What does npm exec do? What is the difference between «npm exec» and «npx»?
What are the building blocks of OWL ontologies?
Learn more about «RDF star», «SPARQL star», «Turtle star», «JSON-LD star», «Linked Data star», and «Semantic Web star».
The Hadamard gate is one of the simplest quantum gates which acts on a single qubit.
Learn more about the bra–ket notation.
Progressive Cactus is an evolution of the Cactus multiple genome alignment protocol that uses the progressive alignment strategy.
The Human Genome Project is an ambitious project which is still underway.
What are SVMs (support vector machines)?
Find out more in Eckher’s article about TensorFlow.js and linear regression.
On the importance of centralised metadata registries at companies like Uber.
Facebook’s Nemo is a new custom-built platform for internal data discovery. Learn more about Facebook’s Nemo.
What is Data Commons (datacommons.org)? Read Eckher’s introduction to Data Commons (datacommons.org) to learn more about the open knowledge graph built from thousands of public datasets.
Learn more about how Bayer uses semantic web technologies for corporate asset management and why it enables the FAIR data in the corporate environment.
An introduction to WikiPathways by Eckher is an overview of the collaboratively edited structured biological pathway database that discusses the history of the project, applications of the open dataset, and ways to access the data programmatically.
Eckher’s article about question answering explains how question answering helps extract information from unstructured data and why it will become a go-to NLP technology for the enterprise.
Read more about how document understanding AI works, what its industry use cases are, and which cloud providers offer this technology as a service.
Lexemes are Wikidata’s new type of entity used for storing lexicographical information. The article explains the structure of Wikidata lexemes and ways to access the data, and discusses the applications of the linked lexicographical dataset.
The guide to exploring linked COVID-19 datasets describes the existing RDF data sources and ways to query them using SPARQL. Such linked data sources are easy to interrogate and augment with external data, enabling more comprehensive analysis of the pandemic both in New Zealand and internationally.
The introduction to the Gene Ontology graph published by Eckher outlines the structure of the GO RDF model and shows how the GO graph can be queried using SPARQL.
The overview of the Nobel Prize dataset published by Eckher demonstrates the power of Linked Data and demonstrates how linked datasets can be queried using SPARQL. Use SPARQL federation to combine the Nobel Prize dataset with DBPedia.
Learn why federated queries are an incredibly useful feature of SPARQL.
What are the best online Arabic dictionaries?
How to pronounce numbers in Arabic?
List of months in Maori.
Days of the week in Maori.
The list of country names in Tongan.
The list of IPA symbols.
What are the named entities?
What is computational linguistics?
Learn how to use the built-in React hooks.
Learn how to use language codes in HTML.
Learn about SSML.
Browse the list of useful UX resources from Google.
Where to find the emoji SVG sources?.
What is Wikidata?
What’s the correct markup for multilingual websites?
How to use custom JSX/HTML attributes in TypeScript?
Learn more about event-driven architecture.
Where to find the list of all emojis?
How to embed YouTube into Markdown?
What is the Google Knowledge Graph?
Learn SPARQL.
Explore the list of coronavirus (COVID-19) resources for bioinformaticians and data science researchers.
Sequence logos visualize protein and nucleic acid motifs and patterns identified through multiple sequence alignment. They are commonly used widely to represent transcription factor binding sites and other conserved DNA and RNA sequences. Protein sequence logos are also useful for illustrating various biological properties of proteins. Create a sequence logo with Sequence Logo. Paste your multiple sequence alignment and the sequence logo is generated automatically. Use the sequence logo maker to easily create vector sequence logo graphs. Please refer to the Sequence Logo manual for the sequence logo parameters and configuration. Sequence Logo supports multiple color schemes and download formats.
Sequence Logo is a web-based sequence logo generator. Sequence Logo generates sequence logo diagrams for proteins and nucleic acids. Sequence logos represent patterns found within multiple sequence alignments. They consist of stacks of letters, each representing a position in the sequence alignment. Sequence Logo analyzes the sequence data inside the user’s web browser and does not store or transmit the alignment data via servers.
Te Reo Maps is an online interactive Maori mapping service. All labels in Te Reo Maps are in Maori, making it the first interactive Maori map. Te Reo Maps is the world map, with all countries and territories translated into Maori. Please refer to the list of countries in Maori for the Maori translations of country names. The list includes all UN members and sovereign territories.
Phonetically is a web-based text-to-IPA transformer. Phonetically uses machine learning to predict the pronunciation of English words and transcribes them using IPA.
Punycode.org is a tool for converting Unicode-based internationalized domain names to ASCII-based Punycode encodings. Use punycode.org to quickly convert Unicode to Punycode and vice versa. Internationalized domains names are a new web standard that allows using non-ASCII characters in web domain names.
My Sequences is an online platform for storing and analyzing personal sequence data. My Sequences allows you to upload your genome sequences and discover insights and patterns in your own DNA.
Словообразовательный словарь «Морфема» дает представление о морфемной структуре слов русского языка и слов современной лексики. Для словообразовательного анализа представлены наиболее употребительные слова современного русского языка, их производные и словоформы. Словарь предназначен школьникам, студентам и преподавателям. Статья разбора слова «сладкоежка» по составу показывает, что это слово имеет два корня, соединительную гласную, суффикс и окончание. На странице также приведены слова, содержащие те же морфемы. Словарь «Морфема» включает в себя не только те слова, состав которых анализируется в процессе изучения предмета, но и множество других слов современного русского языка. Словарь адресован всем, кто хочет лучше понять структуру русского языка.
Разбор слова «кормушка» по составу.
Разбор слова «светить» по составу.
Разбор слова «сбоку» по составу.
Разбор слова «шиповник» по составу.
Разбор слова «народ» по составу.
Разбор слова «впервые» по составу.
Разбор слова «свежесть» по составу.
Разбор слова «издалека» по составу.
Разбор слова «лесной» по составу.
Морфологический разбор глагола «позволяла» онлайн. План разбора.
Для слова «позволяла» найден 1 вариант морфологического разбора
- Часть речи. Общее значение
Часть речи слова «позволяла» — глагол - Морфологические признаки.
- позволять (инфинитив)
- Постоянные признаки:
- 1-е спряжение
- переходный
- несовершенный вид
- изъявительное наклонение
- единственное число
- прошедшее время
- женский род.
Его исключительно культурная речь позволяла смело утверждать, что у него был оксфордский акцент.
Выполняет роль сказуемого.
Поделитесь страницей с друзьями — это лучшая благодарность
Морфологический разбор другого слова
План разбора глагола
- Часть речи. Общее значение
- Морфологические признаки.
- Начальная форма (инфинитив)
- Постоянные признаки:
- Вид (совершенный (что сделать?) или несовершенный (что делать?)
- переходный (употребляется с сущeствительным в винительном падеже без предлога)/ непереходный (не употребляется с существительным в винительном падеже без предлога).
- Спряжение
- Наклонение в зависимости от вопроса:
- Изъявительное — что делал? что делает? что сделает?
- Повелительное — что делай?
- условное — что делал бы? что сделал бы?
- Число
- Время (если есть)
- Лицо (если есть)
- Род (если есть)
- Синтаксическая роль (подчеркнуть как член предложения, является главным или второстепенным членом предложения)
Поделитесь страницей с друзьями — это лучшая благодарность
Оцени материал
7 голосов, оценка 4. 286 из 5
План разбора составлен на основе общих правил, в зависимости от класса и предпочтений учителя ответ может отличаться. Если ваш план разбора отличается от представленного, просто сопоставьте его с данными нашего ответа.
Если морфологический разбор глагола «позволяла» имеет несколько вариантов, то выберите наиболее подходящий вариант разбора исходя из контекста предложения.
Разборы производились исходя из заложенного программного алгоритма, результаты в редких случаях могут быть недостоверны, если вы нашли несоответствие пожалуйста сообщите нам. Представленный результат используется вами на свой страх и риск.
Морфологический разбор слова удаваться онлайн
Слово ‘удаваться’
Слово удаваться является Глаголом (это самостоятельная часть речи, которая отвечает на вопросы «что делать?», «что сделать?»). У глагола удаваться есть постоянные признаки:
- Возвратный/Невозвратный — слово ‘удаваться’ является невозвратный;
- Переходный/Непереходный — слово ‘удаваться’ это непереходный глагол. ; Глагол ‘удаваться’ относится к несовершенному виду.
- Первое лицо: Я — удаюсь/ Мы — удаёмся;
- Второе лицо: Ты — удаёшься/ Вы — удаётесь;
- Третье лицо: Он/Она/Оно — удаётся/ Они — удаются
- Пример изъявительного наклонения: Сколько он ни ловил собаку, она ему не давалась.;
- Пример cослагательного наклонения: Такая кобылка в руки никому не давалась бы.;
- Пример повелительного наклонения: Не давайся схватить тебя, сразу же поединок проиграешь.;
- Род слова определить не возможно потому, что глагол является Инфинитивом.
- Лицо — не определяется в инфинитиве;
- У данного слово время не определяется потому, что слово удаваться является Инфинитивом;
Слово «удаваться» значит:
- Позволять с собой что-нибудь делать, позволять поймать себя, поддаваться.
- Становиться понятным.
«УДАВАТЬСЯ» — это Глагол. Обозначающая действие предмета и отвечает на вопросы «Что делать?» или «Что сделать?». В предложении обычно выполняет роль сказуемого.
давАться
Ударение падает на слог с буквой А. На четвертую букву в слове.
Слово «удаваться» — род не определяется в инфинитиве
Глагол ‘удаваться’ является несовершенным видом.
Переходность глагола «удаваться» — непереходный
Лицо у глагола «удаваться» — не определяется в инфинитиве
«УДАВАТЬСЯ» — это невозвратный глагол
Пример использования наклонений
Изъявительное
Сколько он ни ловил собаку, она ему не давалась.
Сослагательное (условное)
Такая кобылка в руки никому не давалась бы.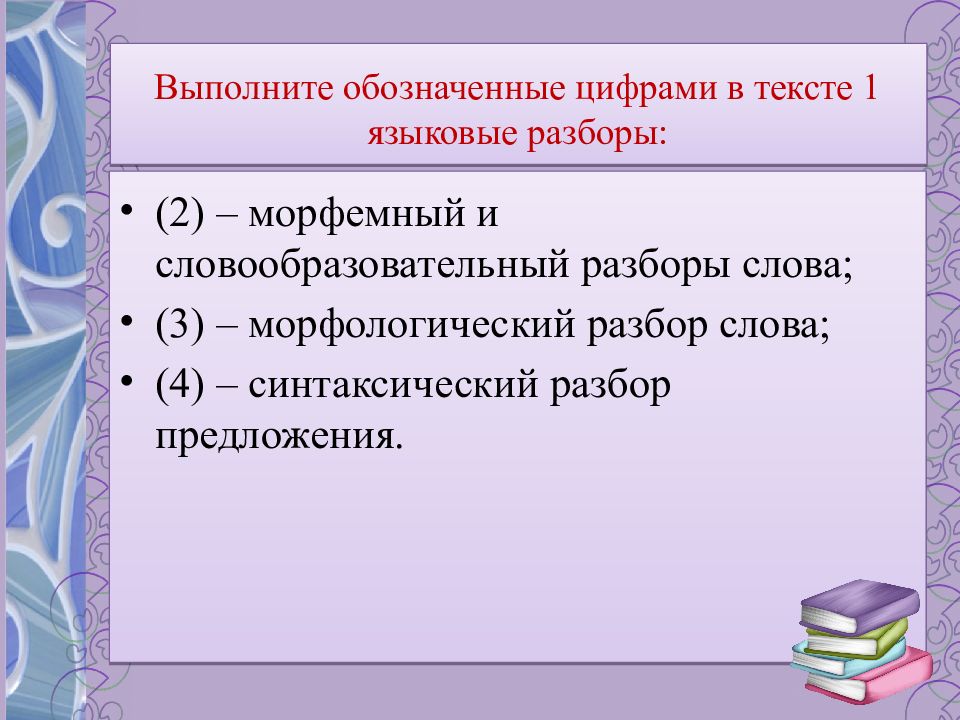
Повелительное
Не давайся схватить тебя, сразу же поединок проиграешь.
Время глагола «удаваться» — не определяется в инфинитиве
Слово «удаваться» — относится к Первому спряжению
будем даваться
будешь даваться
будете даваться
будет даваться
будут даваться
Глагол в прошедшем времени
Она (ед. число)
Оно (ед. число)
Они (мн. число)
- слазить
- добавить
- ворошить
- откупорить
- скряжничать
- квакать
- поворачивать
- вытаптывать
- стираться
- копировать
Наука в Сибири | Соединения с редкоземельными элементам могут светиться и подавлять рост и развитие раковых клеток
В Институте неорганической химии им. А. В. Николаева СО РАН работают над созданием действующих веществ для химиотерапии, которые превосходят уже существующие аналоги по цитотоксичности для раковых клеток, не приводят к резистентности и выступают как агенты для биовизуализации.
Люминесценция полученных соединений
Существует множество способов борьбы с онкологическими заболеваниями, но основным для некоторых видов опухолей по-прежнему остается химиотерапия. В качестве действующих веществ сейчас применяются соединения на основе платины. Они имеют ряд очень серьезных побочных эффектов и часто приводят к резистентности опухоли к лечению. Химиотерапия осуществляется в несколько курсов. Часто первые процедуры проходят успешно, но потом в какой-то момент лекарство перестает действовать на опухолевую линию из-за возникновения у клеток привыкания. Исследователи решили испытать для этих целей соединения меди. Известно, что они препятствуют ангиогенезу (процесс образования новых кровеносных сосудов в новообразовании) и метастазированию.
«Наша научная группа занимается получением смешанно-лигандных полимеров с эндогенными металлами (медь, цинк, марганец, кобальт) и редкоземельными элементами на основе различных органических лигандов. Мы выполняем работы по основной части этой тематики в рамках проекта РНФ и разрабатываем соединения новых противоопухолевых препаратов, которые потенциально способны подавлять рост и развитие раковых клеток», — рассказывает старший научный сотрудник ИНХ СО РАН кандидат химических наук Елизавета Викторовна Лидер.
Ученым также необходимы люминесцирующие соединения для визуализации веществ в клетках. Для этих целей сегодня используются агенты, которые тоже имеют недостатки: они разлагаются на свету и токсичны. Из-за этого их нельзя применять на длительное время.
В ИНХ СО РАН сконструировали ряд комплексов на основе меди, которые превосходят по своим свойствам широко используемый цисплатин. В качестве референсных соединений используются действующие фармакологические препараты на основе платины («Цисплатин» и «Карбоплатин»), которые уже продаются в аптеках. «Работы по цитотоксичности мы ведем совместно с Федеральным исследовательским центром фундаментальной и трансляционной медицины, они предоставили нам доступ к необходимому оборудованию для исследований. Кроме того, мы изучаем взаимодействие с ДНК опухоли, пытаемся понять механизм действия на клеточном уровне», — дополняет Елизавета Лидер.
Исследования поддержаны РНФ, проект 20-73-10207 «Поиск перспективных люминофоров и агентов для противоопухолевой терапии в ряду смешаннолигандных комплексов редкоземельных и эндогенных металлов на основе полипиридиновых, фосфиновых лигандов и производных тетразола».
«Наука в Сибири»
Фото Марии Фёдоровой
Поделись с друзьями:
Грамматика — FieldWorks
Введение
Грамматика, особенно морфология, является важной частью текущей версии Language Explorer. Вместо того, чтобы углубляться во все различные «особенности», связанные с грамматикой, мы предлагаем вам ознакомиться со следующим документом, в котором описывается, как Language Explorer подходит к морфофонологии и морфотактике: Концептуальное введение в грамматику FLEx.
Пример эскиза
Еще один способ узнать, что на данном этапе включено в грамматику, — это взглянуть на один из автоматически сгенерированных набросков грамматики, созданных с помощью Language Explorer.Вот один из выдуманных нами тестовых языков — Калаба.
Разбор
Следующий текст взят из презентации Энди Блэка CTC 04 о возможностях синтаксического анализа в FieldWorks Language Explorer:
Морфологический разбор полезен для:
- Подстрочный текст
- Разбейте слова на их морфемы и дайте каждой морфеме блеск, категорию и т.
Д.
- Разбейте слова на их морфемы и дайте каждой морфеме блеск, категорию и т.
- Проверка орфографии
- Проверка грамматики слова
- Можно ли проанализировать это слово с учетом текущего состояния лексики и грамматики слов?
- Механизм обнаружения языка
- Обнаружение обобщений
- Разработать словарь морфем
- Разработать формализованную морфологию / грамматику слов
- Адаптация с одного родственного языка к другому.
Энди Блэк и Гэри Саймонс написали в 2006 году следующую статью: Подход SIL FieldWorks Language Explorer к морфологическому синтаксическому анализу.
Отличительные особенности текущего инструмента
- Морфологический набросок
В любой момент можно получить запись с описанием текущего состояния грамматики. - Понятия, общие для грамматики I:
Лингвистические понятия корня, основы, перегиба, происхождения, сложных правил и клитики моделируются напрямую. - Скрытность к богатству
Пользователю не обязательно знать, что представляет собой данная форма — он может пометить ее как неизвестную, пока не узнает. - перегиб:
- В качестве конструкции используются флективные шаблоны.
- Имеет инструменты для построения флективных шаблонов.
- Имеет специальный вспомогательный инструмент для сглаживания флективных аффиксов.
- Позволяет использовать классы перегиба.
- Морфологический анализ:
- Используйте и поддерживайте инвентарь словоформ.
- Различать синтаксический анализ, одобренный пользователем, и синтаксический анализ, предсказанный грамматикой.
- При подстрочном анализе текста синтаксический анализ, предсказанный грамматикой, отображается по умолчанию.Пользователь может выбирать или отменять их.
- Возможность показать след синтаксического анализа.
- Наборы слов:
- Возможность создания наборов словоформ, имеющих общую тему; Например:
- Заданная парадигма, включая правильно и неправильно сформированные словоформы;
- слов с общим фонологическим отростком; или
- Слова с составами.
- Возможность проверить точность предсказаний текущей грамматики для каждого такого набора слов.
- Возможность создания наборов словоформ, имеющих общую тему; Например:
- Остаток:
- Разрешить использование «немаркированных» аффиксов.
- Разрешить пользователю писать специальные запреты для морфем.
- Разрешить пользователю писать специальные запреты для алломорфов.
- Разрешить пользователю группировать специальные запреты для фиксации некоторых общих характеристик.
- Возможность / возможность интеграции с остальными инструментами FieldWorks.
Для получения дополнительной информации см. Грамматические категории и Грамматический набросок.
Дата: 21 апреля 2006 г. От: Стивен Граймс Тема: Alchemist 2.0 СОЗДАТЕЛИ: Спраг, Колин; Hu, Yu НАЗВАНИЕ: Alchemist ВЕРСИЯ: 2.0 ДОСТУПЕН НА: http://linguistica.uchicago.edu/alchemist.html Стивен Граймс, Университет Индианы ВВЕДЕНИЕ Alchemist — это инструмент для морфологической аннотации, разработанный Колином Спрагом. и Юй Ху из Чикагского университета в рамках проекта Linguistica Project под руководством Джона Голдсмита.Общая цель Linguistica Project — разработать набор эвристик, которые могут производить неконтролируемый морфологический анализ языка. В этих более широких рамках основной функцией Alchemist является помощь в разработке золотого стандарта для оценки результатов морфологического анализа. Алхимик предназначен для использования лингвистом, который уже знаком с морфологией рассматриваемого тестового языка. В этом обзоре меня дополнительно интересует, пригодится ли Alchemist более широко для полевых лингвистов или морфологов, которые напрямую не заинтересованы в изучении морфологии без учителя. ОБЗОР ПРОГРАММЫ При инициализации программы лингвист начинает с выбора ввода, который будет использоваться — это может быть текстовый корпус или словарь (размеченный список слов). В общем, использование корпуса бегущего текста выгодно перед использованием списков слов, поскольку корпус включает данные о распределении и частоте; кроме того, корпус содержит как производные, так и изменяемые морфологические формы, в то время как словарь может не содержать изменяемых форм. Конечно, качество ввода имеет первостепенное значение, поскольку текст, который не является одноязычным или не «очищен» от случайных символов или знаков препинания, не очень полезен. После завершения базовой настройки в Alchemist лингвист начинает трудную задачу по анализу слов и идентификации морфем. Каждая морфема имеет набор предопределенных атрибутов, которые необходимо указать (также возможны атрибуты, определенные пользователем). Единственный атрибут, который необходимо указать, — это то, является ли морфема корнем или аффиксом. Одна из наиболее интересных особенностей программы заключается в том, что каждая морфема имеет атрибут уверенности, соответствующий убеждению лингвиста в том, насколько «хороша» морфема для языка.Это понятие не определено, но, скорее, оно соответствует «интуиции» лингвиста, которая, вероятно, определяется частотными, семантическими, морфофонологическими или морфологическими соображениями продуктивности. Так, например, английский глагольный суффикс -ing получит высокий рейтинг достоверности, тогда как английское множественное число -en (как в словах «дети» или «волы») получит низкий рейтинг, если его вообще следует считать морфемой. . Имея стратифицированную иерархию морфем в языке, это устанавливает шкалу достоверности градиента, которую неконтролируемый морфологический синтаксический анализатор будет пытаться воспроизвести. К сожалению, каждое слово нужно анализировать индивидуально, поскольку нет возможности анализировать невидимые слова на основе анализа предыдущих слов. Для большого корпуса этот процесс аннотации может показаться очень трудоемким и может включать многократное определение одной и той же морфемы. По завершении морфологического анализа создается XML-файл с использованием тегов и, как показано ниже для английского слова «пение». В представлении XML ниже «пение» сегментировано на две морфемы, а граница морфемы определяется значениями атрибута «start». Alchemist также может экспортировать морфологически аннотированный корпус в виде текста в табличном формате, чтобы его было легче читать для людей. Таким образом, Alchemist служит инструментом для разработки морфологического корпуса языка, который может служить самоцелью или первым шагом в дальнейших исследованиях. ОСОБЕННОСТИ ПРОГРАММЫ Alchemist использует регулярные выражения (сопоставление с образцом) в различных модулях программы. Разработчики считают, что эта возможность сама по себе может быть причиной для использования Alchemist — у пользователя есть возможность искать в лексиконе любое слово, которое соответствует заданному шаблону. При инициализации Alchemist может «очистить» вводимый текст, используя встроенные или определяемые пользователем регулярные выражения, чтобы удалить нежелательные символы, такие как заголовки электронной почты. Примеры встроенных регулярных выражений включают возможность преобразовывать все буквы в нижний регистр, удалять цифры и удалять определенные начальные и конечные символы пунктуации.Синтаксис регулярных выражений должен быть знаком тем, кто знаком с Perl, но графический интерфейс пользователя (GUI) в Alchemist теоретически делает использование этих регулярных выражений менее пугающим. Лексикон можно просматривать в одном из трех основных окон программы в нескольких различных порядках сортировки. Обычно используется алфавитный или обратный алфавитный порядок (в алфавитном порядке справа налево, а не слева направо). Пользователь может выбрать просмотр только проанализированных или непроанализированных слов.iu] * $ /). Все морфемы имеют один обязательный атрибут: они должны быть обозначены либо как аффикс, либо как корень. (Обратите внимание, что для составных слов становится полезной возможность иметь несколько морфем, помеченных как корни). При желании можно указать следующие атрибуты: Тип (Корень, Аффикс, Суффикс, Префикс, Инфикс, Другое) Часть речи (Прилагательное, Существительное, Существительное собственное, Глагол, Другое) Время (Настоящее, Прошедшее простое, Прошедшее несовершенное, Слагательное наклонение) , Другое) Полярность (утвердительная, отрицательная) Человек (1-й, 2-й, 3-й, Другой) Класс (даны номера классов до 20, Другое) Число (Единственное, Двойное, Множественное, Другое) Пол (Женский, Мужской, Нейтральный, Другой) Ясно, что ни одна морфема не будет иметь все перечисленные выше атрибуты, так как некоторые из вышеперечисленных более полезны для существительных или для глаголов.Определяемые пользователем атрибуты также разрешены для классификации более сложных морфологий. Несколько экземпляров одного слова могут быть определены в случае, если одна орфографическая форма будет иметь несколько морфологических анализов. Пример, приведенный в документации к программе, представляет собой орфографическую форму типа «полировка». Он имеет как прилагательное, так и глагольное употребление: pol + ish (что-то из Польши) vs. polish (глагол). (Хотел бы кто-нибудь различать «может» (чтобы иметь возможность) и «может» (контейнер), будет связанным, но второстепенным вопросом.В этой версии Alchemist семантика существует только в той степени, в которой слова определяются их категориями атрибутов.) Лучшая форма помощи пользователю — это не меню справки в программе, а файл .pdf, который можно загрузить с веб-сайта Alchemist. Справка описывает некоторые функции более подробно, чем другие, а некоторые аспекты программы оставлены в некоторой степени недокументированными, но в целом это необходимо для чтения и, как правило, весьма полезно. Для использования специальной формы справки, доступной в самом Alchemist, сначала нужно щелкнуть значок «знак вопроса», который изменяет состояние программы, так что следующий немедленный щелчок обрабатывается как справочный запрос по любому элементу, по которому щелкнули, хотя эта функция имел ограниченную реализацию. ОЦЕНКА В целом программа довольно удобна для пользователя. Alchemist не перегружен опциями меню, поэтому функции программы легко найти, а для этого потребуется довольно быстрое обучение. Разумное использование цвета для выделения морфем также визуально довольно привлекательно. К сожалению, мне удалось найти способы «вывалить» программу. Некоторые случаи критического сбоя происходили, когда я произвольно щелкал в разных окнах, чтобы увидеть, какие функции были доступны.Похоже, что при работе с функцией морфемы слияния возникали и другие сбои. Как упоминалось выше, отдельные экземпляры гомографических морфем изначально рассматриваются как отдельные морфемы. Это значение по умолчанию является преимуществом, когда в языке много идентичных морфем, например -s в английском языке (который используется в качестве метки множественного, притяжательного и единственного числа от третьего лица). Однако это является обузой, когда нужно явно объединить все экземпляры морфемы, чья фонетическая форма однозначна, например, English -tion.Функция слияния изменяет структуру внутренней памяти морфологического лексикона, и я подозреваю, что сбои программы могут быть вызваны повреждением внутренней памяти. Кроме того, мне было непонятно, как «разъединить» две морфемы после того, как они были объединены вместе. Одно из решений — удалить слово из базы данных, а затем создать новую запись для его замены, но я подумал, что должен быть более простой способ сделать это (и, возможно, он есть, хотя это не всегда очевидно). Ряд лингвистических ограничений Alchemist проистекает из ограниченного определения того, чем может быть морфема. Здесь морфология рассматривается как морфология без основных форм, а парадигмы существуют только в той степени, в которой слова имеют общие подстроки. Из-за природы представлений морфема должна состоять из положительного целого числа сегментов / фонем. Учитывая гипотетическую базовую форму / mi / + / ig /, реализованную как [mig], сегмент [i] разделяется двумя морфемами.В Alchemist возможности абстракции ограничены — ни / mi / + / g /, ни / m / + / ig / не были бы идеальными сегментами, но здесь человек вынужден принимать решение. (Возможно, этот сценарий является кандидатом на использование уровня достоверности Alchemist для оценки этих конкретных морфем ниже, но это не идеально, поскольку в целом нет четкой взаимосвязи между морфологической продуктивностью и фонологической непрозрачностью.) Аналогичным образом, морфологический процесс, такой как умлаут, циркумфиксация или тот, который напрямую относится к несегментному или супрасегментарному, как, например, в морфологии морали, будет проблематичным в Alchemist.В конечном итоге лингвист несет ответственность за определение того, что следует принять в качестве привативного в языке (алфавите), и некоторые творческие изменения в базовом представлении могут облегчить проблемы. Например, в тональном языке, где морфология чувствительна к тому, есть ли высокий или низкий тон на гласной, можно удвоить количество гласных, чтобы создать высокий тон и экземпляр низкого тона каждой гласной, чтобы представить эту морфологию. в Алхимике. В целом, однако, без учета множественных зависимостей между отдельными поверхностными сегментами и несегментациями, кажется сложной задачей установить разумный «морфологический золотой стандарт» для большинства языков, за исключением, возможно, китайского. В целом, однако, я считаю Alchemist отличной разработкой, поскольку, по-видимому, существует значительный недостаток общедоступных морфологически аннотированных корпусов. Однако возможное использование таких корпусов не ограничивается только морфологическим обучением. Точно так же, как вычислительные ресурсы позволили фонотактике стать вероятностной, предоставление инструментов для создания морфологически аннотированных корпусов более широко доступными позволит дальнейшее развитие вероятностной морфотактики.Точно так же двуязычные параллельные корпуса с морфологической аннотацией могут привести к развитию машинного перевода. Еще неизвестно, будут ли в конечном итоге использоваться возможности регулярного выражения Alchemist менее технически подкованными лингвистами, но этот аспект программы потенциально может быть использован фонологами для поиска важных примеров слов заданной фонологической формы. Я призываю и призываю всех, кто интересуется, рассмотреть особенности программы, чтобы найти потенциальные приложения в различных областях лингвистики. Программа доступна для загрузки по адресу: http://linguistica.uchicago.edu/alchemist.html О РЕЦЕНЗЕНТЕ Стивен Граймс — доктор философии. студент факультета лингвистики Университета Индианы и интересуется фонологией, морфологией, компьютерной лингвистикой и венгерской лингвистикой. В настоящее время он занимает должность в Исследовательском институте исследований американских индейцев при Университете Индианы, работая над поддержкой разработки автоматизированного текстового процессора, утилиты, помогающей полевым лингвистам в разработке словарей и морфологическом анализе текстов.Он также работает над изучением неконтролируемых методов идентификации морфем в полисинтетических и агглютинативных языках. |
CS440 Лекции
CS440 Лекции CS 440 / ECE 448
Осень 2020
Маргарет Флек
Естественный язык 3
Боути Макбоутфейс (от BBC)
Поиск морфем
Итак, теперь давайте предположим, что у нас есть чистая последовательность слов. Под словом я подразумеваю кусок размера, удобного для более поздних алгоритмов (например,грамм. парсинг, перевод). Это может быть выход распознавателя речи. Или же, поскольку многие системы естественного языка не начинаются с речи, поток слов может быть (очищенный) письменный текст.
Затем эти слова необходимо разделить на морфемы с помощью алгоритма «морфологии». Слова на некоторых языках могут быть очень длинными (например, турецкий), что затрудняет для дальнейшей обработки, если они (хотя бы частично) не разделены в морфемы. Например:
без ответа -> без ответа
предварительных условий -> предварительные условия
Или рассмотрим следующее известное китайское слово:
Чжун + гуу
В современном китайском это одно слово («Китай»).И наша система искусственного интеллекта вероятно, сможет собрать достаточно информации о Китае с помощью рассматривая его как одно двухсложное слово. Но исторически (и в письменной форме system) состоит из двух морфем («середина» и «страна»). Это то, что называется «прозрачное соединение», то есть говорящий на языке будет в курсе отдельных частей. Это знание внутреннего слова структура позволила бы человеку угадать что менее распространенное слово, оканчивающееся на «гуо», также может быть названием страна.
Иногда связанные слова образуются другими процессами, кроме конкатенации, например изменение внутреннего гласного в английском «foot» vs. «feet». Когда это является обычным явлением в языке, мы можем пожелать использовать более абстрактное представление на основе признаков, например
стопа -> стопа + ОСОБЕННОСТИ
футов -> ступня + МНОЖЕСТВЕННОЕ ЧИСЛО
Иногда соответствующее разделение зависит от приложения. Итак, английское притяжательное окончание «s» традиционно пишется как часть предыдущего слова.Однако часто бывает удобно выделить его как отдельное слово при дальнейшей обработке.
POS-теги
Чтобы сгруппировать слова в более крупные единицы (например, предложные фразы), Первым шагом обычно является присвоение тега «части речи» (POS) каждому слову. Вот пример текста из корпуса Брауна, который содержит очень чистые письменный текст.
Северные либералы — главные сторонники гражданских прав и интеграция. Они также привели нацию в направлении государство всеобщего благосостояния.
Вот версия с тегами. Например, «либералы» — это ННС, которая существительное во множественном числе. «Северный» — прилагательное (JJ). Наборы тегов должны различать основные типы слов (например, существительные и прилагательные) и основные варианты, например единственное и множественное число существительных, настоящее и прошедшее время глаголы. Также есть несколько специальных тегов ключевые функциональные слова, такие как HV для «иметь», и пунктуация (например, точка).
Северные / jj либералы / nns являются / ber сторонниками / at вождя / jjs / nns из / в гражданских / jj прав / nns и / cc of / в интеграции / nn./. Они / ppss есть / hv также / rb led / vbn the / at nation / nn in / in / at / at направлении / nn из / в а / при социальном обеспечении / нн государстве / нн ./.
У большинства слов есть только одна общая часть речи. Итак, мы можем подняться до точность около 91% при использовании простого «базового» алгоритма, который всегда угадывает наиболее распространенную часть речи для каждого слова. Однако у некоторых слов есть более одного возможного тега, например «либеральный» может быть существительным или прилагательным. Чтобы понять это правильно, мы можем использовать алгоритмы вроде скрытых марковских моделей (следующая лекция).На чистом тексте хорошо настроенный POS-теггер может обеспечить точность около 97%. Другими словами, POS-тегеры достаточно надежны и в основном используются в качестве стабильная отправная точка для дальнейшего анализа.
Мелкие системы
Многие полезные системы можно построить, используя только простой локальный анализ. слова потока, например слова, биграммы слов, морфемы, теги POS, возможно, неглубокий синтаксический анализ (см. ниже). Эти алгоритмы обычно делают «марковское предположение», т.е. предполагают, что только несколько последних пунктов имеют значение для следующего решения.Например.
- Решаете, вставлять ли границу слова? Посмотрите на последние 5-7 символов.
- Решаете, какой тег POS поставить на следующее слово? Посмотрите на последние 1-3 слова.
Конкретные методы включают конечные автоматы, скрытые марковские модели (HMM) и повторяющиеся нейронные сети (РНС). Позже в этом курсе мы увидим скрытые марковские модели.
Один очень старый тип мелководья система орфографической коррекции. Полезные апгрейды (которые часто работают) включать обнаружение грамматических ошибок, добавление гласных или диакритических знаков на языке (e.грамм. Арабский), где они часто опускаются. Передовые исследовательские задачи включают автоматическую оценку эссе, ответы на стандартные тесты. Распознавание речи использовалось для оценки английского беглость речи и обучение детей чтению (например, вслух). Успех зависит от очень сильных ожиданий относительно того, что человек скажет.
Перевод часто выполняется поверхностными алгоритмами. Чтобы узнать, как делать перевод, мы можем выровнять пары предложений на разных языках, сопоставляя соответствующие слова.
Английский: 18-летние не могут покупать алкоголь.
французский язык: Les 18 ans ne peuvent pas acheter d’alcool
18 | год | лет | банка | ‘т | купить | спирт | |||
Лес | 18 | и | ne | peuvent | па | ахетер | д ‘ | спирт |
Обратите внимание, что некоторые слова не имеют совпадений на другом языке.Для других пар в языках могут быть радикальные изменения в порядке слов.
Корпус совпадающих пар предложений может использоваться для создания словарей переводов (для фразы, а также слова) и извлекать общие сведения об изменениях в порядке слов.
Разбор
Если у нас есть теги POS для слов, мы можем собрать слова в дерево разбора. Есть много стилей построения деревьев синтаксического анализа. Здесь — это дерево округов из банка деревьев Пенсильвании (от Митча Маркуса).
Дерево синтаксического анализа в стиле Penn treebank (от Митча Маркуса)
Альтернативой является дерево зависимостей , подобное приведенным ниже из Лаборатории Google. Достаточно недавний парсер из них называется «Парси МакПарсфейс» после Великобритании Боути Макбоутфейс, показанный выше.
В этом примере левое дерево показывает правильное вложение для слов «в ее машине», то есть изменение слова «водил». Правое дерево показывает интерпретацию, в которой улица находится в машине.
Лингвисты (вычислительные и другие) вели длительные споры по поводу лучший способ нарисовать эти деревья. Однако закодированная информация всегда довольно похожи и в первую очередь связаны с объединением слов которые образуют связные фразы, например «государство всеобщего благосостояния». Это довольно похоже к разбору языков программирования, кроме программирования языки были разработаны, чтобы упростить синтаксический анализ.
«Мелкий синтаксический анализатор» строит только самые нижние части такого дерева.Так что может группировать слова в существительные, предложные фразы и сложные глаголы (например, «идет»). Извлекать эти фразы намного проще чем построение всего дерева синтаксического анализа, и может быть чрезвычайно полезно для строительство неглубоких систем.
Как и низкоуровневые алгоритмы, парсеры часто принимают решения, используя только небольшое количество предшествующего (и, возможно, опережающего) контекста. Однако «один элемент» контекста может быть целым кусок дерева синтаксического анализа. Например, «юную леди» можно считать единый блок.Алгоритмы синтаксического анализа обычно должны учитывать широкий спектр вариантов, например множество вариантов частично построенного дерева. Поэтому они обычно используют поиск луча, т.е. сохраняйте только фиксированное количество гипотез с наилучшей оценкой. Новые методы также попробуйте разделить древовидную структуру между конкурирующими альтернативами (например, динамическое программирование) чтобы они могли хранить больше гипотез и избегать дублирования работы.
Парсеры делятся на три категории
- Unlexicalized: используйте только теги POS для построения дерева.
- По классам: помимо части речи определить общий тип объект или действие (например, человек против транспортного средства)
- Lexicalized: также включить некоторую информацию о слове идентичность / значение
Ценность лексической информации иллюстрируется предложениями. как это, в котором изменение существительная фраза меняет предложную фразу изменяет:
Она шла по улице ..в ее грузовике. (изменяет идущий)
в новом наряде.(изменяет тему)
в Южном Чикаго. (изменяет улицу)
Лучшие парсеры лексикализованы (точность до 94% от Google). Парсер «Parsey McParseface»). Но неясно, сколько информации о слова и их значения. Например, должна ли «машина» всегда вести себя как «грузовик»? Более подробная информация помогает принимать решения (особенно вложение) но требует больше данных для обучения.
Подробнее о наборах ярлыков
Обратите внимание, что набор тегов для корпуса Brown был несколько специализированным. для английского языка, в котором формы «иметь» и «быть» играют важную синтаксическую роль.Для наборов тегов для других языков потребуются некоторые из тех же тегов (например, для существительные), но и категории для типы служебных слов, которые не используются в английском языке. Например, для набора тегов для китайцев или майя потребуется тег для числовых значений. классификаторы, то есть слова, которые идут с числами (например, «три»). для указания приблизительного типа перечисляемого объекта (например, «table» может потребоваться классификатор для больших плоских объектов). Непонятно, лучше ли иметь специализированные наборы тегов. для определенных языков или один универсальный набор тегов, который включает основные функциональные категории для всех языков.
Наборы тегов различаются по размеру в зависимости от теоретических предубеждений люди, создающие аннотированные данные. Меньшие наборы этикеток передают только основная информация о типе слова. Более крупные наборы включают информацию о том, какую роль играет слово в окружающем контексте. Образец размеры
- Penn treebank 36
- Коричневый корпус 87
- «универсал» 12
Разговорный разговорный язык также включает в себя функции, которых нет в письменной речи.В приведенном ниже примере (из корпуса Switchboard) вы можете увидеть заполненную паузу «э-э», а также обрыванное слово «т-». Также обратите внимание на то, что первое предложение разбито на парантийный комментарий («вы знаете»), и третье предложение обрывается в конце. Такие функции усложняют анализ разговорной речи, чем письменный текст.
Я был бы очень осторожен и проверял их. Нашей, пришлось поместить мою мать в дом престарелых. У нее был довольно массивный инсульт о … о …
Я / PRP ‘d / MD быть / VB очень / RB очень / RB осторожно / JJ и / CC, /, ээ / UH, /, вы / PRP знаете / VBP, /, проверяете / VBG them / PRP out / RP./. Э-э / э-э, /, наш / PRP $, /, имел / VBD t- / TO, /, place / VB my / PRP $ mother / NN in / IN a / DT сестринский уход / NN дом / NN ./. Она / ПРП была / ВБД а / ДТ скорее / РБ массивная / JJ ход / НН о / РБ, /, э / УХ, /, про / РБ — /:
Семантика
Представление смысла менее изучено. В современных системах значение индивидуального основы слов (например, «кошка» или «прогулка») основаны на их наблюдаемом контексте. Посмотрим подробности позже в срок. Но эти значения не связаны или слабо связаны с Физический мир.Способы объединения значений отдельных слов в единое значение (например, «рыжий кот») так же хрупки. Очень немногие системы пытаются понять сложные конструкции, включающие кванторы («Сколько стрел не попало в цель?») или относительные предложения. (Примером относительного предложения является «что даст …» в приведенный выше пример синтаксического анализа банка дерева Penn.)
Особая проблема даже для простых приложений заключается в том, что отрицание легко для людей, но сложно для людей. компьютеры.Например, запрос Google по запросу «Африка, а не франкоязычный» возвращает информацию о франкоязычных частях Африки. И это пример циркулярного перевода показывает, что Google выполняет следующее преобразование, которое отменяет полярность совета.
- Ввод: «Так что не пытаюсь через 3 часа».
- Вывод: «Попробуй через 3 часа попробовать».
Приложения, которые могут хорошо работать с ограниченным пониманием включают
- группирование документов по темам, разделение документов в местах смены темы
- анализ настроений: сценаристу нравится этот фильм или этот ресторан?
Три типа поверхностного семантического анализа оказались полезными и почти в пределах имеющихся возможностей:
- классы слов: какие слова похожи друг на друга (например,грамм. люди против овощей)?
- смысловое значение слова: какое было предполагаемое прочтение слова с несколькими значениями (например, «банк»)?
- маркировка семантических ролей: мы знаем, что существительная фраза X связана на глагол Y. Является ли X субъектом / действующим лицом? объект, который действие было сделано? инструмент, используемый для помощи в действии?
- разрешение сопутствующего эталона (см. Ниже)
Разметка семантических ролей включает в себя определение того, как основные словосочетания предложение относится к глаголу.Например, в «Джон вел машину» «Джон» — это субъект / агент, а «машина» — это управляемый объект. Эти отношения не всегда являются объектом, например кто ест кто в «мост поедания грузовиков»? (Поищи в Гугле.)
В настоящее время наиболее популярным представлением классов слов является «вложение слов». Вложения слов дают каждому слову уникальное расположение в многомерном евклидовом пространстве, настроенном так, чтобы похожие слова близко друг к другу. Подробности увидим позже. Популярный алгоритм — word2vec.
Текущий текст содержит ряд «именованных сущностей», то есть существительных, местоимений и существительные, относящиеся к людям, организациям и местам. Совместное разрешение пытается идентифицировать какие именованные сущности относятся к одному и тому же. Например, в этом тексте из Википедии у нас есть определила три сущности, относящиеся к Мишель Обаме, два как Барак Обама, и три как места, которые ни то, ни другое их. Один из источников сложности — это такие предметы, как последний «Обама», который внешне выглядит так, будто мог бы быть любой из них.
[Мишель ЛаВон Робинсон Обама] (родился 17 января 1964 г.) американский юрист, администратор университета и писатель. кто служил [Первая леди США] из С 2009 по 2017 год. Замужем за [44-й президент США], [Барак Обама], и была первой афроамериканской первой леди. Поднят на южной стороне [Чикаго, Иллинойс], [Обама] является выпускник [Университет Принстона] а также [Гарвардская школа права].
Согласованность диалога
С самого раннего возраста люди обладают сильной способностью управлять взаимодействия в течение длительного периода времени.Например. мы можем продолжать говорить на связную тему (например, политику) на весь вечер. Мы может задать дополнительные вопросы, чтобы построить мысленную модель, в которой все части подходят друг к другу. Мы ожидаем, что все части будут соответствовать друг другу при чтении романа. Даже лучшие современные системы искусственного интеллекта пока не могут сделай это.
Одна из конкретных задач — «согласованность диалога». Например, Предположим, вы задаете Google домой вопрос типа «Сколько калорий в банане?» Вы могли бы продолжить с «Как насчет апельсина?» Но большинство этих систем обрабатывают каждый запрос отдельно.Исключения могут содержать только очень краткую теорию контекста или (например, системы обслуживания клиентов) работают в очень ограниченной области. Более интересным примером поддержания согласованности в ограниченной области был Система ILEX для предоставления информация о музейных экспонатах. ( оригинальная бумажная ссылка)
Новейшая нейросетевая система GPT-3 обладает сильной способностью поддерживать локальную согласованность в сгенерированном тексте и диалогах. Однако это происходит Стоимость. Поскольку у него нет реальной модели мира или собственных убеждений, это может быть сбиты с пути наводящими вопросами.
PAPPI: конструкции двойных объектов и нулевая морфема G: примеры анализа
PAPPI: конструкции двойных объектов и нулевая морфема G: примеры анализа Назад к конструкциям двойных объектов и нулевой морфеме G.Модификации теории
Предполагая, что теория принципов и параметров, представленная в стандарте распространение PAPPI, изменения, необходимые для реализации теории двухобъектных конструкций, описанных в (Песецкий 95), являются задокументировано в разделах ниже.Вот краткое описание затронутых модулей:
Структура фразы : внутренняя структура VP и PP должна быть изменена.
Lexicon : введите пустой предлог G. Также изменяется тета-сетка для глаголов с двумя объектами и предлогов, таких как –.
Theta Theory : назначение тета-ролей
Case Theory : для присваивания присваивания Case запретить P присваивать присваиваемый Case его спецификатору.
Правительство : расширить правительство, чтобы позволить глаголу управлять до spec-P.
Структура фразы
PAPPI поставляется с парсером структуры фраз на основе X-bar теория. Как внутренняя структура VP, так и внутренняя структура PP должны быть изменены на позволяют анализировать конструкции двойных объектов как [ VP V [ PP O 1 [ P1 P O 2 ]]].ВП-структура
По умолчанию предполагается, что внутренняя структура VP — [ VP [ V2 [ V1 V DO] IDO]], где V2 и V1 прогнозы на уровне промежуточных столбцов. Эти предположения указаны в xbar.pl
следующим образом:
голова (п). голова (v). голова (а). голова (п). голова (я). голова (с). голова (нег). бар (n1). бар (v1). бар (a1). бар (p1). бар (i1). бар (c1). бар (нег1). бар (v2). макс (np). макс (вп). макс (ap). макс (стр.). макс (i2). макс (c2). макс (негр). %% для меток категорий X и Y, proj (X, Y) выполняется, если Y равно %% непосредственная проекция X. proj (n, n1). proj (n1, np). proj (v, v1). проект (v1, v2). proj (v2, vp). proj (а, а1). proj (a1, ap). proj (p, p1). proj (p1, pp). proj (c, c1). proj (c1, c2). proj (i, i1). proj (i1, i2). proj (нег, нег1). proj (нег1, негр). %% head (X, Y) удерживается, если Y является заголовком X. %% NB. Рефлексивное отношение. голова (п, п). голова (n1, n). голова (np, n). голова (v, v). голова (v1, v). голова (v2, v). головка (вп, в). голова (а, а). голова (а1, а). голова (ап, а). голова (п, п). голова (p1, p). голова (стр, п). голова (c, c). голова (c1, c). голова (c2, c). голова (я, я).голова (i1, i). голова (i2, i). голова (нег, нег). голова (нег1, нег). голова (нег, нег). |
Для нового двойного объекта не требуется промежуточной проекции V2. теории, поскольку косвенные объекты больше не помещаются непосредственно под ВП. Следовательно, мы можем удалить ссылки на V2, что приведет к упрощенному и более упорядоченная система X-bar.
голова (v). бар (v1). макс (вп). proj (v, v1). proj (v1, vp). голова (v, v). голова (v1, v). голова (вп, v). |
Система правил X-bar также может быть упрощена. Схема правил для V2 уровень бара (выделенный ниже) можно исключить.
rule XP -> [XB | spec (XB)] заказанный specFinal st max (XP), proj (XB, XP). спецификация правила (XB) -> [Y] st overtSpec (XB, Y). спецификация правила (XB) -> [] st nullSpec (XB). правило XB2 -> [XB1 | icompl (XB1)] упорядоченный headInitial (X0) ст бар (XB2), proj (XB1, XB2), бар (XB1), голова (XB2, X0), голова (X0).правило icompl (XB) -> [] st lexicalProperty (XB, subcat (_, _), _). правило icompl (XB) -> [] st lexicalProperty (XB, grid (_, Roles), no2ndRole (Roles), _). правило icompl (XB) -> [Y] st lexicalProperty (XB, grid (_, Roles), csr2nd (Roles, Y), Y). правило XB -> [X | Compl (X)] упорядоченный headInitial (X) st bar (XB), proj (X, XB), голова (X). rulecomp (X) -> [] st lexicalProperty (X, grid (_, Roles), no1stRole (Roles), _). Правило Compl (X) -> [Y] stcomp (X, Y). rulecomp (X) -> [Y] st lexicalProperty (X, grid (_, Roles), csr1st (Roles, Y), Y).правило comp (X) -> [Y] st lexicalProperty (X, subcat (Y $ _, _), Y). |
Это приводит к компактной схеме правил X-bar, показанной ниже. Новый
Внутренняя структура VP, предполагаемая для двойных объектов, теперь [ VP V PP], где PP начинается диадическим предлогом, который может вместить
две тета-роли. Это явно закодировано правилом (выделено
ниже), указав, что дополнение X может быть PP при условии, что X имеет
лексическое свойство наличия тета-сетки, удовлетворяющей
предикат has2ndRole / 1
.
rule XP -> [XB | spec (XB)] заказанный specFinal st max (XP), proj (XB, XP). спецификация правила (XB) -> [Y] st overtSpec (XB, Y). спецификация правила (XB) -> [] st nullSpec (XB). правило XB -> [X | Compl (X)] упорядоченный headInitial (X) st bar (XB), proj (X, XB), голова (X). rulecomp (X) -> [] st lexicalProperty (X, grid (_, Roles), no1stRole (Roles), _). Правило Compl (X) -> [Y] stcomp (X, Y). rulecomp (X) -> [Y] st lexicalProperty (X, grid (_, Roles), csr1st (Roles, Y), Y). rulecomp (X) -> [pp] st lexicalProperty (X, grid (_, Roles), has2ndRole (Roles), _). правило comp (X) -> [Y] st lexicalProperty (X, subcat (Y $ _, _), Y). |
Предикат has2ndRole / 1
определяется следующим образом:
has2ndRole (роли): - secondRole (роли, _), !. % зеленый |
Примечания по реализации :
PP, выбранный таким образом с помощью V, также должен быть явно
помечены как подкатегории для структурных примитивов
вроде complement_of / 2
для работы.Это делается путем увеличения
правило структуры фраз с RHS [V, PP] с
цель scPos / 1
(что добавляет флаг подкатегории к PP
положение при его создании).
rhs [v (V), pp (PP)] заказанный headInitial (v) app_goals [scPos (PP)]. |
Наконец, версия has2ndRole / 1
во время синтаксического анализа
нужный. По соглашению он называется schas2ndRole / 1
и
определяется следующим образом:
schas2ndRole (Элемент): - Сетка has_feature элемента (_, IRoles), has2ndRole (IRoles). |
ПП-конструкция
В новой теории ПП диадичны в случае двойного объекта. конструкции, например [ VP V [ PP O 1 [ P1 P O 2 ]]], где O 1 и O 2 являются объектами. Следовательно, мы должны позволить PP иметь возможность наличия спецификатора:spec (p1, np). spec (p1, []). |
Спецификатор NP для PP должен быть A-позицией.Это указано в
правило изменения структуры фразы, добавляющее вызов
до aPos / 1
для всех правил, соответствующих RHS [NP, P1], подробнее
в частности, правило PP -> [NP, P1].
rhs [np (NP), p1 (P1)] заказанные specInitial app_goals [APOS (NP), specPP (NP, P1)]. |
Для вычислительной эффективности, чтобы контролировать структуру фраз
избыточного поколения, мы также модифицируем грамматику, чтобы позволить [NP, P1]
проецировать на PP, только если P имеет внешнюю тета-роль; это закодировано
звонок на specPP / 2
выше. specPP / 2
есть
определено ниже:
specPP (_NP, P1): - Сетка has_feature P1 ([_], _). |
Чтобы контролировать чрезмерную генерацию, мы также добавляем требование через фразу система изменения правил структуры, которая [ PP P1] не может быть проецируется, когда P имеет внешнюю тета-роль:
lhs pp & rhs [p1 (P1)] app_goals [noExtRole (P1)]. |