ответ, верно? Другими словами, если ваш классификатор говорит, что сообщение пользователя принадлежит определенному типу сообщения, вы хотите, чтобы классификатор сделал правильное предположение. Это означает, что вам нужна высокая точность для этого типа сообщения.
Напомним, что указывает, сколько текстов было предсказано правильно из тех, которые должны были быть предсказаны как принадлежащие данному тегу.Другими словами, функция отзыва берет количество текстов, которые были правильно предсказаны как положительные для данного тега, и делит его на количество текстов, которые были либо правильно предсказаны как принадлежащие тегу, либо которые были неверно предсказаны как не принадлежащие тегу.
Отзыв может оказаться полезным при маршрутизации заявок в службу поддержки, например, соответствующей группе. Может быть желательно, чтобы автоматизированная система обнаруживала как можно больше заявок для критического тега (например, заявок около «Беспорядки / время простоя» ) за счет выполнения некоторых неверных прогнозов в процессе.В этом случае прогнозирование поможет выполнить начальную маршрутизацию и как можно скорее решить большинство этих критических проблем. Если прогноз неверен, билет будет перенаправлен членом команды. При обработке тысяч заявок в неделю высокий уровень отзыва (конечно же, с хорошим уровнем точности) может сэкономить командам поддержки много времени и позволить им быстрее решать критические проблемы.
Оценка F1 — гармоничное средство точности и отзывчивости. Он сообщает вам, насколько хорошо работает ваш классификатор, если одинаковое значение придается точности и отзыву.В целом, оценка F1 является гораздо лучшим показателем эффективности классификатора, чем точность.
Перекрестная проверка
Перекрестная проверка довольно часто используется для оценки производительности текстовых классификаторов. Метод прост. Прежде всего, обучающий набор данных случайным образом разбивается на несколько подмножеств одинаковой длины (например, 4 подмножества по 25% исходных данных каждое). Затем все подмножества, кроме одного, используются для обучения классификатора (в данном случае 3 подмножества с 75% исходных данных), и этот классификатор используется для прогнозирования текстов в оставшемся подмножестве.Затем вычисляются все показатели производительности (то есть точность, точность, отзыв, F1 и т. Д.). Наконец, процесс повторяется с новой тестовой складкой до тех пор, пока все складки не будут использованы для целей тестирования.
После использования всех складок вычисляются средние показатели производительности и процесс оценки завершается.
Извлечение текста относится к процессу распознавания структурированной информации из неструктурированного текста. Например, может быть полезно автоматически определять наиболее релевантные ключевые слова из фрагмента текста, определять названия компаний в новостной статье, определять арендодателей и арендаторов в финансовом контракте или определять цены в описаниях продуктов.
Регулярные выражения
Регулярные выражения (также известные как регулярные выражения) работают как эквивалент правил, определенных в задачах классификации. В этом случае регулярное выражение определяет шаблон символов, который будет связан с тегом.
Например, приведенный ниже шаблон обнаружит большинство адресов электронной почты в тексте, если им предшествуют и следуют пробелы:
(? I) \ b (?: [A-zA-Z0-9 _-.] +) @ ( ?: (?: [[0-9] {1,3}. [0-9] {1,3}. [0-9] {1,3}.) | (?: (?: [A- zA-Z0-9 -] +.) +)) (?: [a-zA-Z] {2,4} | [0-9] {1,3}) (?:]?) \ b
Обнаружив это совпадение в текстах и присвоив ему тег email , мы можем создать элементарное средство извлечения адресов электронной почты.
У такого подхода есть очевидные плюсы и минусы. С другой стороны, вы можете быстро создавать экстракторы текста и получать хорошие результаты при условии, что вы можете найти правильные шаблоны для того типа информации, которую хотите обнаружить. С другой стороны, регулярные выражения могут быть чрезвычайно сложными и их может быть действительно сложно поддерживать и масштабировать, особенно когда требуется много выражений для извлечения желаемых шаблонов.
Условные случайные поля
Условные случайные поля (CRF) — это статистический подход, часто используемый при извлечении текста на основе машинного обучения.Этот подход изучает образцы, которые должны быть извлечены, путем взвешивания набора характеристик последовательностей слов, которые появляются в тексте. Используя CRF, мы можем добавить несколько переменных, которые зависят друг от друга, к шаблонам, которые мы используем для обнаружения информации в текстах, такой как синтаксическая или семантическая информация.
Это обычно генерирует гораздо более богатые и сложные шаблоны, чем использование регулярных выражений, и потенциально может кодировать гораздо больше информации. Однако для его реализации требуются дополнительные вычислительные ресурсы, поскольку все функции должны быть рассчитаны для всех рассматриваемых последовательностей, и все веса, присвоенные этим функциям, должны быть изучены, прежде чем определять, должна ли последовательность принадлежать тегу. или нет.
Одним из основных преимуществ подхода CRF является его способность к обобщению. После того, как экстрактор был обучен с использованием подхода CRF для текстов определенной области, он будет иметь возможность достаточно хорошо обобщить то, что он изучил, на другие области.
Экстракторы иногда оцениваются путем вычисления тех же стандартных показателей производительности, которые мы объяснили выше для классификации текста, а именно: точность , точность , отзыв и оценка F1 .Однако эти показатели не учитывают частичное совпадение шаблонов. Чтобы извлеченный сегмент был действительно положительным для тега, он должен идеально совпадать с сегментом, который должен был быть извлечен.
Рассмотрим следующий пример:
‘Ваш рейс отправится 14 января 2020 года в 15:30 из SFO’
Если мы создали средство извлечения даты, мы ожидаем, что он вернется 14 января 2020 года в виде дата из текста выше, верно? Итак, если бы вывод экстрактора был 14 января 2020 г., мы бы посчитали его истинным положительным результатом для тега DATE .
А что, если бы выход экстрактора был 14 января? Вы бы сказали, что добыча была плохой? Вы бы сказали, что для тега DATE это было ложное срабатывание? Чтобы зафиксировать частичные совпадения, подобные этому, можно использовать некоторые другие показатели производительности для оценки производительности экстракторов. Одним из примеров этого является семейство показателей ROUGE.
ROUGE (ориентированный на отзыв дублер для оценки Gisting) — это семейство показателей, используемых в областях машинного перевода и автоматического резюмирования, которые также можно использовать для оценки производительности экстракторов текста.Эти метрики в основном вычисляют длину и количество последовательностей, которые перекрываются между исходным текстом (в данном случае нашим исходным текстом) и переведенным или обобщенным текстом (в данном случае нашим извлечением).
В зависимости от длины единиц, перекрытие которых вы хотите сравнить, вы можете определить метрики ROUGE-n (для единиц длины n ) или вы можете определить метрику ROUGE-LCS или ROUGE-L, если вы намереваетесь сравнить самую длинную общую последовательность (LCS).
Визуализация текстовых данных
Теперь вы знаете множество методов анализа текста для разбивки данных, но что вы делаете с результатами? Инструменты бизнес-аналитики (BI) и визуализации данных позволяют легко понять ваши результаты на поразительных информационных панелях, выявить закономерности, тенденции и незамедлительно дать действенную информацию в общих чертах или мельчайших деталях.
Визуализация данных повышает ценность результатов интеллектуального анализа текста за счет преобразования сложных концепций в убедительные и легкие для понимания визуальные эффекты. Все дело в высококачественной аналитической информации, которая приводит к умным бизнес-решениям, основанным на данных!
MonkeyLearn Studio — это универсальный инструмент для сбора, анализа и визуализации данных. Методы машинного обучения глубокого обучения позволяют выбрать необходимый анализ текста (извлечение ключевых слов, анализ тональности, классификация аспектов и т. Д.) И объединить их вместе для одновременной работы.
Вы сразу поймете важность текстовой аналитики. Просто загрузите свои данные и визуализируйте результаты, чтобы получить ценные сведения. Все это работает вместе в едином интерфейсе, поэтому вам больше не нужно выгружать и скачивать между приложениями.
Взгляните на панель инструментов MonkeyLearn Studio ниже, где мы провели анализ настроений на основе аспектов по отзывам клиентов о Zoom. Обзоры сначала сортируются по «аспектам» или категориям (удобство использования, поддержка, надежность и т. Д.), Затем каждая категория анализируется по настроениям, чтобы показать полярность мнений.
Вы можете видеть, что отдельные обзоры организованы по дате и времени, поэтому вы можете отслеживать аспекты и настроения, поскольку они меняются с течением времени. Представьте, что этот анализ используется для тысяч упоминаний вашего бренда в социальных сетях, в обзорах продуктов или в обращениях в службу поддержки клиентов.
Теперь вы можете поиграть с общедоступной информационной панелью MonkeyLearn Studio, чтобы убедиться, насколько легко ею пользоваться. Поиск по индивидуальному настроению, дате, категории и т. Д. Самое лучшее в MonkeyLearn Studio — это то, что вы можете добавлять или удалять анализы, добавлять новые данные и изменять визуализации прямо на панели инструментов.
Бесплатный инструмент визуализации Google позволяет создавать интерактивные отчеты с использованием самых разных данных. После импорта данных вы можете использовать различные инструменты для создания отчета и превращения данных в впечатляющую визуальную историю. Делитесь результатами с отдельными людьми или группами, публикуйте их в Интернете или встраивайте на свой веб-сайт.
Looker — это платформа для анализа бизнес-данных, предназначенная для передачи значимых данных любому сотруднику компании. Идея состоит в том, чтобы позволить командам получить более полное представление о том, что происходит в их компании.
Вы можете подключаться к различным базам данных и автоматически создавать модели данных, которые можно полностью настроить в соответствии с конкретными потребностями. Взгляните сюда, чтобы начать.
Tableau — это инструмент бизнес-аналитики и визуализации данных с интуитивно понятным и удобным для пользователя подходом (не требуется технических навыков). Tableau позволяет организациям работать практически с любым существующим источником данных и предоставляет мощные возможности визуализации с более продвинутыми инструментами для разработчиков.
Для всех, кто хочет попробовать, доступна пробная версия.Узнайте, как выполнять анализ текста в Tableau.
Приложения и примеры анализа текста
Знаете ли вы, что 80% бизнес-данных — это текст? Текст присутствует во всех основных бизнес-процессах, от заявок в службу поддержки до отзывов о продуктах и онлайн-взаимодействий с клиентами. Автоматический анализ текста в реальном времени может помочь вам справиться со всеми этими данными с помощью широкого спектра бизнес-приложений и вариантов использования. Повысьте эффективность и уменьшите количество повторяющихся задач, которые часто имеют большое влияние на текучесть кадров.Лучшее понимание мнения клиентов без необходимости разбирать миллионы сообщений в социальных сетях, онлайн-обзоры и ответы на опросы.
Если вы работаете в сфере обслуживания клиентов, продуктов, маркетинга или продаж, существует ряд приложений для анализа текста, которые автоматизируют процессы и позволяют получать информацию из реального мира. И, что самое главное, для этого вам не понадобится опыт в области науки о данных или инженерии.
Мониторинг социальных сетей
Допустим, вы работаете в Uber и хотите знать, что пользователи говорят о бренде.Вы читали положительные и отрицательные отзывы в Twitter и Facebook. Но каждый день отправляется 500 миллионов твитов, а Uber ежемесячно получает тысячи упоминаний в социальных сетях. Можете ли вы представить себе анализ всех их вручную?
Здесь на помощь приходит анализ тональности, чтобы проанализировать мнение о данном тексте. Анализируя ваши упоминания в социальных сетях с помощью модели анализа настроений, вы можете автоматически разделить их на положительных , нейтральных или отрицательных .Затем пропустите их через анализатор тем, чтобы понять тему каждого текста. Запуская аспектно-ориентированный анализ настроений, вы можете автоматически определять причины положительных или отрицательных упоминаний и получать такую информацию, как:
- Каковы основные жалобы на Uber в социальных сетях?
- Уровень успешности обслуживания клиентов Uber — люди довольны или недовольны этим?
- Что нравится пользователям Uber в сервисе, когда они упоминают Uber положительно?
Допустим, вы только что добавили новую услугу в Uber.Например, Uber Eats. Это решающий момент, и ваша компания хочет знать, что люди говорят о Uber Eats, чтобы вы могли как можно скорее исправить любые сбои и усовершенствовать лучшие функции. Вы также можете использовать аспектно-ориентированный анализ настроений в своих профилях в Facebook, Instagram и Twitter для любых упоминаний Uber Eats и обнаружения таких вещей, как:
- Довольны ли люди до сих пор с Uber Eats?
- Какую проблему нужно исправить наиболее срочно?
- Как мы можем включить положительные истории в нашу маркетинговую и PR-коммуникацию?
Вы можете использовать анализ текста не только для отслеживания упоминаний вашего бренда в социальных сетях, но и для отслеживания упоминаний ваших конкурентов.Клиент жалуется на услуги конкурента? Это дает вам возможность привлечь потенциальных клиентов и показать им, насколько лучше ваш бренд.
Мониторинг бренда
Следите за комментариями о вашем бренде в режиме реального времени, где бы они ни появлялись (социальные сети, форумы, блоги, сайты обзоров и т. Д.). Вы сразу поймете, когда возникнет что-то негативное, и сможете использовать положительные комментарии в своих интересах.
Сила отрицательных отзывов достаточно велика: 40% потребителей откладывают покупку, если у компании есть отрицательные отзывы.Рассерженный клиент, жалующийся на плохое обслуживание клиентов, может распространяться как лесной пожар в считанные минуты: друг делится этим, затем другой, затем еще один . .. И прежде, чем вы это узнаете, негативные комментарии стали вирусными.
- Узнайте, как репутация вашего бренда меняется с течением времени.
- Сравните репутацию вашего бренда с репутацией вашего конкурента.
- Определите, какие аспекты наносят ущерб вашей репутации.
- Определите, какие элементы повышают репутацию вашего бренда в онлайн-СМИ.
- Определите потенциальные PR-кризисы, чтобы вы могли справиться с ними как можно скорее.
- Подключайтесь к данным за определенный момент, например, день запуска нового продукта или подачи заявки на IPO. Просто проведите анализ настроений в социальных сетях и упоминания в прессе в этот день, чтобы узнать, что люди говорят о вашем бренде.
- Публикуйте положительные отзывы о своем бренде, чтобы привлечь внимание к себе.
Служба поддержки клиентов
Несмотря на опасения и ожидания многих людей, анализ текста не означает, что обслуживание клиентов будет полностью автоматизировано.Это просто означает, что компании могут оптимизировать процессы, чтобы команды могли тратить больше времени на решение проблем, требующих человеческого взаимодействия. Таким образом компании смогут увеличить удержание, учитывая, что 89 процентов клиентов меняют бренды из-за плохого обслуживания клиентов. Но как анализ текста может помочь службе поддержки вашей компании?
Маркировка билетов
Позвольте машинам делать всю работу за вас. Анализ текста автоматически определяет темы и маркирует каждый тикет. Вот как это работает:
- Модель анализирует язык и выражения языка клиента, например, «Я получил неправильный заказ.”
- Затем он сравнивает его с другими подобными разговорами.
- Наконец, он находит совпадение и автоматически маркирует билет. В этом случае это может быть тег Shipping Problems .
Это происходит автоматически при поступлении нового запроса, освобождая агентов клиентов, чтобы они могли сосредоточиться на более важных задачах.
Маршрутизация и сортировка билетов: найдите подходящего человека для работы
Машинное обучение может считывать заявку на предмет или срочность и автоматически направлять ее в соответствующий отдел или сотрудника.
Например, для SaaS-компании, которая получает билет клиента с просьбой о возмещении, система интеллектуального анализа текста определит, какая команда обычно занимается вопросами выставления счетов, и отправит им билет. Если в билете написано что-то вроде «Как мне интегрировать ваш API с python?» , он пойдет прямо к команде, отвечающей за помощь в интеграции.
Аналитика билетов: узнавайте больше от своих клиентов
Что обычно оценивается для определения эффективности работы группы обслуживания клиентов? Общие KPI: время первого отклика , среднее время до разрешения (т.е.е. сколько времени требуется вашей команде для решения проблем) и удовлетворенность клиентов (CSAT). И, давайте посмотрим правде в глаза, общая удовлетворенность клиентов во многом зависит от первых двух показателей.
Но как мы можем получить актуальную информацию CSAT из разговоров с клиентами? Как мы можем определить, доволен ли клиент способ решения проблемы? Или если они выразили разочарование по поводу решения проблемы?
В этой ситуации можно использовать анализ тональности на основе аспектов. Этот тип анализа текста исследует чувства и темы, стоящие за словами по различным каналам поддержки, таким как заявки в службу поддержки, разговоры в чате, электронные письма и опросы CSAT.Модель анализа текста может понимать слова или выражения, чтобы определить взаимодействие со службой поддержки как положительное , отрицательное или нейтральное , понять, что было упомянуто (например, Service или UI / UX ), и даже определить настроения за словами (например, Печаль , Гнев и т. д.).
Выявление срочности: расставьте приоритеты по билетам срочности
«С чего начать?» — это вопрос, который часто задают себе представители службы поддержки клиентов. Срочность, безусловно, хорошая отправная точка, но как определить уровень срочности, не тратя драгоценное время на размышления?
Программное обеспечение для интеллектуального анализа текста может определять уровень срочности заявки клиента и соответствующим образом маркировать ее. Тикеты поддержки со словами и выражениями, обозначающими срочность, например, «как можно скорее» или «сразу же» , должным образом помечены как Priority .
Чтобы увидеть, как анализ текста работает для определения срочности, ознакомьтесь с этой демонстрационной моделью обнаружения срочности MonkeyLearn.
Голос клиента (VoC) и отзывы клиентов
Когда вы получаете клиента, ключевым моментом становится его удержание, поскольку привлечение новых клиентов обходится в 5-25 раз дороже, чем удержание тех, которые у вас уже есть. Вот почему пристальное внимание к голосу клиента может дать вашей компании четкое представление об уровне удовлетворенности клиентов и, как следствие, их удержании. Кроме того, это может дать вам полезную информацию, чтобы расставить приоритеты в дорожной карте продукта с точки зрения клиента.
Анализ ответов NPS
Возможно, у вашего бренда уже есть опрос об удовлетворенности клиентов, наиболее распространенным из которых является Net Promoter Score (NPS). В этом опросе задается вопрос: «Насколько вероятно, что вы порекомендуете [бренд] другу или коллеге?» . Ответ — оценка от 0 до 10, и результат делится на три группы: промоутеры , пассивные и недоброжелатели .
Но вот и сложная часть: в конце есть открытый вопрос-ответ. «Почему вы выбрали Х-балл?» Ответ может дать вашей компании бесценную информацию.Без текста вам остается только догадываться, что пошло не так. А теперь, благодаря анализу текста, вам больше не нужно вручную читать эти открытые ответы.
Вы можете делать то же, что и Promoter.io: извлекать основные ключевые слова из отзывов ваших клиентов, чтобы понять, что хвалят или критикуют в вашем продукте. Упоминается ли ключевое слово «Продукт» в основном промоутерами или недоброжелателями? Обладая этой информацией, вы сможете использовать свое время, чтобы получить максимальную отдачу от ответов NPS и начать действовать.
Другой вариант — следовать по стопам Retently, используя анализ текста для классификации ваших отзывов по различным темам, таким как Поддержка клиентов, Дизайн продукта, и Характеристики продукта, затем анализируйте каждый тег с анализом настроений, чтобы увидеть, насколько положительно или отрицательно клиенты чувствовать по каждой теме. Теперь они знают, что они на правильном пути с дизайном продукта, но им еще нужно работать над функциями продукта.
Анализ опросов клиентов
Есть ли у вашей компании другая система опросов клиентов? Если это система баллов или закрытые вопросы, проанализировать ответы будет несложно: просто вычислите числа.
Однако, если у вас есть опрос с открытым текстом, независимо от того, предоставляется ли он по электронной почте или в виде онлайн-формы, вы можете отказаться от ручной пометки каждого отдельного ответа, позволив анализу текста сделать эту работу за вас. Помимо экономии времени, вы также можете иметь согласованные критерии маркировки без ошибок, 24/7.
Business Intelligence
Анализ данных лежит в основе каждой операции бизнес-аналитики. Итак, что может сделать компания, чтобы понять, например, тенденции продаж и показатели с течением времени? С помощью числовых данных команда бизнес-аналитики может определить, что происходит (например, продажи X снижаются), но не , почему .Цифры легко анализировать, но они также несколько ограничены. С другой стороны, текстовые данные являются наиболее распространенным форматом деловой информации и могут предоставить вашей организации ценное представление о ваших операциях. Анализ текста с помощью машинного обучения может автоматически анализировать эти данные для немедленного анализа.
Например, вы можете запустить извлечение ключевых слов и анализ настроений в ваших упоминаниях в социальных сетях, чтобы понять, на что люди жалуются на ваш бренд.
Вы также можете запустить анализ настроений на основе аспектов для отзывов клиентов, в которых упоминается плохое качество обслуживания клиентов. В конце концов, 67% потребителей называют плохой клиентский опыт одной из основных причин ухода. Может быть, это плохая поддержка, неисправная функция, неожиданный простой или внезапное изменение цены. Анализ отзывов клиентов может пролить свет на детали, и команда сможет принять соответствующие меры.
А как же ваши конкуренты? Что говорят их обзоры? Пропустите их через свою модель анализа текста и посмотрите, что они делают правильно, а что неправильно, и улучшите ваше собственное принятие решений.
Продажи и маркетинг
Поисковые работы — самая сложная часть процесса продаж. И становится все труднее и труднее. Отдел продаж всегда хочет заключать сделки, что требует повышения эффективности процесса продаж. Но 27% торговых агентов тратят более часа в день на ввод данных, а не на продажу, что означает, что критическое время теряется на административную работу, а не на закрытие сделок.
Анализ текста упрощает выполнение ручных задач по продажам, в том числе:
- Обновление статуса сделки как «Не интересует» в вашей CRM.
- Оценка потенциальных клиентов на основе описания компании.
- Выявление потенциальных клиентов в социальных сетях, которые выражают покупательское намерение.
GlassDollar, компания, которая связывает учредителей с потенциальными инвесторами, использует текстовый анализ для поиска наиболее качественных совпадений. Как? Они используют текстовый анализ для классификации компаний по их описаниям. Результаты? Они сэкономили дни ручной работы, и прогнозы были точными на 90% после обучения модели классификации текста.Вы можете узнать больше об их опыте работы с MonkeyLearn здесь.
Анализ текста может не только автоматизировать ручные и утомительные задачи, но также может улучшить вашу аналитику, чтобы сделать воронки продаж и маркетинга более эффективными. Например, вы можете автоматически анализировать ответы на ваши электронные письма и разговоры о продажах, чтобы понять, скажем, падение продаж:
- Какие блоки мешают заключению сделки?
- Что вызывает интерес у покупателя?
- Что беспокоит клиентов?
А теперь представьте, что цель вашего отдела продаж — привлечь новый сегмент для вашего SaaS: людей старше 40 лет.Первое впечатление — товар им не нравится, но почему ? Просто отфильтруйте разговоры о продажах этой возрастной группы и запустите их в своей модели анализа текста. Команды продаж могут принимать более обоснованные решения, используя углубленный текстовый анализ разговоров с клиентами.
Наконец, вы можете использовать машинное обучение и анализ текста, чтобы улучшить общий процесс продаж. Например, Drift, маркетинговая платформа для общения, интегрировала MonkeyLearn API, чтобы позволить получателям автоматически отказываться от рекламных писем в зависимости от того, как они отвечают.
Пора увеличить продажи и перестать тратить драгоценное время на потенциальных клиентов, которые никуда не денутся. Компания Xeneta, занимающаяся морскими перевозками, разработала алгоритм машинного обучения и обучила его определять, какие компании являются потенциальными клиентами, на основе описаний компаний, собранных с помощью FullContact (SaaS-компания, имеющая описания миллионов компаний).
Вы можете сделать то же самое или настроить таргетинг на пользователей, которые посещают ваш веб-сайт, для:
- Получите информацию о том, где работают потенциальные клиенты, используя такую услугу, как Clearbit, и классифицируйте компанию по типу бизнеса, чтобы увидеть, является ли это потенциальным лидером.
- Извлекайте информацию, чтобы легко узнать должность пользователя, компанию, в которой он работает, ее тип бизнеса и другую важную информацию.
- Оттачивайте наиболее квалифицированных потенциальных клиентов и экономьте время на их поиске: торговые представители получат информацию автоматически и сразу же начнут нацеливаться на потенциальных клиентов.
Product Analytics
Представим, что у вашего стартапа есть приложение в магазине Google Play. Вы получаете несколько необычно негативных комментариев.Что происходит?
Вы можете узнать, что происходит за считанные минуты, используя модель анализа текста, которая группирует обзоры по различным тегам, таким как Ease of Use и Integrations. Затем запустите их с помощью модели анализа настроений, чтобы выяснить, положительно или отрицательно отзываются о товарах клиенты. Наконец, с помощью MonkeyLearn Studio можно создавать графики и отчеты для визуализации и определения приоритетов проблем продукта.
Мы сделали это с помощью обзоров Slack с сайта обзора продуктов Capterra и получили довольно интересные выводы.Вот как это сделать:
Мы проанализировали отзывы с помощью аспектно-ориентированного анализа тональности и распределили их по основным темам и настроениям.
Мы извлекли ключевые слова с помощью средства извлечения ключевых слов, чтобы понять, почему отзывы с пометкой «Эффективность-Качество-Надежность» имеют тенденцию быть отрицательными.
Ресурсы для анализа текста
Существует ряд ценных ресурсов, которые помогут вам начать работу со всем, что может предложить анализ текста.
API анализа текста
Вы можете использовать библиотеки с открытым исходным кодом или API SaaS для создания решения для анализа текста, которое соответствует вашим потребностям. Библиотеки с открытым исходным кодом требуют много времени и технических ноу-хау, в то время как инструменты SaaS часто могут быть запущены сразу же и практически не требуют опыта программирования.
Библиотеки с открытым исходным кодом
Python
Python — наиболее широко используемый язык в научных вычислениях, точка. Такие инструменты, как NumPy и SciPy, сделали его быстрым, динамичным языком, который вызывает библиотеки C и Fortran там, где требуется производительность.
Эти вещи в сочетании с процветающим сообществом и разнообразным набором библиотек для реализации моделей обработки естественного языка (NLP) сделали Python одним из наиболее предпочтительных языков программирования для анализа текста.
NLTK
NLTK, набор инструментов для естественного языка, является лучшей в своем классе библиотекой для задач анализа текста. NLTK используется во многих университетских курсах, поэтому на нем написано много кода, и нет недостатка в пользователях, знакомых как с библиотекой, так и с теорией NLP, которые могут помочь ответить на ваши вопросы.
SpaCy
SpaCy — это промышленная статистическая библиотека НЛП. Помимо обычных функций, он добавляет интеграцию с глубоким обучением и модели сверточных нейронных сетей для нескольких языков.
В отличие от NLTK, которая является исследовательской библиотекой, SpaCy стремится быть проверенной на практике производственной библиотекой для анализа текста.
Scikit-learn
Scikit-learn — это полный и зрелый набор инструментов для машинного обучения для Python, построенный на основе NumPy, SciPy и matplotlib, что дает ему отличную производительность и гибкость для построения моделей анализа текста.
TensorFlow
Разработанная Google, TensorFlow на сегодняшний день является наиболее широко используемой библиотекой для распределенного глубокого обучения. Глядя на этот график, мы видим, что TensorFlow опережает конкурентов:
PyTorch
PyTorch — это платформа глубокого обучения, созданная Facebook и специально предназначенная для глубокого обучения. PyTorch — это библиотека, ориентированная на Python, которая позволяет вам определять большую часть архитектуры вашей нейронной сети в терминах кода Python и только внутренне имеет дело с высокопроизводительным кодом более низкого уровня.
Keras
Keras — широко используемая библиотека глубокого обучения, написанная на Python. Он разработан для обеспечения быстрых итераций и экспериментов с глубокими нейронными сетями, и как библиотека Python он уникально удобен для пользователя.
Важной особенностью Keras является то, что он предоставляет, по сути, абстрактный интерфейс для глубоких нейронных сетей. Фактические сети могут работать поверх Tensorflow, Theano или других бэкэндов. Эта внутренняя независимость делает Keras привлекательным вариантом с точки зрения его долгосрочной жизнеспособности.
Разрешающая лицензия MIT делает его привлекательным для предприятий, стремящихся разрабатывать собственные модели.
R
R — лучший язык для решения любых статистических задач. Его коллекция библиотек (13 711 на момент написания статьи о CRAN намного превосходит возможности любого другого языка программирования для статистических вычислений и больше, чем у многих других экосистем. Короче говоря, если вы решите использовать R для чего-либо, связанного со статистикой, вы не будете окажетесь в ситуации, когда вам придется изобретать велосипед, не говоря уже о целой пачке.
Caret
caret — это пакет R, предназначенный для создания полных конвейеров машинного обучения с инструментами для всего, от приема и предварительной обработки данных, выбора функций и автоматической настройки модели.
mlr
Проект «Машинное обучение в R» (сокращенно mlr) предоставляет полный набор инструментов машинного обучения для языка программирования R, который часто используется для анализа текста.
Java
Java не нуждается в представлении. Язык может похвастаться впечатляющей экосистемой, которая выходит за рамки самой Java и включает библиотеки других языков JVM, таких как Scala и Clojure.Помимо этого, JVM протестирована в боевых условиях, на ее разработку и настройку производительности были потрачены тысячи человеко-лет, поэтому Java, вероятно, обеспечит вам лучшую в своем классе производительность для всей вашей работы по НЛП с анализом текста.
CoreNLP
Стэнфордский проект CoreNLP предоставляет проверенный и активно поддерживаемый инструментарий НЛП. Хотя он написан на Java, у него есть API для всех основных языков, включая Python, R и Go.
OpenNLP
Проект Apache OpenNLP — еще один набор инструментов машинного обучения для НЛП.Его можно использовать на любом языке платформы JVM.
Weka
Weka — это библиотека Java для машинного обучения под лицензией GPL, разработанная в Университете Вайкато в Новой Зеландии. В дополнение к исчерпывающему набору API-интерфейсов машинного обучения Weka имеет графический пользовательский интерфейс под названием Explorer , который позволяет пользователям в интерактивном режиме разрабатывать и изучать свои модели.
Weka поддерживает извлечение данных из баз данных SQL напрямую, а также глубокое обучение с помощью платформы deeplearning4j.
API-интерфейсы SaaS
Использование API-интерфейса SaaS для анализа текста имеет множество преимуществ:
Большинство инструментов SaaS представляют собой простые plug-and-play решения без библиотек для установки и без новой инфраструктуры.
API-интерфейсы SaaS предоставляют готовые к использованию решения. Вы даете им данные, и они возвращают анализ. Все остальные проблемы — производительность, масштабируемость, ведение журнала, архитектура, инструменты и т. Д. — перекладываются на сторону, ответственную за поддержку API.
Часто вам просто нужно написать несколько строк кода, чтобы вызвать API и получить обратно результаты.
API-интерфейсы SaaS обычно предоставляют готовые интеграции с инструментами, которые вы, возможно, уже используете. Это позволит вам создать действительно решение без кода. Узнайте, как интегрировать анализ текста с Google Таблицами.
Некоторые из наиболее известных SaaS-решений и API-интерфейсов для анализа текста включают:
В настоящее время ведутся дебаты между сборками и покупками, когда речь идет о приложениях для анализа текста: создайте свой собственный инструмент с помощью программного обеспечения с открытым исходным кодом или используйте Инструмент анализа текста SaaS?
Создание собственного программного обеспечения с нуля может быть эффективным и полезным, если у вас есть годы опыта в области науки о данных и инженерии, но это требует много времени и может стоить сотни тысяч долларов.
Инструменты SaaS, с другой стороны, являются отличным способом погрузиться в дело. Они могут быть простыми, легкими в использовании и столь же мощными, как создание собственной модели с нуля. MonkeyLearn — это платформа для анализа текста SaaS с десятками предварительно обученных моделей. Или вы можете настроить свой собственный, часто всего за несколько шагов для получения столь же точных результатов. И все это без опыта программирования.
Учебные наборы данных
Если вы поговорите с любым специалистом в области науки о данных, он скажет вам, что истинным узким местом для построения лучших моделей являются не новые и лучшие алгоритмы, а больше данных.
Действительно, в машинном обучении данные являются королем: простая модель, учитывая тонны данных, вероятно, превзойдет ту, которая использует все уловки из книги, чтобы превратить каждый бит обучающих данных в осмысленный ответ.
Итак, вот несколько высококачественных наборов данных, которые вы можете использовать для начала работы:
Классификация тем
Набор данных новостей Reuters: один из самых популярных наборов данных для классификации текста; он содержит тысячи статей от Reuters, разделенных на 135 категорий в соответствии с их тематикой, например, «Политика», «Экономика», «Спорт» и «Бизнес».
20 групп новостей: очень известный набор данных, содержащий более 20 тыс. Документов по 20 различным темам.
Анализ настроений
Обзоры продуктов: набор данных с миллионами отзывов покупателей о товарах на Amazon.
Twitter настроения авиакомпаний на Kaggle: еще один широко используемый набор данных для начала работы с анализом настроений. Он содержит более 15 тысяч твитов об авиакомпаниях (помеченных как положительные, нейтральные или отрицательные).
Первые дебаты Республиканской партии в Twitter: еще один полезный набор данных с более чем 14000 помеченных твитов (положительных, нейтральных и отрицательных) из первых дебатов Республиканской партии в 2016 году.
Другие популярные наборы данных
Спамбаза: этот набор данных содержит 4601 сообщение электронной почты, помеченное как спам, а не как спам.
SMS Spam Collection: еще один набор данных для обнаружения спама. Он содержит более 5 тысяч SMS-сообщений, помеченных как спам, а не как спам.
Разжигание ненависти и оскорбительная лексика: набор данных, содержащий более 24 тыс. Помеченных твитов, сгруппированных по трем тегам: чистые, разжигающие ненависть и оскорбительные выражения.
Поиск больших объемов и высококачественных наборов обучающих данных — самая важная часть анализа текста, более важная, чем выбор языка программирования или инструментов для создания моделей. Помните, что конвейер машинного обучения с наилучшей архитектурой бесполезен, если его модели основаны на ненадежных данных.
Учебники по анализу текста
Лучший способ учиться — это делать.
Сначала мы рассмотрим учебники для конкретных языков программирования с использованием инструментов с открытым исходным кодом для анализа текста.Это поможет вам глубже понять доступные инструменты для выбранной вами платформы.
Затем мы рассмотрим пошаговое руководство по MonkeyLearn, чтобы вы могли сразу приступить к анализу текста.
Учебники с использованием библиотек с открытым исходным кодом
В этом разделе мы рассмотрим различные учебные пособия по анализу текста на основных языках программирования для машинного обучения, которые мы перечислили выше.
Python
NLTK
Официальная книга NLTK — это полный ресурс, который учит вас NLTK от начала до конца.Кроме того, справочная документация является полезным ресурсом для консультации во время разработки.
Другие полезные руководства включают:
SpaCy
spaCy 101: все, что вам нужно знать: часть официальной документации, это руководство показывает вам все, что вам нужно знать, чтобы начать использовать SpaCy.
В этом руководстве показано, как создать конвейер WordNet с помощью SpaCy.
Кроме того, есть официальная документация по API, в которой объясняется архитектура и API SpaCy.
Если вы предпочитаете длинный текст, существует ряд книг о SpaCy или с его участием:
Scikit-learn
Официальная документация scikit-learn содержит ряд руководств по базовому использованию scikit-learn, построению конвейеров , и оценивающих оценщиков.
Scikit-learn Tutorial: Machine Learning in Python показывает, как использовать scikit-learn и Pandas для исследования набора данных, его визуализации и обучения модели.
Для читателей, которые предпочитают книги, есть несколько вариантов:
Keras
На официальном веб-сайте Keras есть обширный API, а также учебная документация.Для читателей, которые предпочитают длинный текст, рекомендуется обратиться к книге «Глубокое обучение с Керасом». В книге используются примеры из реальной жизни, чтобы дать вам четкое представление о Керасе.
Другие руководства:
Практическая классификация текста с помощью Python и Keras: в этом руководстве реализована модель анализа настроений с использованием Keras и показано, как обучать, оценивать и улучшать эту модель.
Классификация текста в Keras: в этой статье строится простой классификатор текста для набора данных новостей Reuters.Он классифицирует текст статьи по ряду категорий, таких как спорт, развлечения и технологии.
TensorFlow
TensorFlow Tutorial для начинающих знакомит с математикой, лежащей в основе TensorFlow, и включает примеры кода, которые запускаются в браузере, что идеально подходит для исследования и обучения. Цель урока — классифицировать уличные знаки.
Книга «Практическое машинное обучение с помощью Scikit-Learn и TensorFlow» поможет вам получить интуитивное понимание машинного обучения с помощью TensorFlow и scikit-learn.
Наконец, есть официальное руководство по началу работы с TensorFlow.
PyTorch
Официальное руководство по началу работы от PyTorch показывает вам основы PyTorch. Если вас интересует что-то более практичное, ознакомьтесь с этим руководством по чат-боту; он показывает вам, как создать чат-бота с помощью PyTorch.
Учебник «Глубокое обучение для НЛП с помощью PyTorch» — это мягкое введение в идеи, лежащие в основе глубокого обучения, и их применение в PyTorch.
Наконец, официальный справочник API объясняет функционирование каждого отдельного компонента.
R
Caret
Краткое введение в пакет Caret показывает, как обучать и визуализировать простую модель. Практическое руководство по машинному обучению в R показывает, как подготовить данные, построить и обучить модель, а также оценить ее результаты. Наконец, у вас есть официальная документация, которая очень полезна для начала работы с Caret.
mlr
Для тех, кто предпочитает длинный текст, на arXiv можно найти обширный учебник по MLR. Это больше похоже на книгу, чем на статью, и в ней есть обширные и подробные образцы кода для использования mlr.Также есть официальная шпаргалка по mlr, удобный ресурс для отладки.
Java
CoreNLP
Если вы хотите узнать о CoreNLP, вам следует ознакомиться с учебным пособием Linguisticsweb.org, в котором объясняется, как быстро начать работу и выполнить ряд простых задач NLP из командной строки. Кроме того, в этом руководстве CloudAcademy показано, как использовать CoreNLP и визуализировать его результаты. Вы также можете ознакомиться с этим руководством, посвященным анализу настроений с помощью CoreNLP.Наконец, есть это руководство по использованию CoreNLP с Python, которое полезно для начала работы с этой структурой.
OpenNLP
Перво-наперво: официальное руководство Apache OpenNLP должно быть
отправная точка. Книга «Укрощение текста» была написана разработчиком OpenNLP и использует фреймворк, чтобы показать читателю, как реализовать анализ текста. Более того, это руководство проведет вас по полной экскурсии по OpenNLP, включая токенизацию, часть тегов речи, синтаксический анализ предложений и разбиение на части.
Weka
В библиотеке Weka есть официальная книга «Интеллектуальный анализ данных: практические инструменты и методы машинного обучения», которая пригодится для знакомства с Weka.
Если вы предпочитаете видео тексту, существует также ряд MOOC, использующих Weka:
Практическое руководство по анализу текста с помощью MonkeyLearn
Давайте представим, что производительность вашей службы поддержки падает: вручную классифицируйте темы и отправьте каждый запрос в соответствующий отдел. делая их работу очень сложной.
Вы готовы к использованию решения для машинного обучения, но у вашей команды разработчиков просто нет времени или опыта, и вы не хотите нанимать новых сотрудников.
Хорошая новость заключается в том, что MonkeyLearn предлагает простую и удобную платформу для создания собственных моделей анализа текста без использования кода или машинного обучения.
Следуйте приведенным ниже пошаговым инструкциям по классификации и извлечению текста, чтобы узнать, как начать анализ текста с помощью машинного обучения всего за несколько минут.Это безболезненно и легко.
Создайте свой собственный классификатор текста
1. Создайте новую модель
Сначала перейдите на панель управления и нажмите «Создать модель», затем выберите «Классификатор»:
2. Теперь выберите «Классификация тем»
3. Загрузите данные для обучения классификатора
Первое, что нужно вашей новой модели, — это данные. Вы можете импортировать данные из файлов CSV или Excel или из Twitter, Gmail, Zendesk, Freshdesk и др .:
После загрузки данных вы приступите к обучению своей модели.
4. Определите теги
Следующим шагом является определение тегов, которые вы хотите использовать в текстовом классификаторе при сортировке данных:
5. Начните обучение своей модели, добавив теги к примерам
Теперь отметьте текст по вашим критериям.
Обучить вашу модель очень просто. Просто назначьте соответствующий тег каждому фрагменту текста, как в примере ниже:
Если вы заметите, что некоторые примеры уже помечены, значит машинное обучение выполняет свою работу! Помните, что это первые шаги модели с вашими данными, поэтому она может делать некоторые ошибки на этом пути.Просто поменяйте теги, если что-то не так, и вы увидите более точные результаты в кратчайшие сроки. Чем больше данных вы пометите, тем точнее станет модель.
6. Протестируйте свой классификатор
Последним шагом является проверка классификатора текста. После того, как вы закончите размечать начальный набор примеров, перейдите на вкладку «Выполнить» и введите новый текст, чтобы увидеть, как он работает:
Если вы все еще видите ошибки, вы можете вернуться на вкладку «Сборка» для дальнейшее обучение.
Заставьте ваш новый классификатор текста работать
Теперь, когда ваш новый классификатор тем запущен и работает, все, что вам нужно сделать, это загрузить новые данные и позволить модели делать свое дело.С MonkeyLearn вы можете просто загрузить больше файлов CSV и Excel для анализа новых данных или использовать одну из наших интеграций:
Если вы умеете программировать, вы также можете использовать API MonkeyLearn для запуска модели анализа текста на Python, Ruby, PHP, Javascript или Java:
После того, как ваши данные будут проанализированы, вы сможете увидеть все с поразительной детализацией с помощью MonkeyLearn Studio.
А теперь перейдем к извлечению текста. Предположим, что только что получены результаты вашего последнего опроса об удовлетворенности клиентов, и вам нужно найти способ просеять открытые ответы, чтобы заменить ручные процедуры, которые тратят впустую время.Эти ответы содержат важную информацию о том, как клиенты относятся к вашим услугам. Ваша цель — извлечь из этих ответов наиболее релевантные ключевые слова и получить полезную информацию.
Объедините это с анализом настроений, и вы также узнаете, как они относятся к этим темам: положительно , отрицательно или нейтрально .
1. Создайте новый экстрактор
Войдите в MonkeyLearn и перейдите на свою панель управления, нажмите «Создать модель», а затем «Экстрактор»:
2.Выберите способ загрузки данных модели
Теперь вы можете загружать данные с таких платформ, как Twitter или Gmail, или из служб поддержки клиентов, таких как Front, Zendesk и Freshdesk, а также из RSS и файлов CSV или Excel.
3. Создайте теги для своей модели для извлечения
Вам понадобится как минимум два тега, чтобы начать обучение модели извлечения текста, но вы всегда можете добавить больше по ходу, если чувствуете, что отсутствуют важные данные:
В этом примере выше мы выбираем теги, которые дадут нам представление о том, как клиенты видят три важных аспекта нашего сервиса.Мы извлекли данные из нашего последнего опроса об удовлетворенности с помощью тегов Support , UX / UI и Pricing .
4. Обучите свой новый экстрактор текста
После того, как вы сказали своему экстрактору, на какие теги нужно обращать внимание, пришло время вручную пометить некоторые примеры, выделив слова и выражения, связанные с ними. После небольшого обучения вы начнете замечать, что экстрактор начинает предсказывать теги на основе ваших критериев:
. Вы можете вернуться на вкладку «Построить» и продолжить обучение своей модели, если она не предсказывает должным образом.
Что дальше? Вы готовы использовать новую модель извлечения текста. Вы можете скормить ему новые данные, загрузив новые файлы CSV и Excel или используя одну из интеграций MonkeyLearn:
Как мы упоминали ранее, MonkeyLearn также позволяет интегрировать экстрактор с его API, используя Python, Ruby, PHP, Javascript или Java:
Takeaway
Анализ текста больше не является эксклюзивной темой для инженеров-программистов с опытом машинного обучения. Он стал мощным инструментом, который помогает компаниям во всех отраслях получать полезные и действенные идеи из своих текстовых данных. Экономия времени, автоматизация задач и повышение производительности никогда не были такими простыми, как никогда, позволяя компаниям избавляться от громоздких задач и помогать своим командам предоставлять более качественные услуги своим клиентам.
Если вы хотите попробовать анализ текста, посетите MonkeyLearn и начните обучение своим собственным классификаторам и экстракторам текста — кодирование не требуется благодаря нашему удобному интерфейсу и интеграции.
И взгляните на общедоступную информационную панель MonkeyLearn Studio, чтобы увидеть, что может сделать визуализация данных, чтобы увидеть ваши результаты в общих чертах или в мельчайших деталях.
Свяжитесь с нашей командой, если у вас есть какие-либо сомнения или вопросы об анализе текста и машинном обучении, и мы поможем вам начать работу!
языков программирования: анализ
языков программирования: анализCOS 441 — синтаксический анализ — 8 февраля 1996 г.
Абстрактный синтаксис
Абстрактный синтаксис — это представление программы, которая:
- абстрагирует ненужные детали конкретного синтаксиса;
- сохраняет только достаточно информации, чтобы мы могли назначить значение (семантика) терминов; и
- соответствует структуре BNF языка.
Анализ означает интерпретацию входного потока как термины на доступном языке. Напомним, что мы рассматриваем языковые синтаксис состоит из трех уровней: лексические элементы, контекстно-свободный синтаксис, и контекстно-зависимый синтаксис. Следовательно, мы проанализируем язык рассматривая эти три слоя по отдельности.
Лексический анализатор или токенизатор принимает входной поток символов
и разбивает на жетоны. Для этого курса мы будем использовать Scheme’s
tokenizer, чтобы сделать это за нас.
Парсер берет поток токенов, созданный лексическим анализатором, и создает представление абстрактного синтаксиса программы, называемое абстрактное синтаксическое дерево или дерево синтаксического анализа . Как видите, термин синтаксический анализ часто используется для обозначения простой интерпретации поток токенов в контекстно-свободный синтаксис.
Вернемся к примеру запроса. Запрос:
query :: = Word | НЕ запрос | (запрос И запрос)Чтобы разобрать запросы, мы должны исправить представление для токенов и представление для запросов, т.е. для абстрактного синтаксис запросов. Для токенов, мы будем использовать следующие представления:
Слово - символ НЕ НЕ И И (- "(" ) - ")"Предположим, что у нас есть функция tokenize : ввод -> список токенов который преобразует входной поток в список таких токенов.Предположим, что функции make-Word, make-Not и make-And строить соответствующие представления запросов.
Теперь мы можем написать функцию parse для анализа запросов. Эта функция примет на вход список токенов и вернет пара абстрактного запроса и оставшаяся часть ввода.
(определить синтаксический анализ (лямбда (ввод) (cond ((равно? 'НЕ (ввод автомобиля)) (пусть * ((r (parse (cdr input))) (д (автомобиль г)) (отдых (cdr r))) (минусы (make-Not q) отдыхают))) ((символ? (ввод автомобиля)) (минусы (make-Word (ввод автомобиля)) (ввод cdr))) ((равно? "(" (ввод автомобиля)) (пусть * ((r1 (parse (вход cdr))) (q1 (автомобиль r1)) (rest1 (cdr r1)) (rest2 (cdr rest1)); пропустить "И" (r2 (синтаксический анализ rest2)) (q2 (автомобиль r2)) (rest3 (cdr r2)) (rest4 (cdr rest3))); пропускать ")" (минусы (make-а q1 q2) rest4))) (else (ошибка «Неверный ввод»)))))
Это довольно просто, потому что грамматика запросов — LL0. Но мы можем сделать это еще проще, воспользовавшись
встроенный синтаксический анализатор Scheme для языка, называемого s-выражений .
S-выражения определяются следующим образом:
sexp :: = #t | #f | номер | char | символ | () | нить | (sexp. sexp) | # (sexp *) | (sexp *)S-выражение формы
(sexp. Sexp)
пара; s-выражение вида # (sexp *)
есть
вектор; и (sexp *)
— список. Списки
представлены парами и нулем.S-выражения строятся
на читать
и (цитата из sexp)
,
сокращенно 'sexp
.Если мы теперь немного изменим синтаксис запросов, чтобы запросы являются подмножеством s-выражений, мы можем использовать s-выражение синтаксический анализатор, который сделает за нас часть синтаксического анализа. Давайте переопределим запросы следующим образом:
q :: = слово | (НЕ q) | (И q q)Обратите внимание на круглые скобки, которые теперь требуются вокруг запроса NOT. Наша новая функция синтаксического анализа принимает список токенов и возвращает просто проанализированный запрос:
(определить синтаксический анализ (лямбда (sexp) (cond ((symbol? sexp) (make-Word sexp)) ((пара? sexp) (cond ((равно? 'NOT (car sexp)) (make-Not (parse (cadr sexp)))) ((равно? 'И (автомобиль sexp)) (make-And (parse (cadr sexp)) (parse (caddr sexp)))) (else (ошибка «Неверный ввод»)))) (else (ошибка «Неверный ввод»)))))
Давайте теперь создадим синтаксический анализатор для подмножества Scheme.Мы рассмотрим следующее подмножество:
e :: = #t | #f | () | номер | ... | Икс | (лямбда (x *) e) | (если e e e) | (cond (e e) * [(else e)]) | (e e *)Мы будем представлять токены точно так, как их представляет Схема в s-выражения. Мы используем
define-record
средство для построения представлений абстрактного синтаксиса:(константа определения записи (значение)) (определить-запись Var (имя)) (определение-запись Лам (формальное тело)) (определить-запись Если (проверить, затем еще)) (определение-запись Cond (пункты else)) (определение-запись Ap (забавные аргументы))Каждые
(определение-запись Foo (field1.